Towards scalable deployment of Hidden Markov models in occupancy estimation: A novel methodology applied to the study case of occupancy detection
ENERGY AND BUILDINGS(2022)
摘要
Occupancy detection and estimation are two of the main areas of research in smart buildings. This is due to its significant effect in the deployment of energy saving buildings and various other futuristic user centered applications. Occupancy detection refers to the determination of presence of occupants in a space or smart building, whereas occupancy estimation represents the exact determination of the number of occupants. Recently, machine learning approaches have made momentous advancements in the smart buildings field. Hidden Markov models (HMMs) have played an important role in such amelioration. Consequently, in this paper, we propose and comprehensively study a novel approach for the employment of HMMs in occupancy applications of smart buildings. In particular, the benchmark approach in the literature relies on a state-based model deployment. Hence, an implicit assumption is made that the states would automatically represent the physical phenomena of the data. This is not always the case. Our proposed framework promises a scalable stable deployment of HMMs, particularly in relation to the status quo, with model selection criteria for the determination of the number of states. Our extensive experiments show considerable improvement across the various evaluation metrics. Finally, this work also establishes multiple promising venues of future investigation for practitioners and researchers in the field, of which we discuss some of, especially at the intersection of machine learning and smart buildings. (c) 2021 Elsevier B.V. All rights reserved.
更多查看译文
关键词
Hidden Markov models, Machine learning, Occupancy detection, Smart buildings
AI 理解论文
溯源树
样例
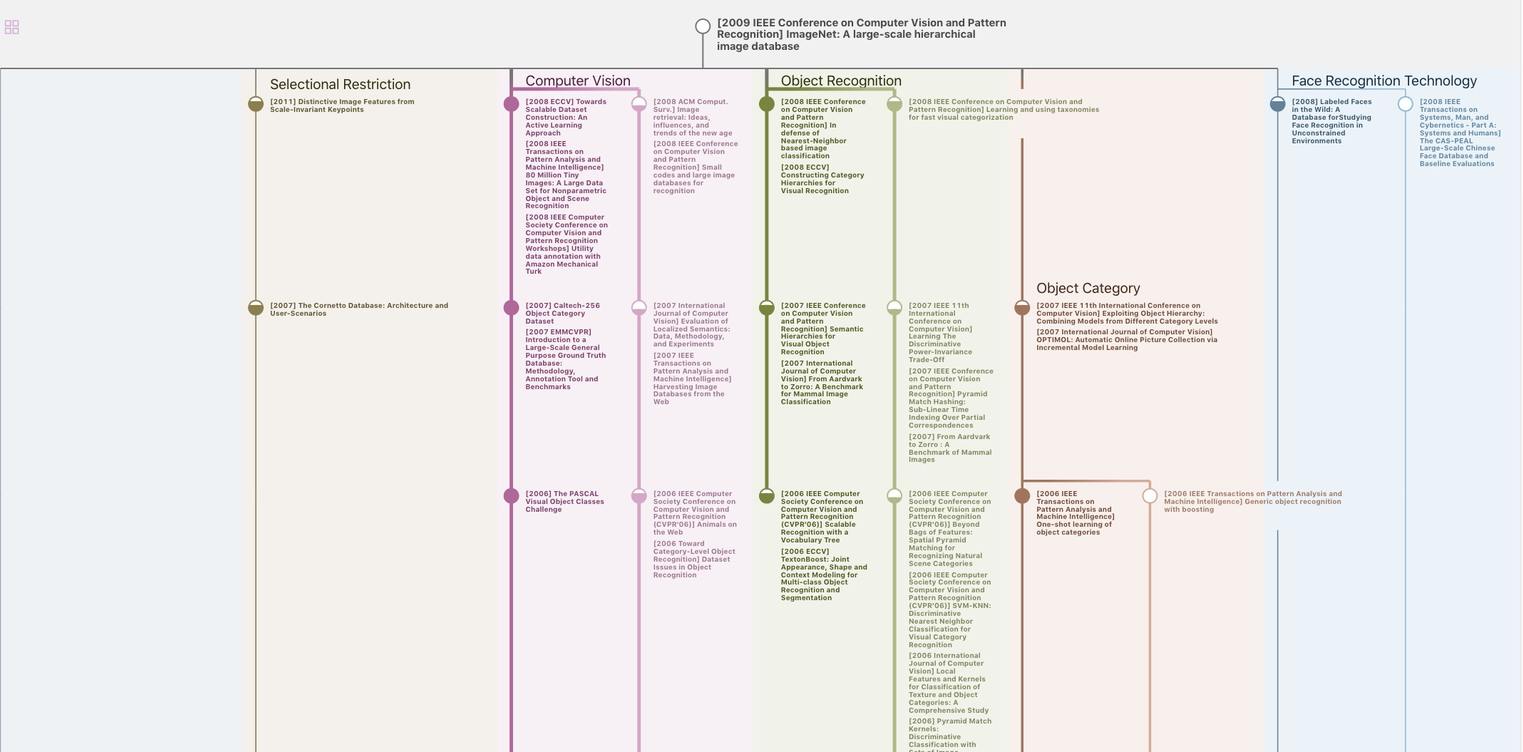
生成溯源树,研究论文发展脉络
Chat Paper
正在生成论文摘要