Accurate and effective framework for identifying track defects
Measurement(2022)
摘要
Timely and effective identification of track surface defects is helpful to improve the safety of railway operation. However, the complex service environment leads to the diversity of defects, and it is difficult to realize automatic identification. Therefore, an improved residual network feature extraction module is proposed, and a framework of track surface defect identification is constructed. To be specific, the overall framework and the core method are described in detail at first. Then, the optimal training parameters are determined through experiments, and the performance of different feature extraction modules is further compared. Finally, the performance of the proposed framework is verified by experiments using multi-class images. The results show that the average identification accuracy is 94.45%, the average frame speed is 0.000052 s. Correspondingly, the feature identification model adopted in this paper offers Accuracy of 97.09%, Loss of 0.0773 and RMSE of 0.06. The proposed model yields strong robustness.
更多查看译文
关键词
Track surface,Surface defect identification,Convolutional neural network,Improved residual network
AI 理解论文
溯源树
样例
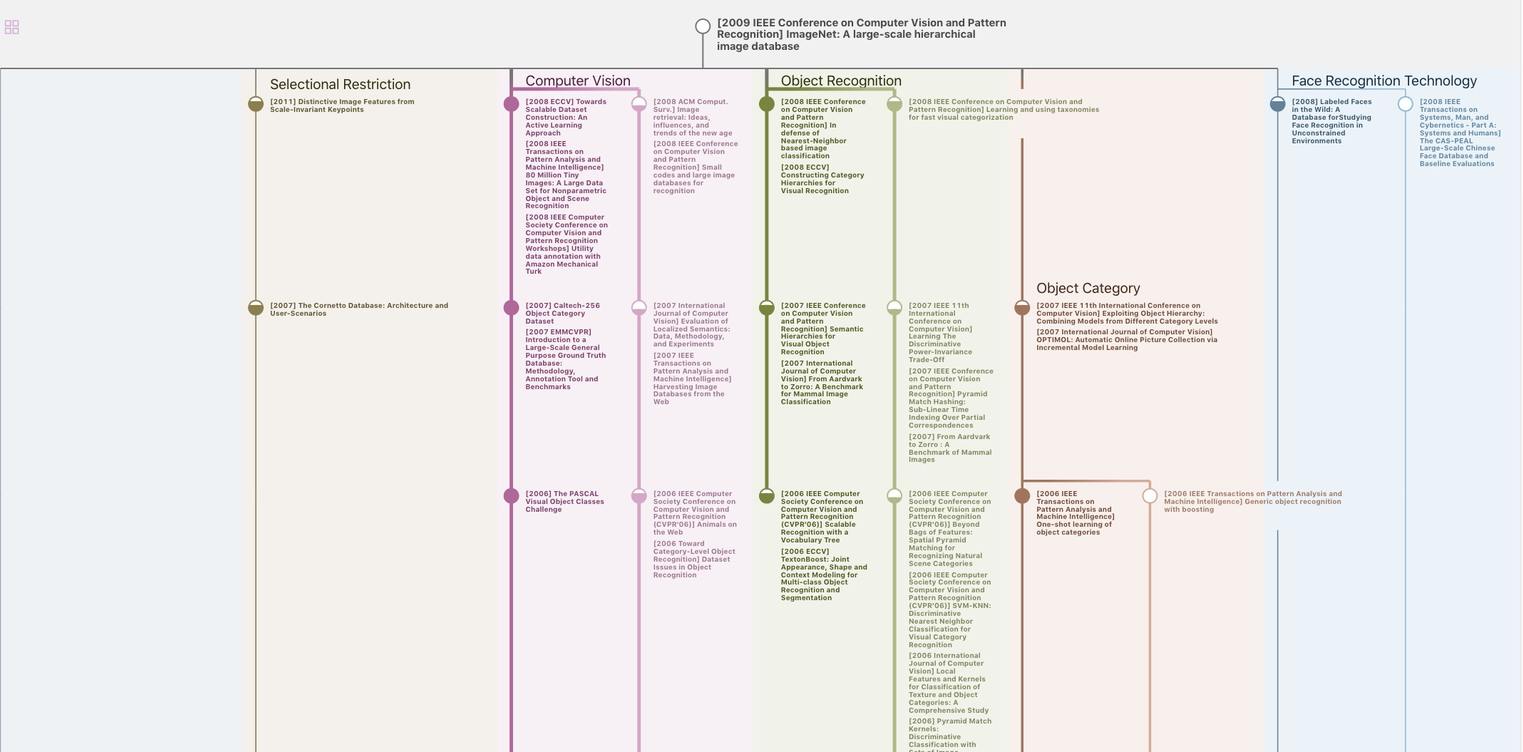
生成溯源树,研究论文发展脉络
Chat Paper
正在生成论文摘要