Estimating location parameters in sample-heterogeneous distributions
INFORMATION AND INFERENCE-A JOURNAL OF THE IMA(2022)
摘要
Estimating the mean of a probability distribution using i.i.d. samples is a classical problem in statistics, wherein finite-sample optimal estimators are sought under various distributional assumptions. In this paper, we consider the problem of mean estimation when independent samples are drawn from d-dimensional non-identical distributions possessing a common mean. When the distributions are radially symmetric and unimodal, we propose a novel estimator, which is a hybrid of the modal interval, shorth and median estimators and whose performance adapts to the level of heterogeneity in the data. We show that our estimator is near optimal when data are i.i.d. and when the fraction of 'low-noise' distributions is as small as Omega (d log n/n) is the number of samples. We also derive minimax lower bounds on the expected error of any estimator that is agnostic to the scales of individual data points. Finally, we extend our theory to linear regression. In both the mean estimation and regression settings, we present computationally feasible versions of our estimators that run in time polynomial in the number of data points.
更多查看译文
关键词
location estimation, robust statistics, sample heterogeneity
AI 理解论文
溯源树
样例
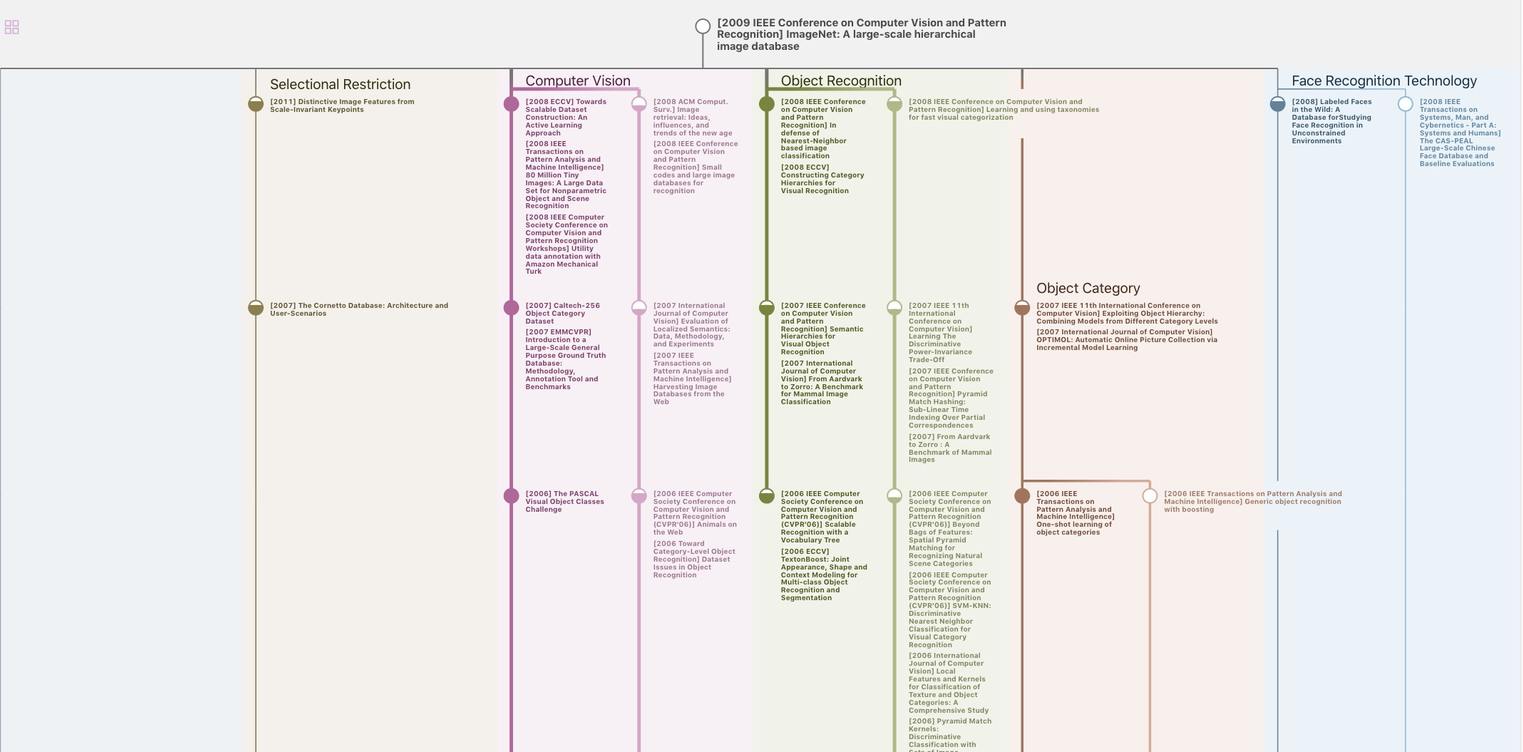
生成溯源树,研究论文发展脉络
Chat Paper
正在生成论文摘要