Learning Associations between Features and Clusters: An Interpretable Deep Clustering Method
2021 INTERNATIONAL JOINT CONFERENCE ON NEURAL NETWORKS (IJCNN)(2021)
摘要
Clustering is a challenging problem when many features are irrelevant to separate clusters. Also, different clusters may relate to various feature subsets. This work proposes a deep clustering algorithm that localizes the search for instance clusters and their relevant features. The relevant features of each cluster are defined as those with high associations (dependency) within that cluster. Given the number of clusters K, we formulate the problem as K-parallel auto-reconstructive learning, where low-rank graph learning, rooted in graph Laplacian theory, is used to explore the unknown feature associations of each cluster. The model performs automatic feature weighting on residuals to minimize loss from the corresponding cluster. Through such design, different feature subsets can be learned to calculate the loss from different clusters. Subsequently, the associations between features and clusters can be acquired, and better clustering result can be achieved. Moreover, the associated features of each cluster can be used to interpret the clustering patterns.
更多查看译文
关键词
Deep Clustering, Interpretability, Laplacian
AI 理解论文
溯源树
样例
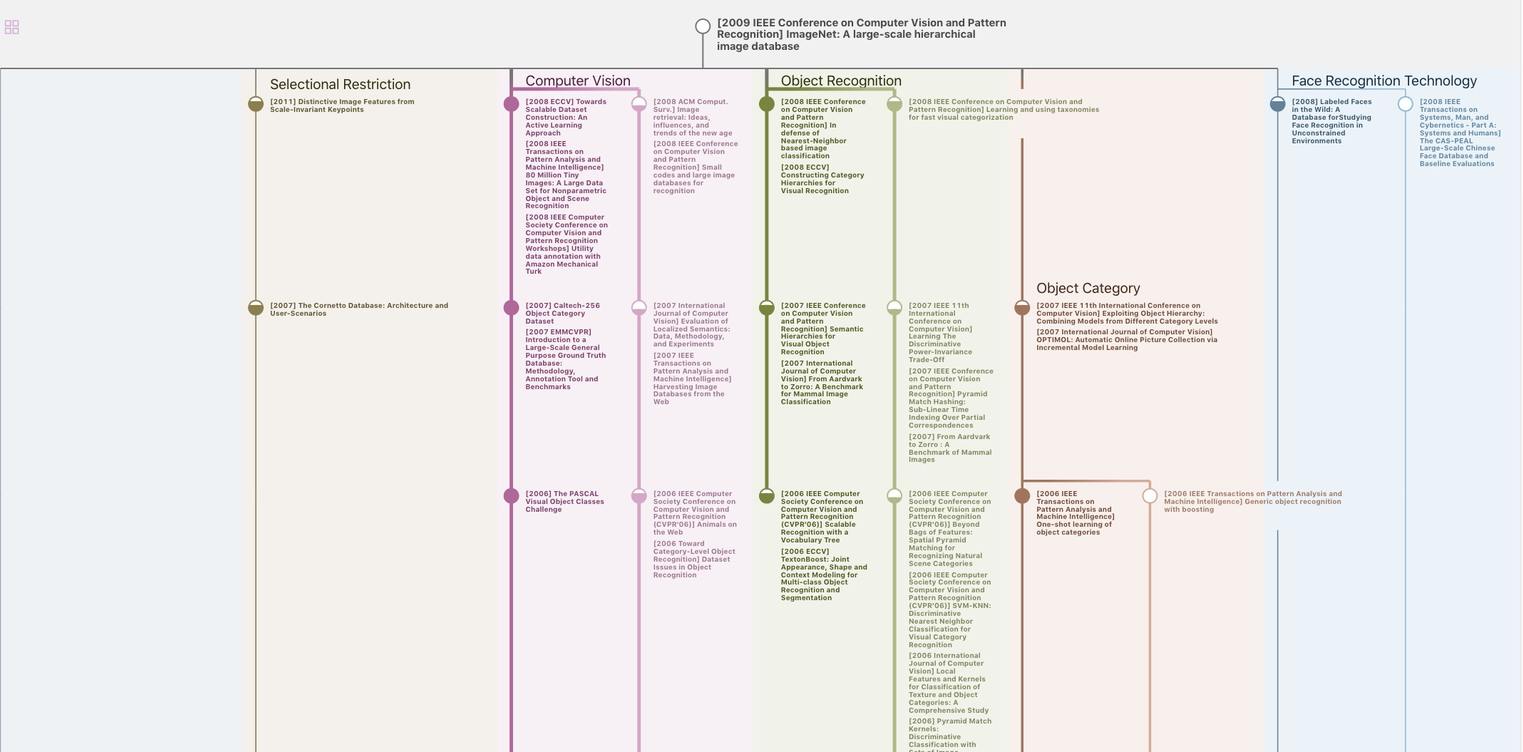
生成溯源树,研究论文发展脉络
Chat Paper
正在生成论文摘要