A data-driven Kriging model based on adversarial learning for reliability assessment
STRUCTURAL AND MULTIDISCIPLINARY OPTIMIZATION(2022)
摘要
The huge computational cost is a main barrier of structural reliability assessment for complex engineering. Surrogate models can release the CPU burden of reliability assessment, however, it is very challenging to guarantee the prediction accuracy of failure probability. In this study, we propose an Adversarial Learning-Based Kriging model (ALBK), where two models learn from and compete with each other to achieve an improved model accuracy. First, the initial models are established, and fitting accuracy is evaluated by each other with the proposed criterion. Then, the modeling parameters are optimized according to the evaluation results. The data-driven criteria and adversarial relationship promote the evolution of modeling parameters. Moreover, a triple-indicator method is provided to choose the final model and avoid oscillation. The ALBK adjusts modeling parameters with alternative evolution, while the predicted values are more accurate than those of Kriging. Finally, an adaptive ALBK method is provided with new samples added to improve the accuracy of reliability assessment. Through several numerical examples, it can be seen that the ALBK always provides the best results with the fewest assessment calls, and the robustness is also good.
更多查看译文
关键词
Surrogate model,Adversarial learning,Kriging,GANs,Reliability assessment
AI 理解论文
溯源树
样例
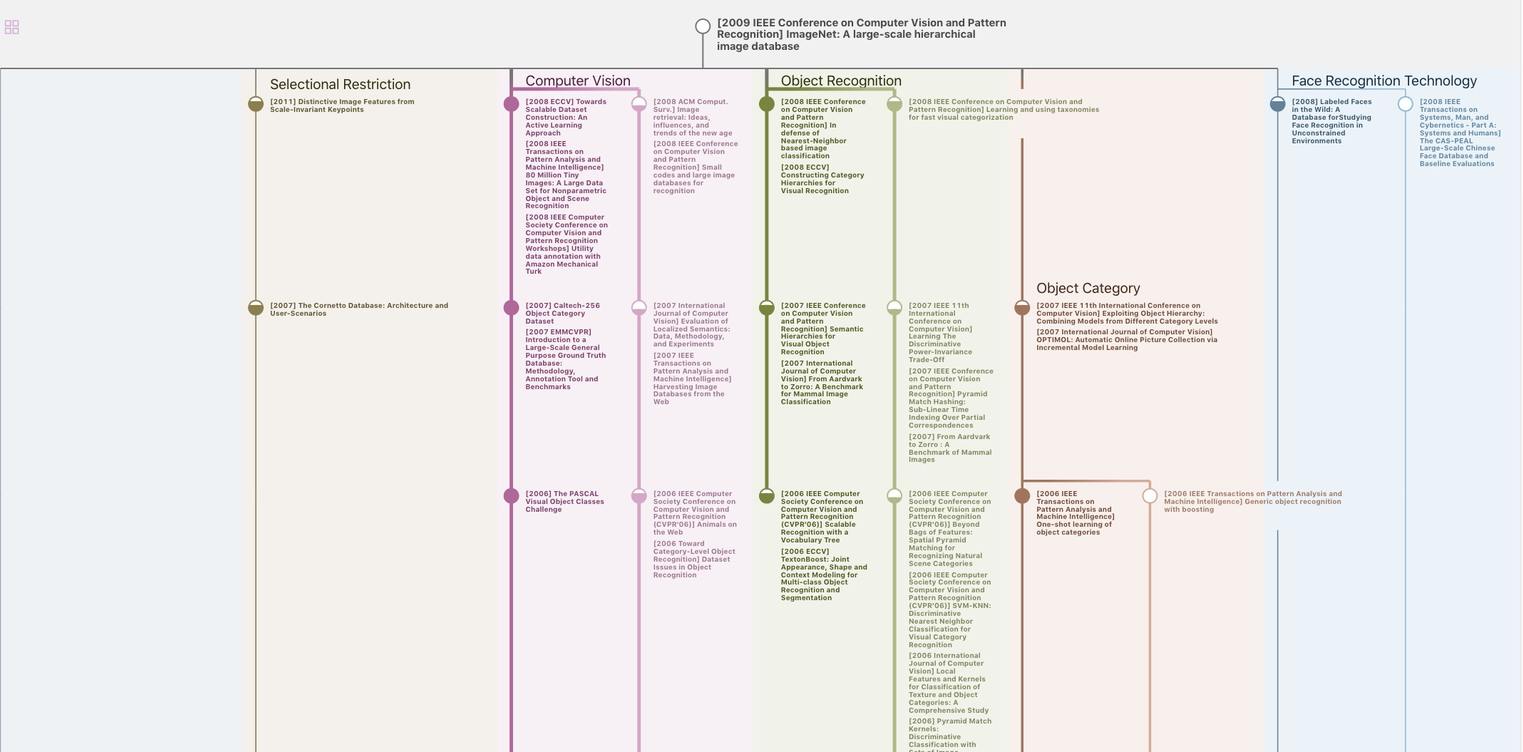
生成溯源树,研究论文发展脉络
Chat Paper
正在生成论文摘要