Semantic Sampling
LOW RESOURCE SOCIAL MEDIA TEXT MINING(2021)
摘要
A variety of tasks involving social media text require mining rare samples. In text classification, information retrieval, and other NLP tasks, working with very skewed or imbalanced data sets poses many challenges. In such settings, training data sets can be rapidly bootstrapped using highly targeted sampling strategies. This chapter draws on work in active learning, semantic similarity, and sampling strategies to address a variety of social media text mining tasks. The topics involved are particularly well suited for social media analysis. Most tasks surrounding user generated social media text such as content moderation, and recommendations often involve rapid model construction in response to real world events in real time. The methods discussed allow task-specific data sets and models to be constructed rapidly often using just a handful of initial samples. We then explore extensions to sample across languages-allowing powerful pipelines that can transfer resources from well-resourced languages to their low-resource counterparts.
更多查看译文
关键词
Active learning, Cross lingual sampling, Semantic sampling, Rare positive mining, Certainty sampling, Uncertainty sampling
AI 理解论文
溯源树
样例
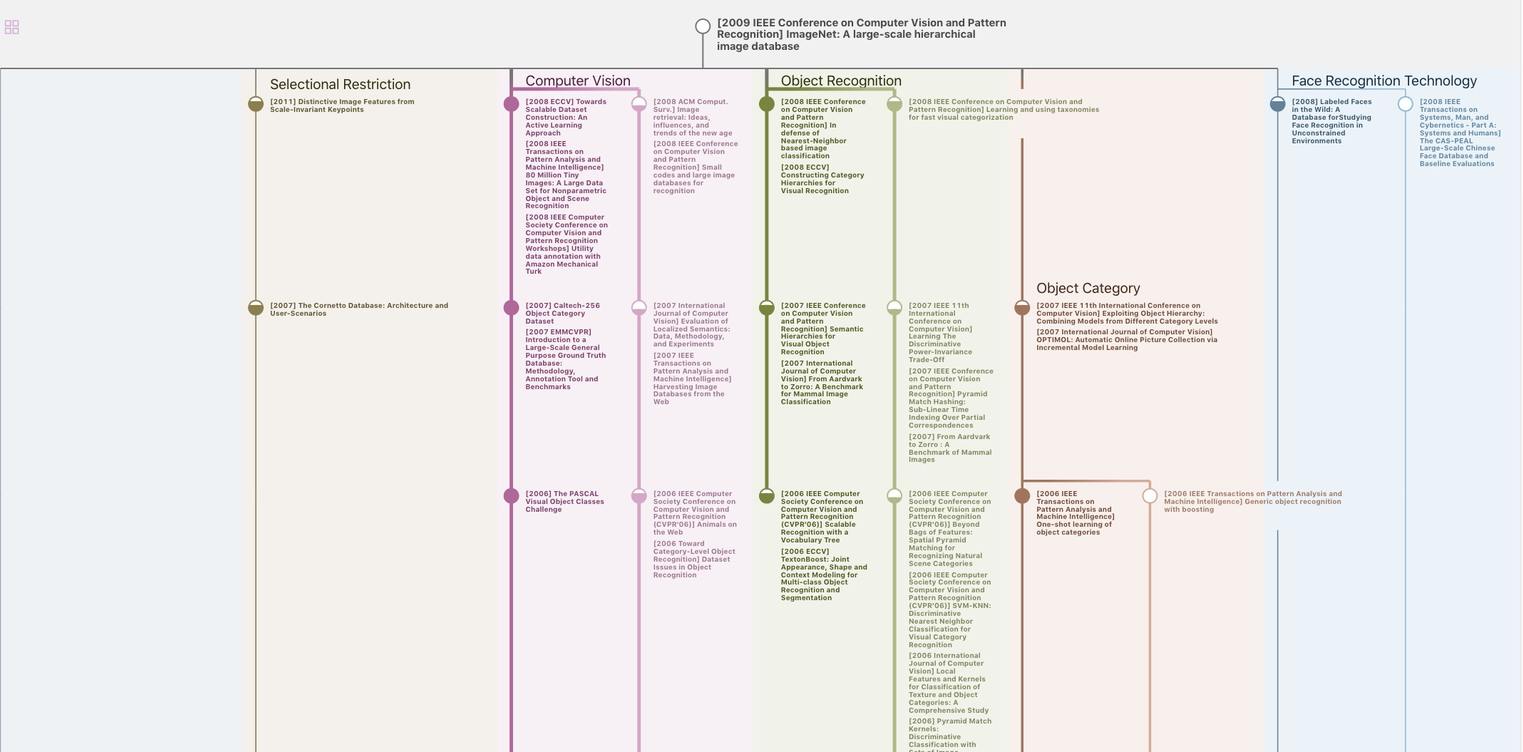
生成溯源树,研究论文发展脉络
Chat Paper
正在生成论文摘要