Cascaded Model (Conventional plus Deep Learning) for Weakly Supervised Segmentation of Left Ventricle in Cardiac Magnetic Resonance Images
IETE TECHNICAL REVIEW
摘要
Accurate segmentation of biomedical images is of utmost importance for the classification in various physiopathological conditions. The conventional method of segmentation of biomedical images is manual labeling by image processing and biomedical experts which is a hectic as well as a tedious task to carry. Also, it takes a longer time to label, and still, inter-operative error possibilities exist. To overcome these problems, an end-to-end segmentation pipeline is required with minimal or no operator intervention. Here, we propose to use a cascade of conventional and deep learning methods to obtain accurate segmentation. First, seed region growing, random walker, and K-means clustering are used individually to roughly delineate the region of interest, and then, the generated masks are used to train the deep learning model to get the final segmentation. The proposed method is validated for the segmentation of the left ventricle in cardiac magnetic resonance images (ACDC Dataset). The method is again cross-validated with another dataset on which the model is not previously trained. Further, the segmentation pipeline is experimented to work with a lesser number of images in three different levels (Degree I, II, and III). Dice score, Jaccard index, and Hausdorff distance are used as metrics to show the effectiveness of the proposed weakly supervised method. The results obtained by different methods are competitive enough to the state-of-the-art(supervised) method. The proposed cascaded weakly supervised method paves the way towards the unsupervised segmentation of biomedical images with minimal or no manual intervention.
更多查看译文
关键词
Autoencoder, Cardiac MRI, Deep learning, K-means clustering, Random walker, Seed region growing
AI 理解论文
溯源树
样例
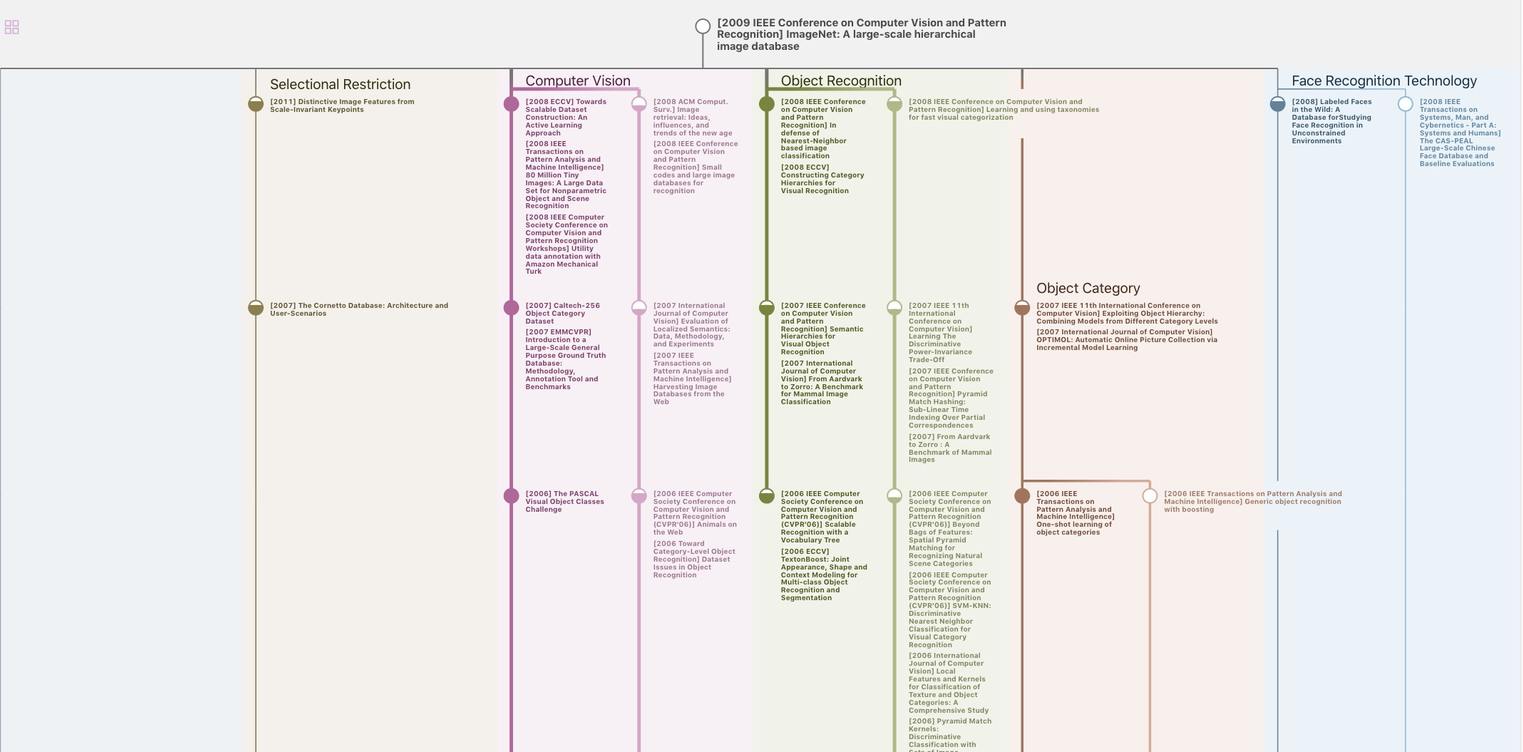
生成溯源树,研究论文发展脉络
Chat Paper
正在生成论文摘要