E-Pilots: A System to Predict Hard Landing During the Approach Phase of Commercial Flights
IEEE ACCESS(2022)
摘要
More than half of all commercial aircraft operation accidents could have been prevented by executing a go-around. Making timely decision to execute a go-around manoeuvre can potentially reduce overall aviation industry accident rate. In this paper, we describe a cockpit-deployable machine learning system to support flight crew go-around decision-making based on the prediction of a hard landing event. This work presents a hybrid approach for hard landing prediction that uses features modelling temporal dependencies of aircraft variables as inputs to a neural network. Based on a large dataset of 58177 commercial flights, the results show that our approach has 85% of average sensitivity with 74% of average specificity at the go-around point. It follows that our approach is a cockpit-deployable recommendation system that outperforms existing approaches.
更多查看译文
关键词
Aircraft, Machine learning, Atmospheric modeling, Accidents, Safety, Meters, Recurrent neural networks, Decision support systems, hard landing prediction, machine learning, neural networks
AI 理解论文
溯源树
样例
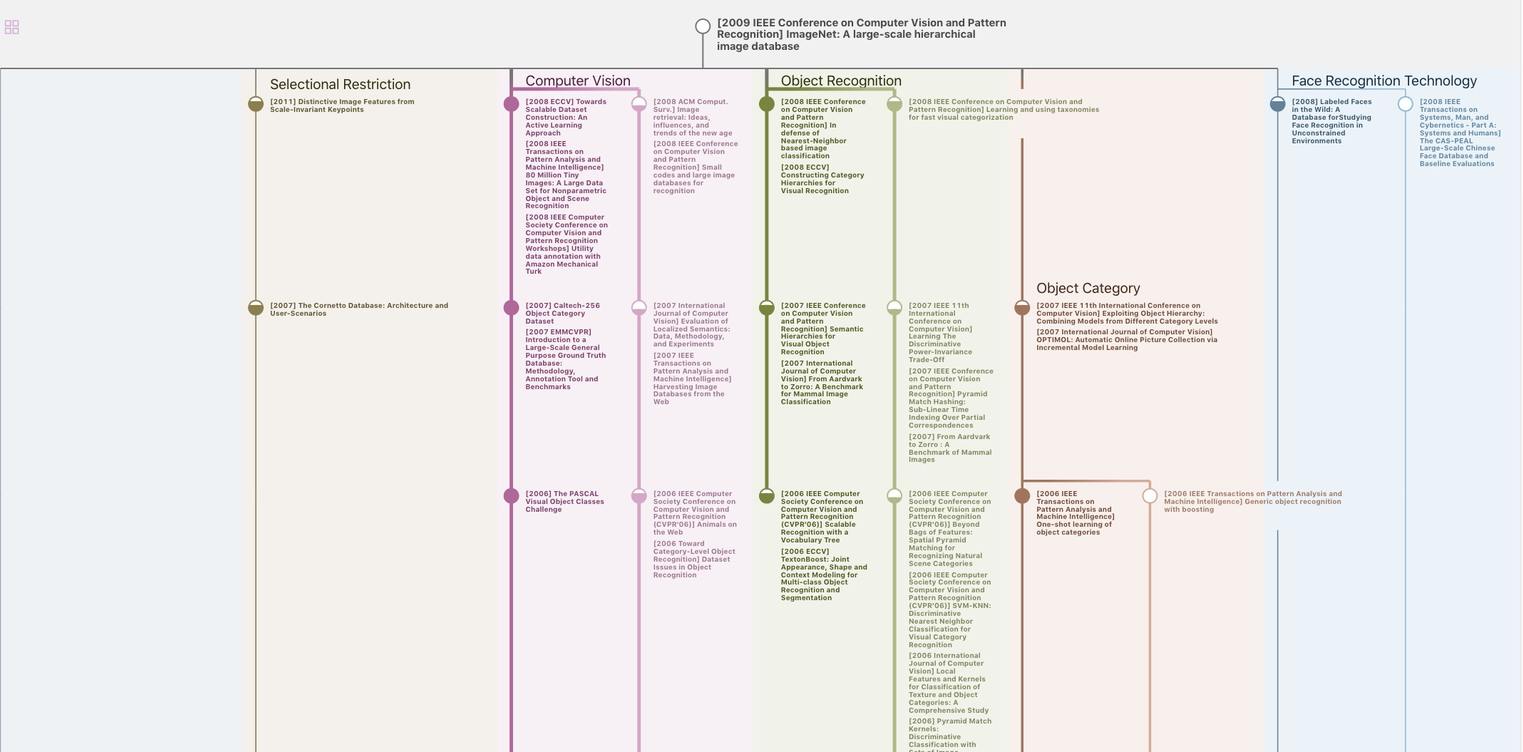
生成溯源树,研究论文发展脉络
Chat Paper
正在生成论文摘要