Computationally efficient integrated design and predictive control of flexible energy systems using multi-fidelity simulation-based Bayesian optimization
OPTIMAL CONTROL APPLICATIONS & METHODS(2021)
摘要
We present a multi-fidelity black-box optimization approach for integrated design and control (IDC) of constrained nonlinear systems in the presence of uncertainty. The IDC framework is becoming increasingly important for the systematic design of next-generation (flexible) manufacturing and energy systems. However, identifying optimal solutions to realistic IDC problems is intractable when (i) the dynamics occur on much shorter timescales than the system lifetime, (ii) the uncertainties are described by continuous random variables with high variance, and (iii) operational decisions involve a mixture of discrete and continuous variables. Instead of aggressively simplifying the problem to improve tractability, we develop a simulation-based optimization procedure using high-quality decision rules that map information that can be measured online to optimal control actions. In particular, we rely on the Bayesian optimization (BO) framework that has been shown to perform very well on noisy and expensive-to-evaluate objective functions. We also discuss how BO can be extended to take advantage of computationally cheaper low-fidelity approximations to the high-fidelity IDC cost function. Three major low-fidelity approximation strategies are described in this work, which are related to the simplification of the system simulator, decision rule solution method, and time grid. Lastly, we demonstrate the advantages of multi-fidelity BO on the design of a solar-powered building heating/cooling system (with battery and grid support) under uncertain weather and demand conditions with hourly variation over a year-long planning horizon.
更多查看译文
关键词
optimization methods, predictive control, uncertain systems
AI 理解论文
溯源树
样例
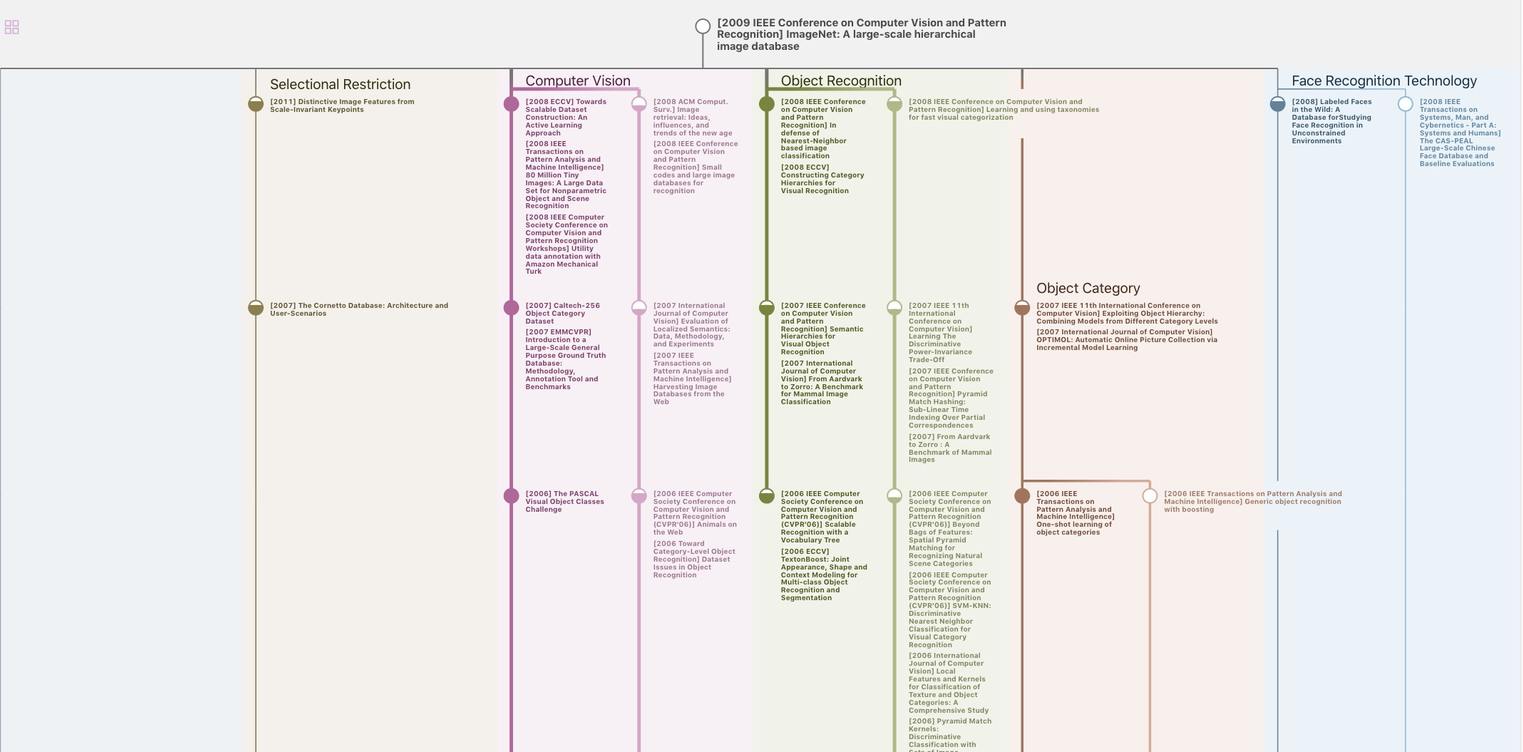
生成溯源树,研究论文发展脉络
Chat Paper
正在生成论文摘要