Speeding up turbulent reactive flow simulation via a deep artificial neural network: A methodology study
CHEMICAL ENGINEERING JOURNAL(2022)
摘要
Turbulent reactive flow simulation often requires accounting for turbulence-chemistry interactions and the subgrid phenomena. Their complexity leads to a trade-off between computational efficiency on one hand and computational accuracy on the other. We attempt to bridge this gap by coupling the power of machine learning with the turbulent reactive flow simulation, specifically in the form of a deep artificial neural network. The Lagrangian Monte Carlo method is chosen as a demonstration case as it is one of the most accurate models for turbulent reactive flow simulation, but also one of the most time-consuming. The workflow consists of training data generation, deep neural network construction, and implementation in ANSYS-Fluent, followed by an evaluation of model accuracy and efficiency, which results in an order of magnitude faster simulation without loss of accuracy thanks to our data-driven deep neural network. This approach can be of universal relevance in speeding up time-consuming models in the field of reactive flow simulation.
更多查看译文
关键词
Turbulent Reactive Flow Simulation, Artificial Neural Network, Lagrangian PDF Method, Turbulence-Chemistry Interaction, Sub-Grid Effect
AI 理解论文
溯源树
样例
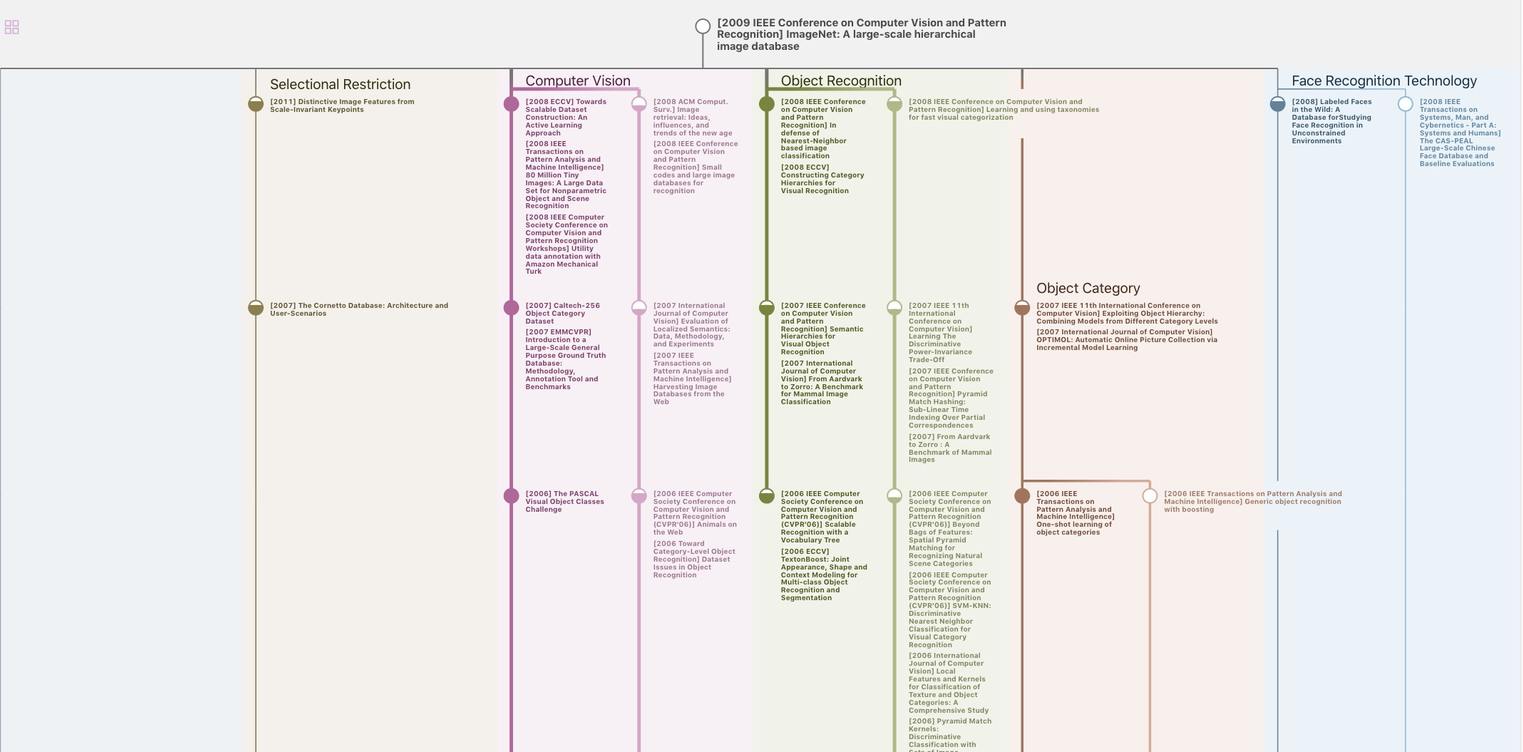
生成溯源树,研究论文发展脉络
Chat Paper
正在生成论文摘要