SCC-rFMQ: a multiagent reinforcement learning method in cooperative Markov games with continuous actions
International Journal of Machine Learning and Cybernetics(2022)
摘要
Although many multiagent reinforcement learning (MARL) methods have been proposed for learning the optimal solutions in continuous-action domains, multiagent cooperation domains with independent learners (ILs) have received relatively few investigations, especially in traditional RL domain. In this paper, we propose an sample based independent learning method, named Sample Continuous Coordination with recursive Frequency Maximum Q-Value (SCC-rFMQ), which divides the multiagent cooperative problem with continuous actions into two layers. The first layer samples a finite set of actions from the continuous action spaces by a re-sampling mechanism with variable exploratory rates, and the second layer evaluates the actions in the sampled action set and updates the policy using a reinforcement learning cooperative method. By constructing cooperative mechanisms at both levels, SCC-rFMQ can handle cooperative problems in continuous action cooperative Markov games effectively. The effectiveness of SCC-rFMQ is experimentally demonstrated on two well-designed games, i.e., a continuous version of the climbing game and a cooperative version of the boat problem. Experimental results show that SCC-rFMQ outperforms other reinforcement learning algorithms.
更多查看译文
关键词
Cooperative Markov games, Reinforcement learning, Continuous action space, Multiagent learning
AI 理解论文
溯源树
样例
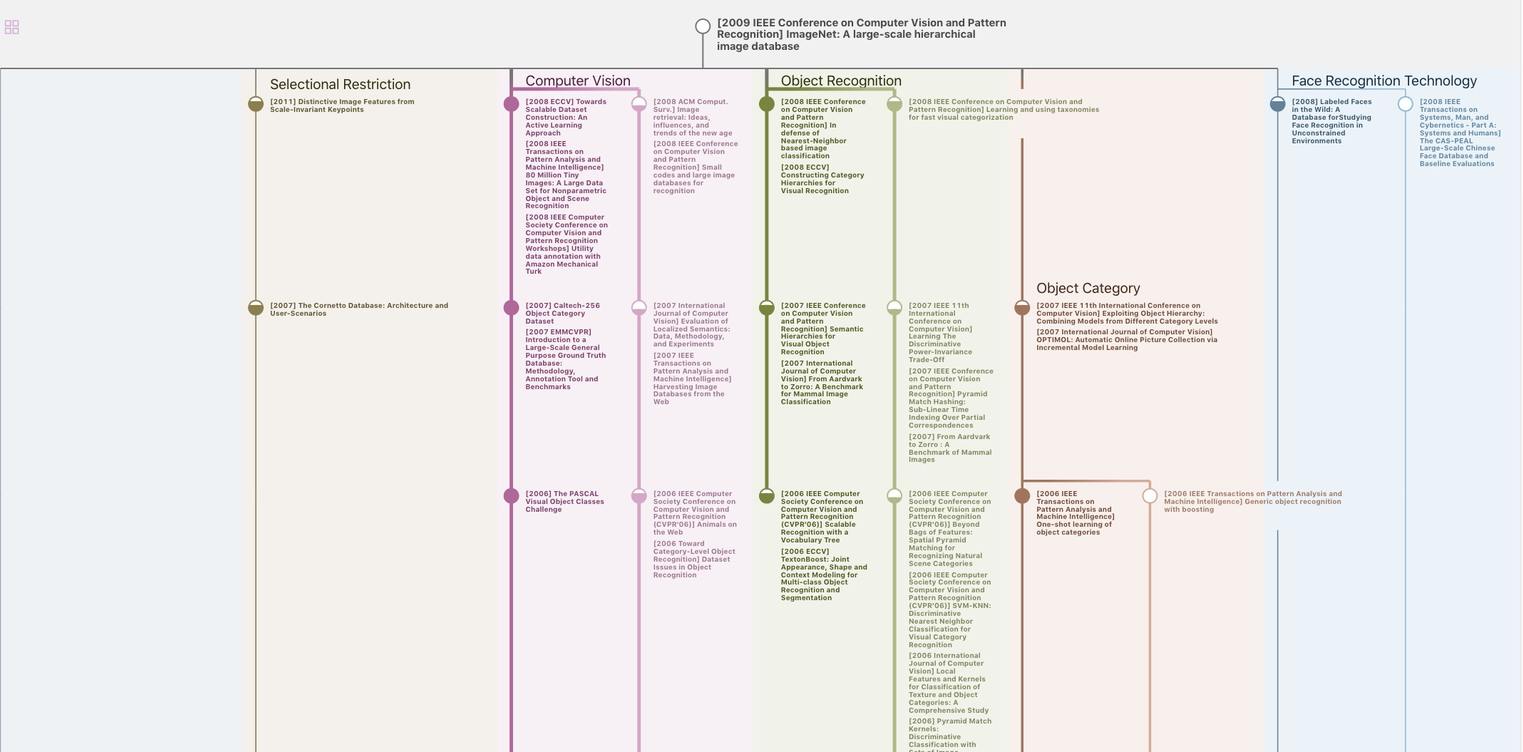
生成溯源树,研究论文发展脉络
Chat Paper
正在生成论文摘要