A Framework of Structural Damage Detection for Civil Structures Using Fast Fourier Transform and Deep Convolutional Neural Networks
APPLIED SCIENCES-BASEL(2021)
摘要
In the field of structural health monitoring (SHM), vibration-based structural damage detection is an important technology to ensure the safety of civil structures. By taking advantage of deep learning, this study introduces a data-driven structural damage detection method that combines deep convolutional neural networks (DCNN) and fast Fourier transform (FFT). In this method, the structural vibration data are fed into FFT method to acquire frequency information reflecting structural conditions. Then, DCNN is utilized to automatically extract damage features from frequency information to identify structural damage conditions. To verify the effectiveness of the proposed method, FFT-DCNN is carried out on a three-story building structure and ASCE benchmark. The experimental result shows that the proposed method achieves high accuracy, compared with classic machine-learning algorithms such as support vector machine (SVM), random forest (RF), K-Nearest Neighbor (KNN), and eXtreme Gradient boosting (xgboost).
更多查看译文
关键词
structural health monitoring, FFT-DCNN, structural damage detection, deep learning, civil structures
AI 理解论文
溯源树
样例
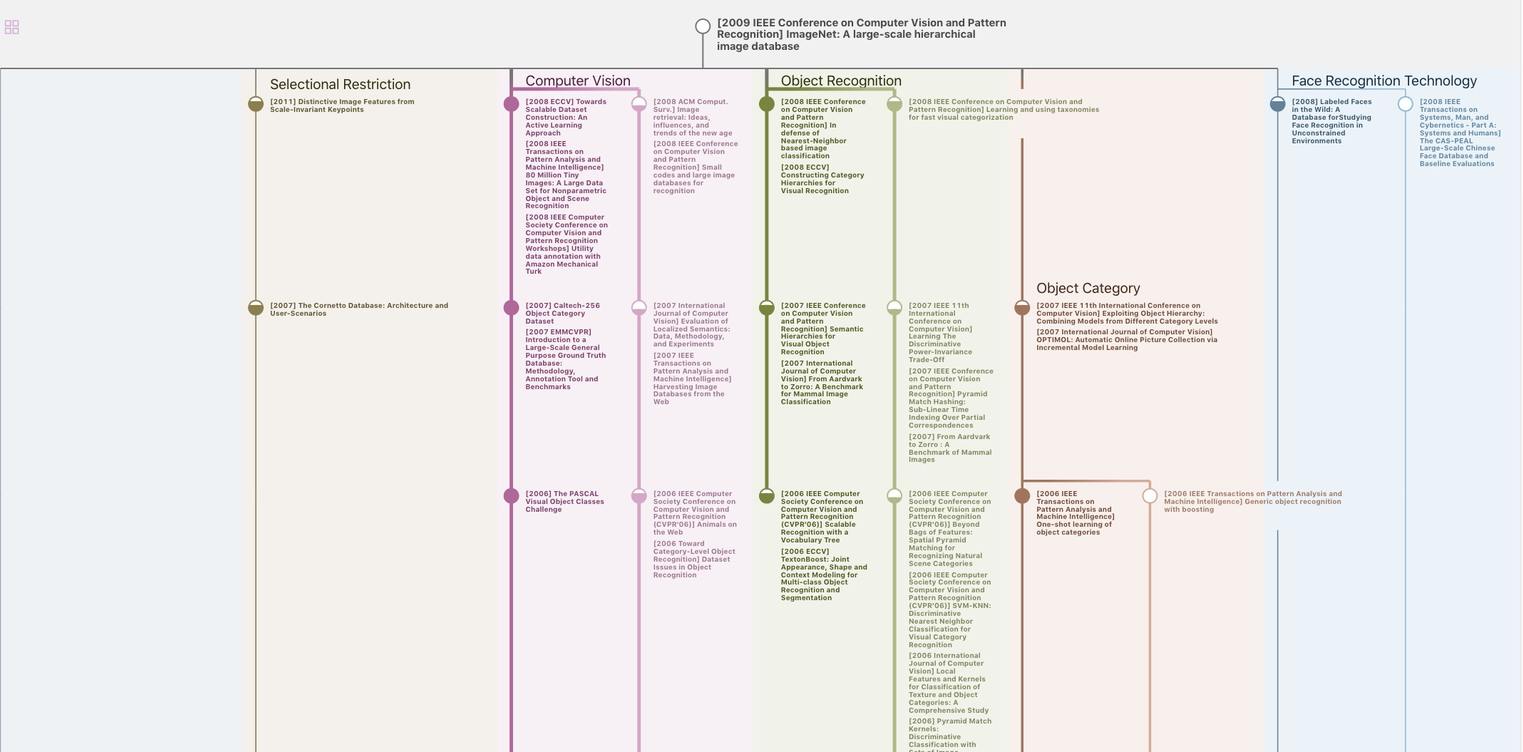
生成溯源树,研究论文发展脉络
Chat Paper
正在生成论文摘要