Modelling personalised car-following behaviour: a memory-based deep reinforcement learning approach
TRANSPORTMETRICA A-TRANSPORT SCIENCE(2024)
摘要
To adapt to human-driving habits, this study develops a personalised car-following model via a memory-based deep reinforcement learning approach. Specifically, Twin Delayed Deep Deterministic Policy Gradients (TD3) is integrated with a long short-term memory (LSTM) (abbreviated as LSTM-TD3). Using the NGSIM dataset, unsupervised learning-based clustering and data feature analyses are performed. The driving characteristics related to safety, efficiency and comfort are extracted for different driving styles, i.e. aggressive, common and conservative. Then, reward functions are constructed for different driving styles by incorporating their driving characteristics. By resorting to the TD3 policy within a recurrent actor-critic framework, LSTM-TD3 optimises the car-following behaviour via trial-and-error interactions according to the reward functions. Results show that compared with LSTM-DDPG and DDPG, LSTM-TD3 reproduces personalised car-following behaviour with desirable convergence speed and reward. It reveals that LSTM-TD3 can reflect the essential difference in safety, efficiency and comfort requirements among different driving styles.
更多查看译文
关键词
Car-following,autonomous driving,twin delayed deep deterministic policy gradients,long short-term memory,driving styles
AI 理解论文
溯源树
样例
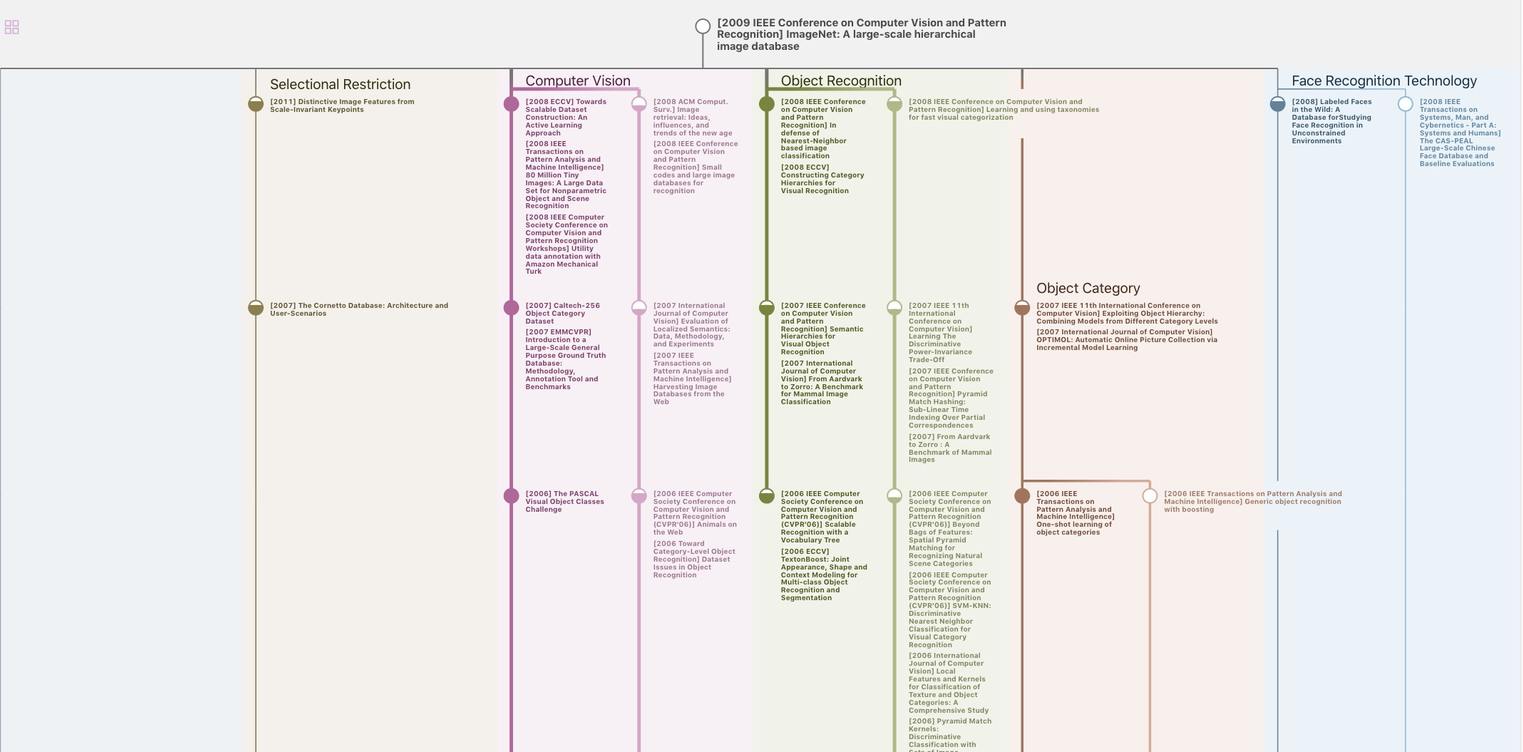
生成溯源树,研究论文发展脉络
Chat Paper
正在生成论文摘要