Efficient Brain Decoding Based on Adaptive EEG Channel Selection and Transformation
IEEE TRANSACTIONS ON EMERGING TOPICS IN COMPUTATIONAL INTELLIGENCE(2022)
摘要
Electroencephalography (EEG) based brain-computer interface (BCI) has a wide range of applications in neuro-rehabilitation and motor assistance. However, brain activities, acquired from a large number of EEG channels, are highly inter-correlated or irrelevant to the brain decoding task, thus reducing the decoding efficiency and accuracy. How to adaptively select the optimal channel number depend on different trials remains a big challenge. To solve this problem, an efficient end-to-end brain decoding model named AdaEEGNet, is proposed in this study. It can reduce the computational cost by adaptively controlling the number of input channels and improve the classification accuracy by reducing over-fitting. Specifically, a lightweight policy module is designed to analyze which channel is needed for decoding current EEG trial. Due to the channel selection process is indifferentiable, we propose to use the Gumbel-Estimator to back-propagate the gradient to train the whole framework. Additionally, a weight coefficient is designed to make a trade-off between brain decoding accuracy and efficiency. To validate the proposed AdaEEGNet feasibility in improving decoding efficiency and accuracy, extensive experiments were conducted on BCI competition IV dataset. The results show that our methods can improve the decoding accuracy by 2% with only 65% computational cost significantly compared with the baseline method.
更多查看译文
关键词
Electroencephalography, Decoding, Brain modeling, Computational modeling, Optimized production technology, Feature extraction, Data models, Channel selection, channel transformation, brain decoding, computational cost, classification accuracy
AI 理解论文
溯源树
样例
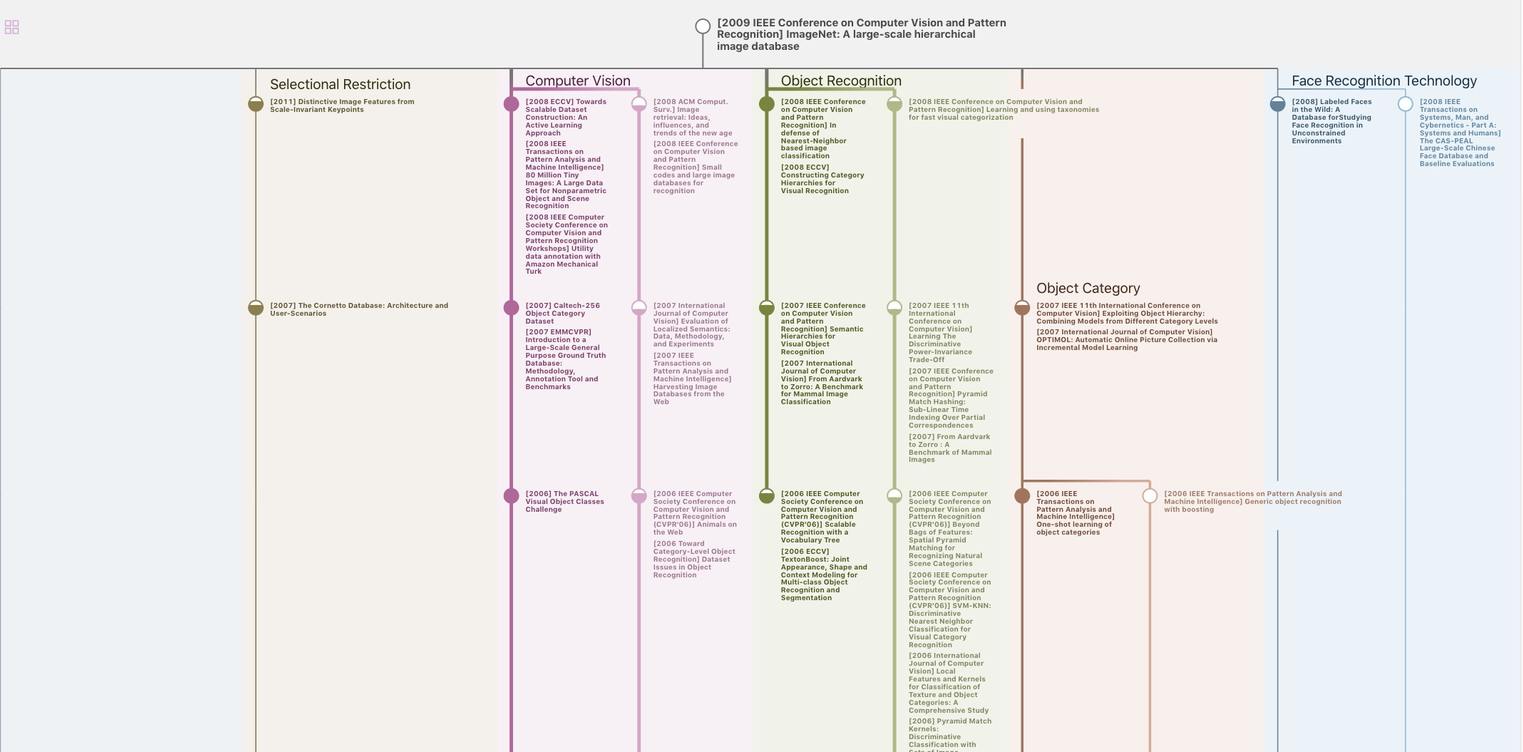
生成溯源树,研究论文发展脉络
Chat Paper
正在生成论文摘要