Epoch-Evolving Gaussian Process Guided Learning for Classification
IEEE TRANSACTIONS ON NEURAL NETWORKS AND LEARNING SYSTEMS(2024)
摘要
The conventional mini-batch gradient descent algorithms are usually trapped in the local batch-level distribution information, resulting in the ``zig-zag'' effect in the learning process. To characterize the correlation information between the batch-level distribution and the global data distribution, we propose a novel learning scheme called epoch-evolving Gaussian process guided learning (GPGL) to encode the global data distribution information in a non-parametric way. Upon a set of class-aware anchor samples, our GP model is built to estimate the class distribution for each sample in mini-batch through label propagation from the anchor samples to the batch samples. The class distribution, also named the context label, is provided as a complement for the ground-truth one-hot label. Such a class distribution structure has a smooth property and usually carries a rich body of contextual information that is capable of speeding up the convergence process. With the guidance of the context label and ground-truth label, the GPGL scheme provides a more efficient optimization through updating the model parameters with a triangle consistency loss. Furthermore, our GPGL scheme can be generalized and naturally applied to the current deep models, outperforming the state-of-the-art optimization methods on six benchmark datasets.
更多查看译文
关键词
Computational modeling,Pipelines,Deep learning,Context modeling,Predictive models,Feature extraction,Data models,Gaussian process (GP),global distribution-aware learning,non-parametric modeling,top-down strategy
AI 理解论文
溯源树
样例
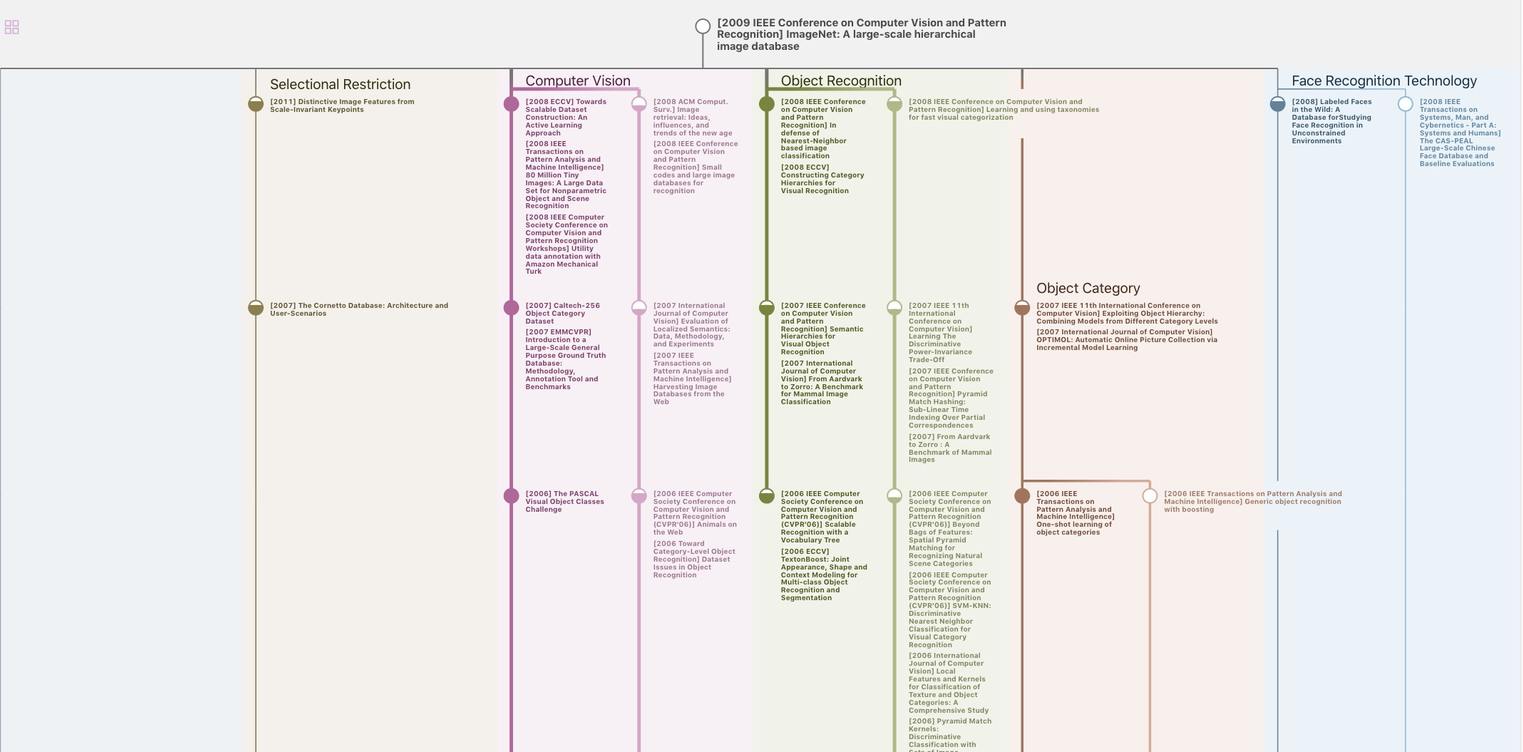
生成溯源树,研究论文发展脉络
Chat Paper
正在生成论文摘要