MGRL: attributed multiplex heterogeneous network representation learning based on multi-granularity information fusion
International Journal of Machine Learning and Cybernetics(2022)
摘要
Nowadays, attributed multiplex heterogeneous network (AMHN) representation learning has shown superiority in many network analysis tasks due to its ability to preserve both the structure of the network and the semantics of the nodes. However, few people consider the correlation between content attributes within each node. No personalized analysis model is designed for different semantics of heterogeneous relations. To address these issues, this paper proposes an MGRL model. MGRL adopts a filter based on variance discrimination to filter out the noise information in the node content attributes. To better utilize semantic characteristics of heterogeneous relations, personalized fusion models are designed according to heterogeneous relation categories: peer relations and subordinate relations. Results of experiments conducted on three real-world datasets show an obvious advantage of the proposed MGRL model over state-of-the-art baseline methods.
更多查看译文
关键词
Network analysis,Attributed multiplex heterogeneous network,Heterogeneous neighbors sampling,Type-fusion model,Variance weight,Multi-granularity information,Representation learning
AI 理解论文
溯源树
样例
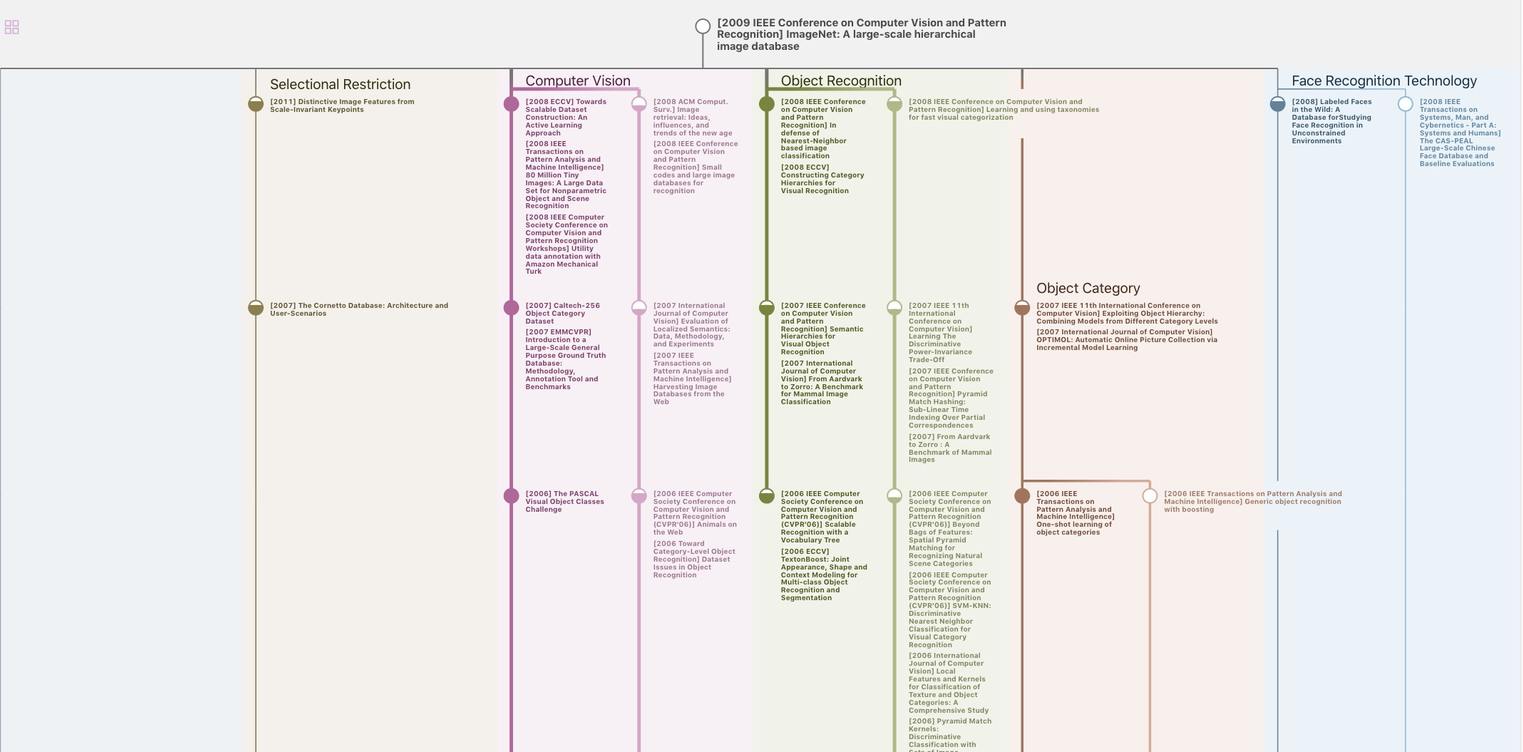
生成溯源树,研究论文发展脉络
Chat Paper
正在生成论文摘要