Pairwise feature-based generative adversarial network for incomplete multi-modal Alzheimer’s disease diagnosis
VISUAL COMPUTER(2022)
摘要
Magnetic resonance imaging (MRI) and positron emission tomography (PET) are widely used in diagnosis of Alzheimer’s disease (AD). In practice, incomplete modality problem is unavoidable due to the cost of data acquisition. Deep learning based models especially generative adversarial networks (GAN) are usually adopted to impute missing images. However, there are still some problems: (1) there are many regions unrelated to the disease and have little significance in the actual diagnosis in brain images, which are very cumbersome to generate. (2) The image generated by GAN would introduce noises causing the poor performance in the diagnostic model. To address these problems, a pairwise feature-based generation adversarial network is proposed. Specifically, features from the original brain images are extracted firstly. For the paired data without modality loss, the extracted MRI features are used as input to generate its corresponding PET features, which not only reduces the scale of the model, but also ensures the direct correlation between the generated features and the diagnosis. In addition, the available real PET features of the paired samples are added as label to constrain the generated ones. Finally, the attention mechanism is adopted in both the generator and discriminator, which can effectively retain the structural information of the feature itself. A large number of experiments have demonstrated that our proposed method has achieved promising results in the diagnosis of AD.
更多查看译文
关键词
Incomplete multi-modal,GAN,AD,Medical imaging
AI 理解论文
溯源树
样例
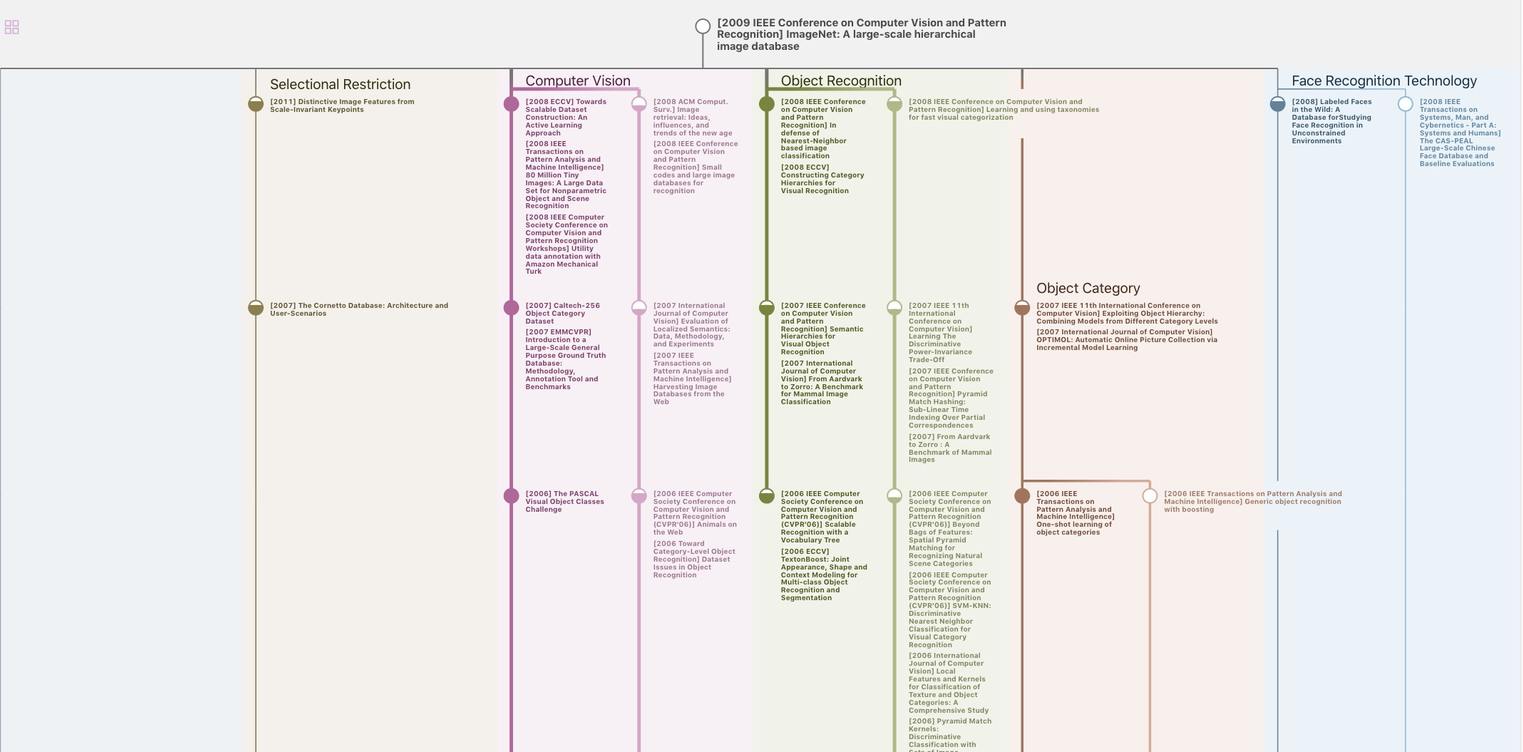
生成溯源树,研究论文发展脉络
Chat Paper
正在生成论文摘要