Forecasting hotel daily room demand with transformed data using time series methods
Journal of Revenue and Pricing Management(2021)
摘要
Increasing tourism and economic growth have led to a remarkably increase in demand and competition for hotel business in Thailand. This paper aims to explore the benefits of data transforming to help forecast hotel daily room demand for a case-study hotel. The proposed results can be a forecasting framework for other hotels with similar demand patterns. The case-study hotel is a local 4-star, 97-room hotel in Thailand. The recorded data of daily room demand from 2016 to 2019 are used. For room demand forecasting, two different datasets of daily demand are used, i.e., pre-processed data and transformed data by smoothing technique. Different time series forecasting models are performed: (1) Same day last year, (2) Holt–Winters, (3) Seasonal Autoregressive integrated moving average (SARIMA), and (4) Box–Jenkins Box–Cox transformation trigonometric ARMA errors trend and multiple seasonal patterns. We compared the accuracy of each model in forecasting of room demand with the actual room occupancies in 2019. The model accuracy is evaluated using mean absolute error (MAE), mean absolute percentage error (MAPE), and median absolute percentage error (MdAPE). It is found that SARIMA using transformed dataset provided the best accuracy (MAE, 6.18; MAPE, 25.04%; MdAPE, 13.64%) and best fit with plots for 2-week forecast horizon of room demand data. This paper introduces the use of transformed dataset to increase the performance of SARIMA model, as compared to the pre-processed data. To our knowledge, unlike other research, this paper proposed the method of data pre-processing and data smoothing to deal with the high variation in room demand data.
更多查看译文
关键词
Hotel demand forecasting, Hotel revenue management, Time series model, SARIMA
AI 理解论文
溯源树
样例
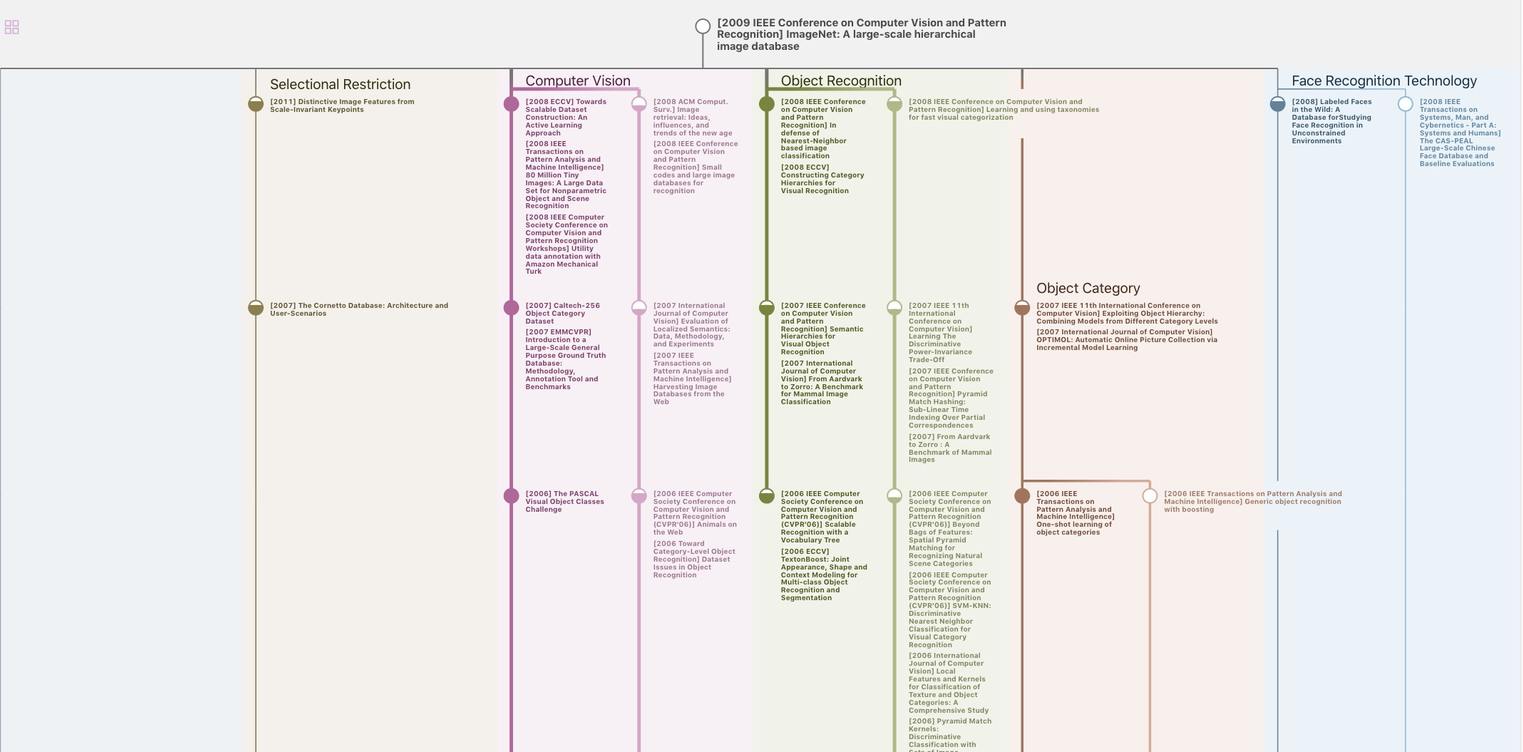
生成溯源树,研究论文发展脉络
Chat Paper
正在生成论文摘要