2F-TP: Learning Flexible Spatiotemporal Dependency for Flexible Traffic Prediction
IEEE TRANSACTIONS ON INTELLIGENT TRANSPORTATION SYSTEMS(2023)
摘要
Accurate traffic prediction is a critical yet challenging task in Intelligent Transportation Systems, benefiting a variety of smart services, e.g., route planning and traffic management. Although extensive efforts have been devoted to this problem, it is still not well solved due to the flexible dependency within traffic data along both spatial and temporal dimensions. In this paper, we explore the flexibility from three aspects, namely the time-varying local spatial dependency, the dynamic temporal dependency, and the global spatial dependency. Then we propose a novel Dual Graph Gated Recurrent Neural Network (DG(2)RNN) to effectively model all these dependencies and offer flexible (multi-step) predictions for future traffic flow. Specifically, we design a Dual Graph Convolution Module to capture the local spatial dependency from two perspectives, namely road distance and adaptive correlation. To model the dynamic temporal dependency, we firstly develop a Bidirectional Gated Recurrent Layer to capture the forward and backward sequential contexts of historical traffic flow, then combine the derived hidden states with their various contributions learned by a temporal attention mechanism. Besides, we further design a spatial attention mechanism to learn the latent global spatial dependency among all locations to facilitate the prediction. Extensive experiments on three types of real-world traffic datasets demonstrate that our model outperforms state-of-the-arts. Results also show our model has more stable performance for the flexible prediction with varying prediction horizons.
更多查看译文
关键词
Roads, Predictive models, Adaptation models, Recurrent neural networks, Data models, Spatiotemporal phenomena, Logic gates, Spatiotemproal dependency, traffic prediction, graph convolutional networks, attention mechanism
AI 理解论文
溯源树
样例
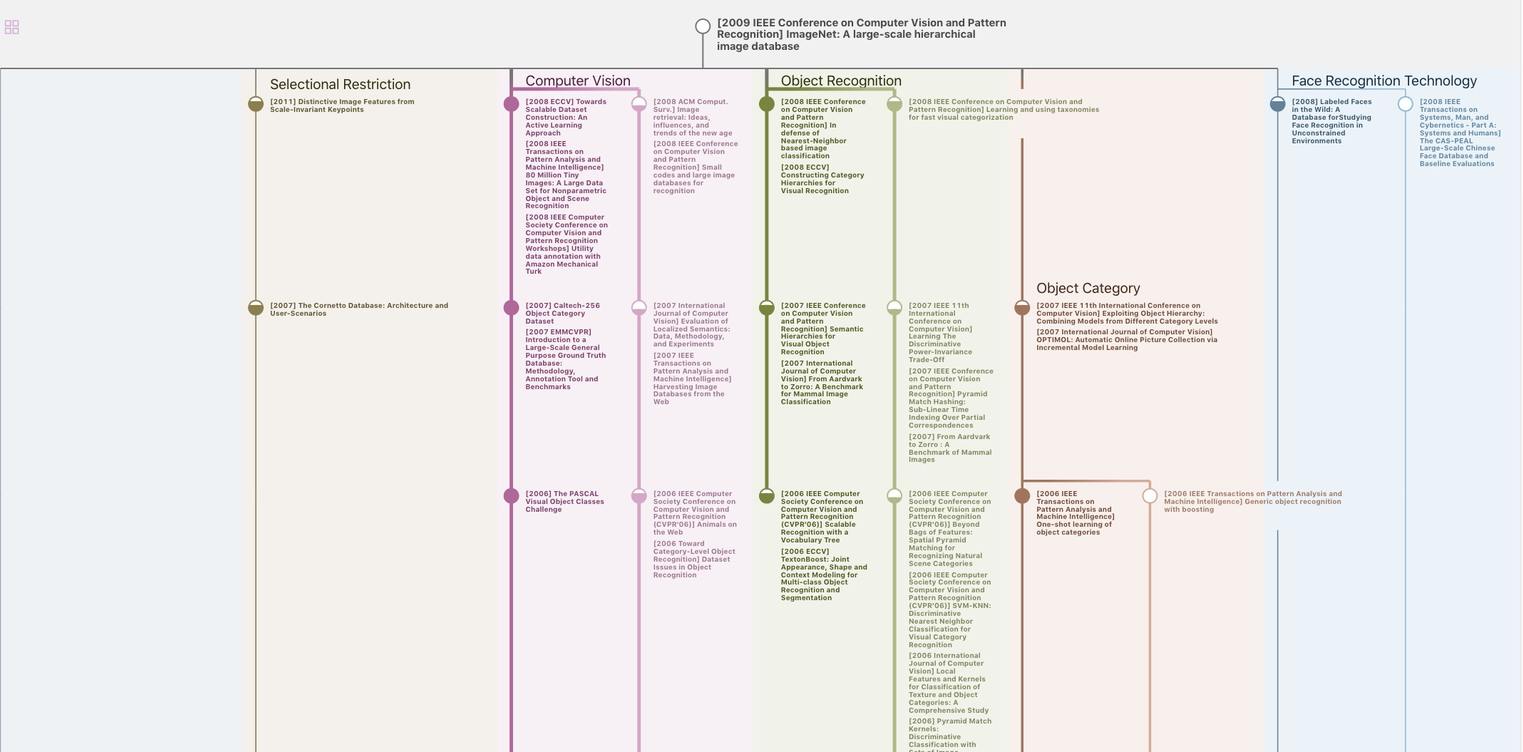
生成溯源树,研究论文发展脉络
Chat Paper
正在生成论文摘要