Machine Learning Techniques Applied to Predict Tropospheric Ozone in a Semi-Arid Climate Region
MATHEMATICS(2021)
摘要
In the last decade, ground-level ozone exposure has led to a significant increase in environmental and health risks. Thus, it is essential to measure and monitor atmospheric ozone concentration levels. Specifically, recent improvements in machine learning (ML) processes, based on statistical modeling, have provided a better approach to solving these risks. In this study, we compare Naive Bayes, K-Nearest Neighbors, Decision Tree, Stochastic Gradient Descent, and Extreme Gradient Boosting (XGBoost) algorithms and their ensemble technique to classify ground-level ozone concentration in the El Paso-Juarez area. As El Paso-Juarez is a non-attainment city, the concentrations of several air pollutants and meteorological parameters were analyzed. We found that the ensemble (soft voting classifier) of algorithms used in this paper provide high classification accuracy (94.55%) for the ozone dataset. Furthermore, variables that are highly responsible for the high ozone concentration such as Nitrogen Oxide (NOx), Wind Speed and Gust, and Solar radiation have been discovered.
更多查看译文
关键词
tropospheric ozone, machine learning, El Paso-Juarez, semi-arid climate
AI 理解论文
溯源树
样例
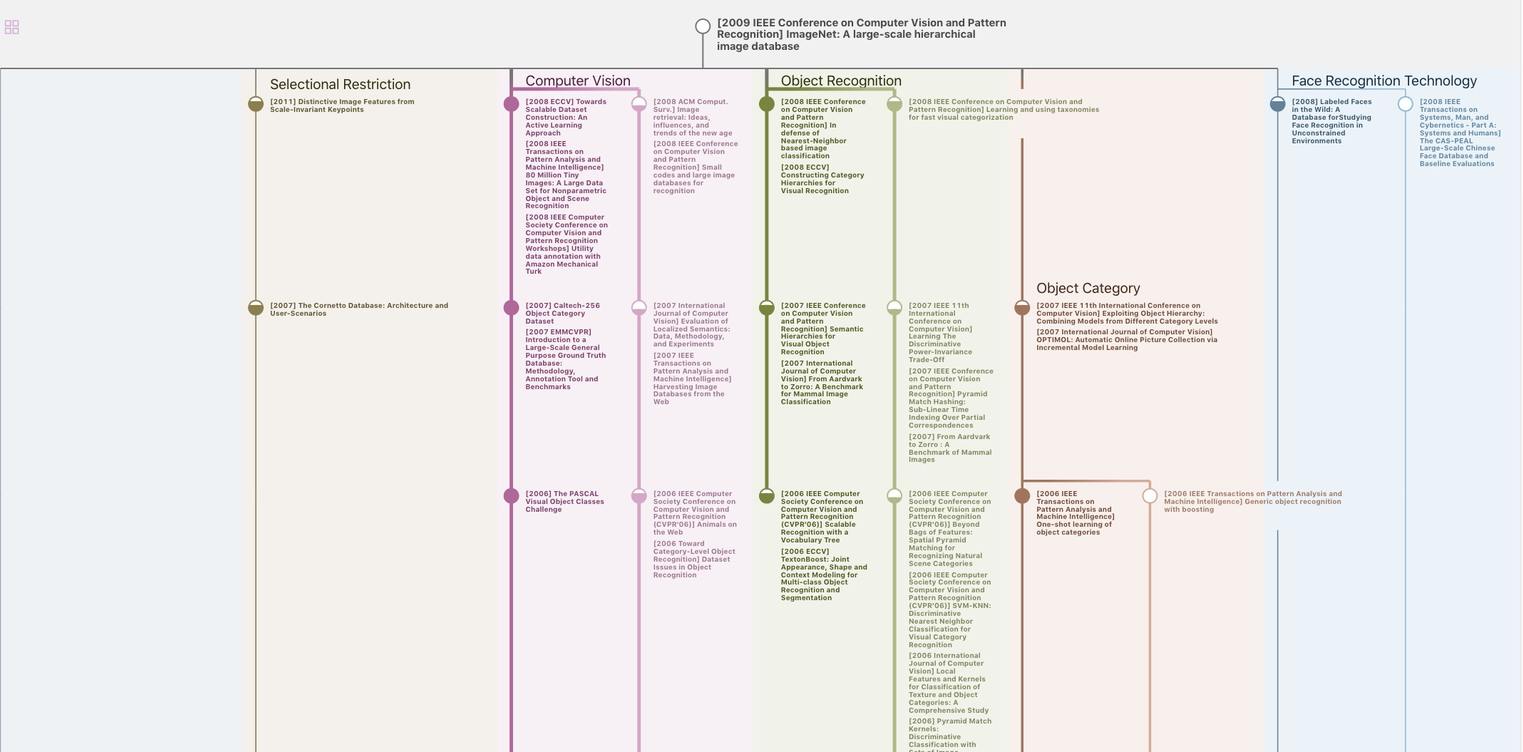
生成溯源树,研究论文发展脉络
Chat Paper
正在生成论文摘要