LightAdam: Towards a Fast and Accurate Adaptive Momentum Online Algorithm
Cognitive Computation(2022)
摘要
Adaptive optimization algorithms enjoy fast convergence and have been widely exploited in pattern recognition and cognitively-inspired machine learning. These algorithms may however be of high computational cost and low generalization ability due to their projection steps. Such limitations make them difficult to be applied in big data analytics, which may typically be seen in cognitively inspired learning, e.g. deep learning tasks. In this paper, we propose a fast and accurate adaptive momentum online algorithm, called LightAdam, to alleviate the drawbacks of projection steps for the adaptive algorithms. The proposed algorithm substantially reduces computational cost for each iteration step by replacing high-order projection operators with one-dimensional linear searches. Moreover, we introduce a novel second-order momentum and engage dynamic learning rate bounds in the proposed algorithm, thereby obtaining a higher generalization ability than other adaptive algorithms. We theoretically analyze that our proposed algorithm has a guaranteed convergence bound, and prove that our proposed algorithm has better generalization capability as compared to Adam. We conduct extensive experiments on three public datasets for image pattern classification, and validate the computational benefit and accuracy performance of the proposed algorithm in comparison with other state-of-the-art adaptive optimization algorithms
更多查看译文
关键词
Adaptive training algorithm,Convex optimization,Online learning,Projection-free
AI 理解论文
溯源树
样例
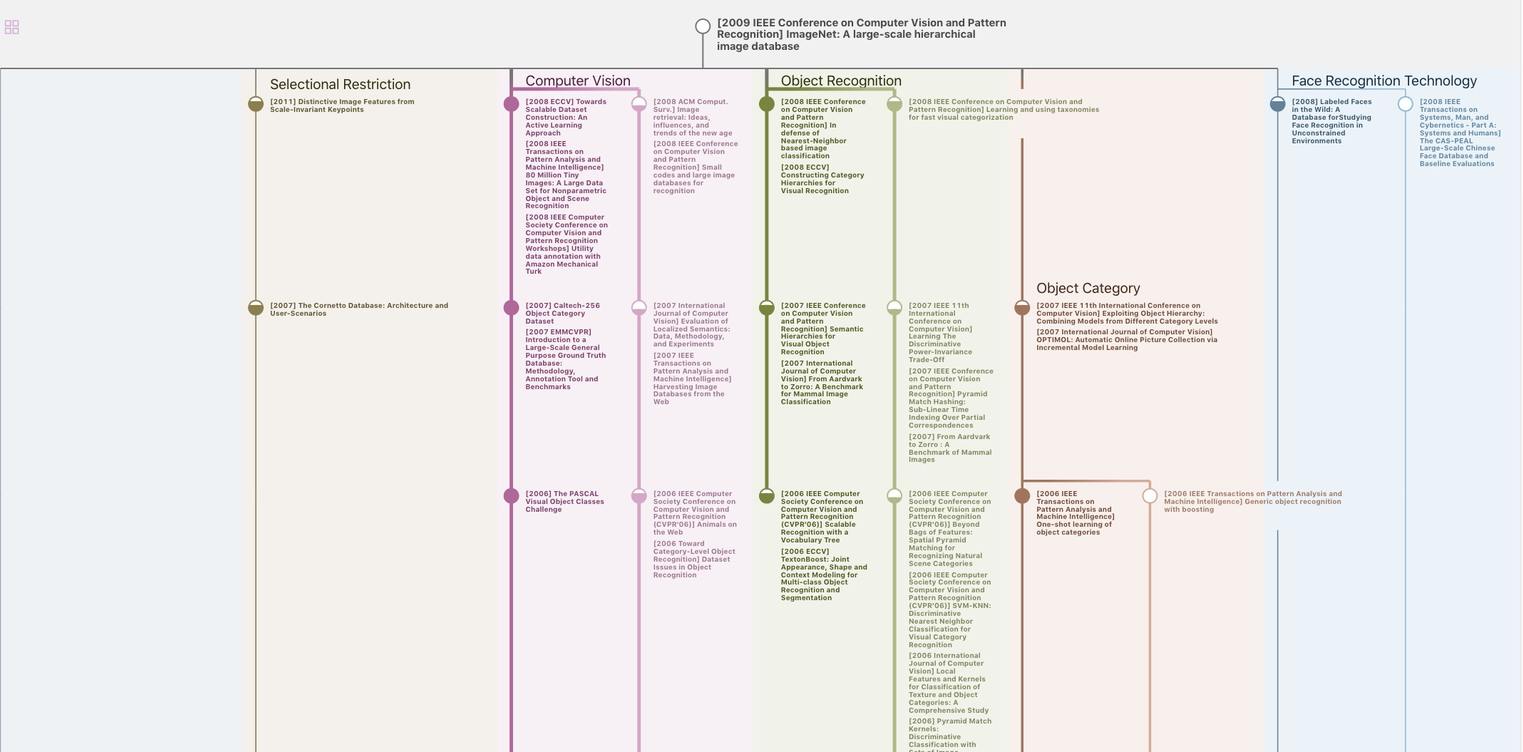
生成溯源树,研究论文发展脉络
Chat Paper
正在生成论文摘要