Tests and classification methods in adaptive designs with applications
JOURNAL OF APPLIED STATISTICS(2023)
摘要
Statistical tests for biomarker identification and classification methods for patient grouping are two important topics in adaptive designs of clinical trials related to genomic studies. In this article, we evaluate four test methods for biomarker identification in the first stage of an adaptive design: a model-based identification method, the popular two-sided t-test, the nonparametric Wilcoxon Rank-Sum test (two-sided), and the Regularized Generalized Linear Models. For patients grouping in the second stage, we examine classification methods such as Random Forest, Elastic-net Regularized Generalized Linear Models, Support Vector Machine (SVM), Gradient Boosting Machine (GBM), and Extreme Gradient Boosting (XGBoost). Simulation studies are carried out to assess the performance of the different methods. The best identification methods are chosen based on the well-known F-1 score, while the best classification techniques are selected based on the area under a receiver operating characteristic curve (AUC). The chosen methods are then applied to the Adaptive Signature Design (ASD) with a real data set from breast cancer patients for the purpose of evaluating the performance of ASD in different situations.
更多查看译文
关键词
Boosting and optimization, logistic regression, classification trees, genes, sensitive and non-sensitive patients, targeted agent
AI 理解论文
溯源树
样例
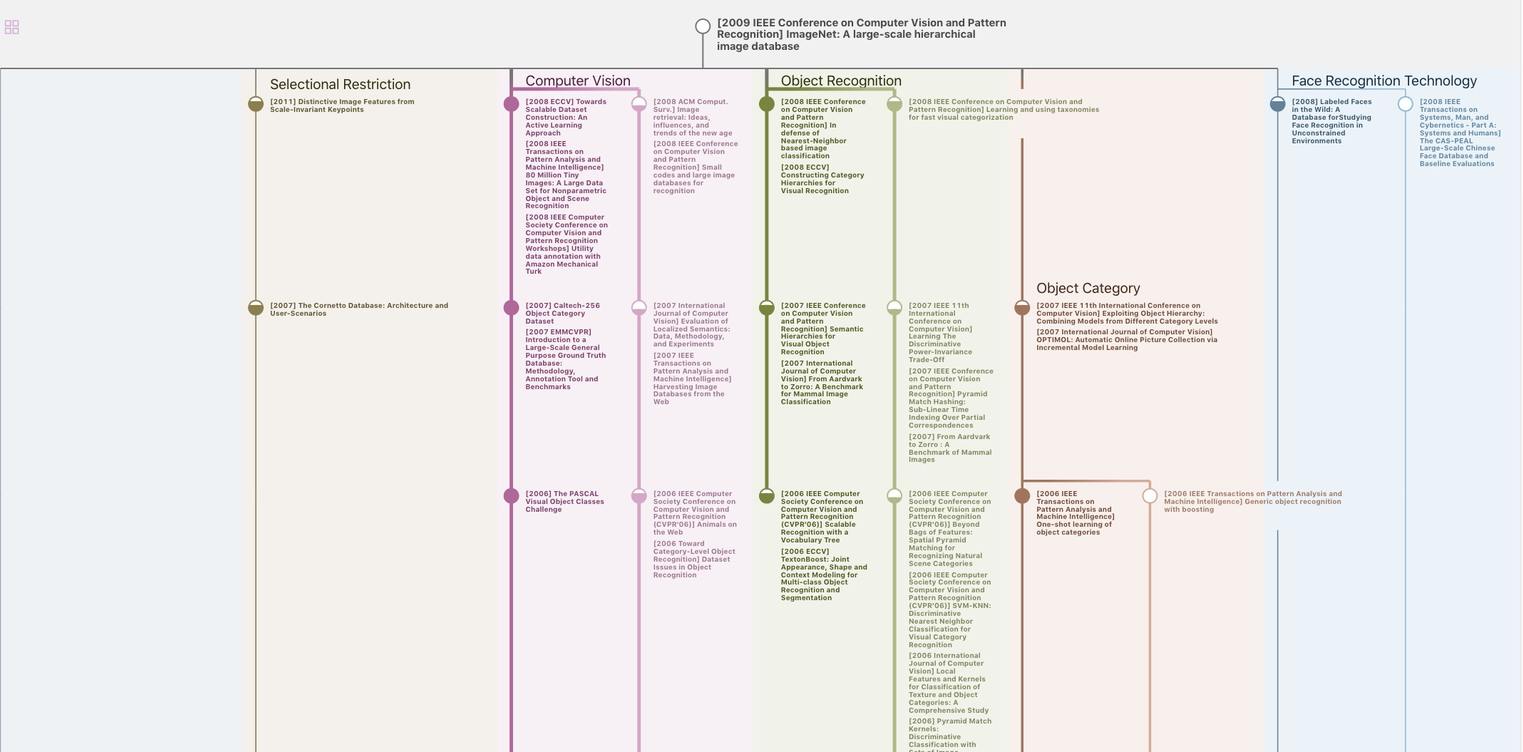
生成溯源树,研究论文发展脉络
Chat Paper
正在生成论文摘要