Profiling Casualty Severity Levels of Road Accident Using Weighted Majority Voting
CMC-COMPUTERS MATERIALS & CONTINUA(2022)
摘要
To determine the individual circumstances that account for a road traffic accident, it is crucial to consider the unplanned connections amongst various factors related to a crash that results in high casualty levels. Analysis of the road accident data concentrated mainly on categorizing accidents into different types using individually built classification methods which limit the prediction accuracy and fitness of the model. In this article, we proposed a multi-model hybrid framework of the weighted majority voting (WMV) scheme with parallel structure, which is designed by integrating individually implemented multinomial logistic regression (MLR) and multilayer perceptron (MLP) classifiers using three different accident datasets i.e., IRTAD, NCDB, and FARS. The proposed WMV hybrid scheme overtook individual classifiers in terms of modern evaluation measures like ROC, RMSE, Kappa rate, classification accuracy, and performs better than state-of-the-art approaches for the prediction of casualty severity level. Moreover, the proposed WMV hybrid scheme adds up to accident severity analysis through knowledge representation by revealing the role of different accident-related factors which expand the risk of casualty in a road crash. Critical aspects related to casualty severity recognized by the proposed WMV hybrid approach can surely support the traffic enforcement agencies to develop better road safety plans and ultimately save lives.
更多查看译文
关键词
Prediction, hybrid framework, severity, class, casualty
AI 理解论文
溯源树
样例
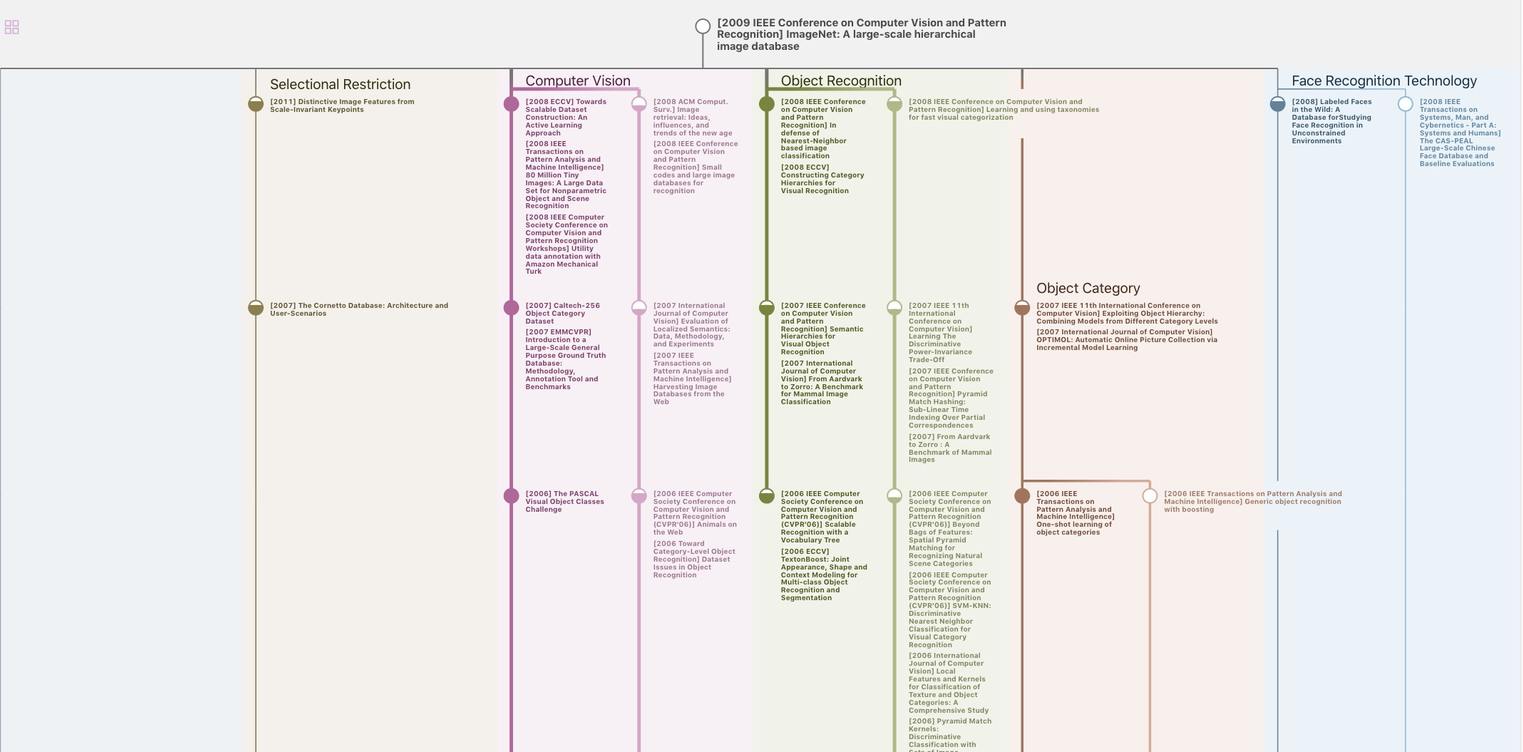
生成溯源树,研究论文发展脉络
Chat Paper
正在生成论文摘要