LLRHNet: Multiple Lesions Segmentation Using Local-Long Range Features
FRONTIERS IN NEUROINFORMATICS(2022)
摘要
The encoder-decoder-based deep convolutional neural networks (CNNs) have made great improvements in medical image segmentation tasks. However, due to the inherent locality of convolution, CNNs generally are demonstrated to have limitations in obtaining features across layers and long-range features from the medical image. In this study, we develop a local-long range hybrid features network (LLRHNet), which inherits the merits of the iterative aggregation mechanism and the transformer technology, as a medical image segmentation model. LLRHNet adopts encoder-decoder architecture as the backbone which iteratively aggregates the projection and up-sampling to fuse local low-high resolution features across isolated layers. The transformer adopts the multi-head self-attention mechanism to extract long-range features from the tokenized image patches and fuses these features with the local-range features extracted by down-sampling operation in the backbone network. These hybrid features are used to assist the cascaded up-sampling operations to local the position of the target tissues. LLRHNet is evaluated on two multiple lesions medical image data sets, including a public liver-related segmentation data set (3DIRCADb) and an in-house stroke and white matter hyperintensity (SWMH) segmentation data set. Experimental results denote that LLRHNet achieves state-of-the-art performance on both data sets.
更多查看译文
关键词
iterative aggregation, transformer, image patches, long-range features, multiple lesions
AI 理解论文
溯源树
样例
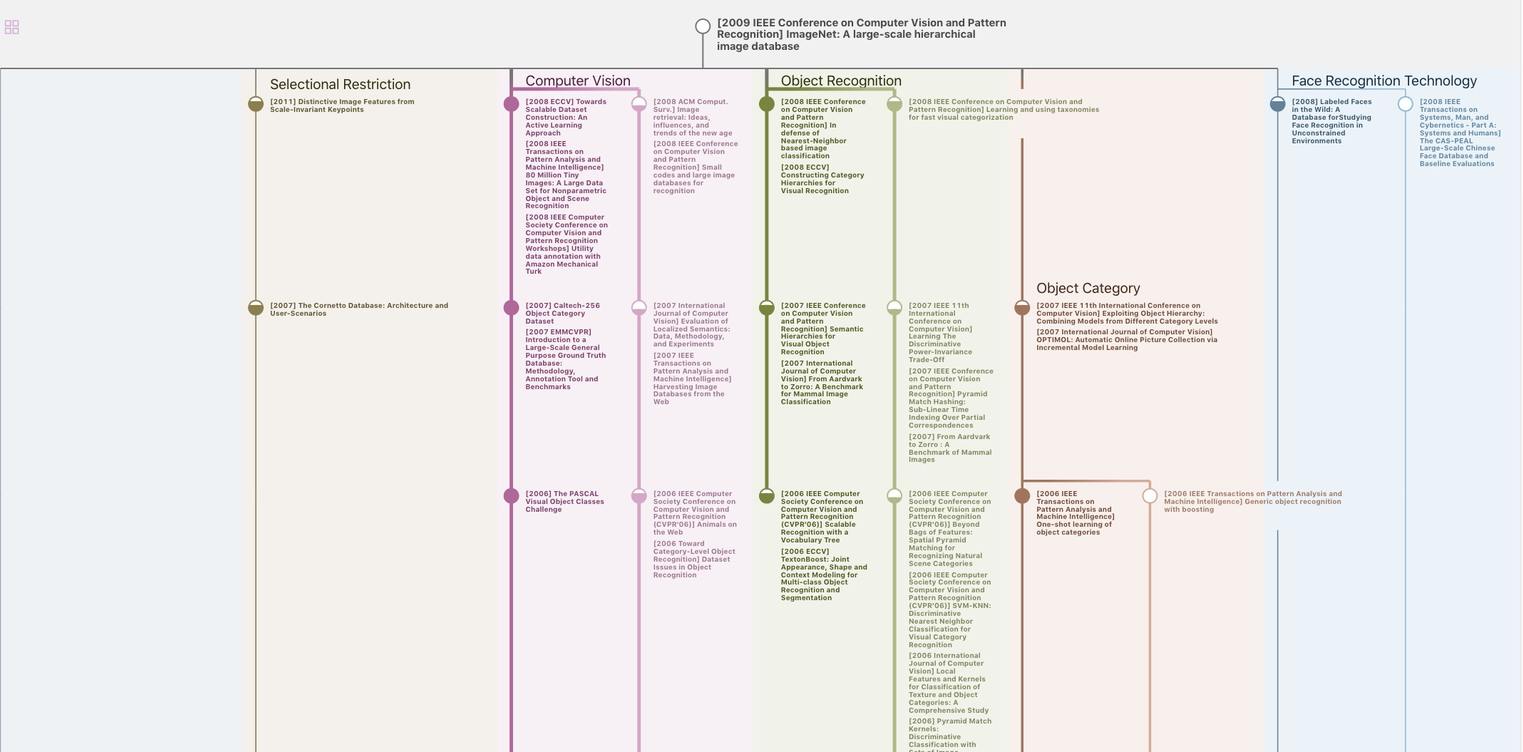
生成溯源树,研究论文发展脉络
Chat Paper
正在生成论文摘要