Topic Modeling for Interpretable Text Classification From EHRs
FRONTIERS IN BIG DATA(2022)
摘要
The clinical notes in electronic health records have many possibilities for predictive tasks in text classification. The interpretability of these classification models for the clinical domain is critical for decision making. Using topic models for text classification of electronic health records for a predictive task allows for the use of topics as features, thus making the text classification more interpretable. However, selecting the most effective topic model is not trivial. In this work, we propose considerations for selecting a suitable topic model based on the predictive performance and interpretability measure for text classification. We compare 17 different topic models in terms of both interpretability and predictive performance in an inpatient violence prediction task using clinical notes. We find no correlation between interpretability and predictive performance. In addition, our results show that although no model outperforms the other models on both variables, our proposed fuzzy topic modeling algorithm (FLSA-W) performs best in most settings for interpretability, whereas two state-of-the-art methods (ProdLDA and LSI) achieve the best predictive performance.
更多查看译文
关键词
text classification, topic modeling, explainability, interpretability, electronic health records, psychiatry, natural language processing, information extraction
AI 理解论文
溯源树
样例
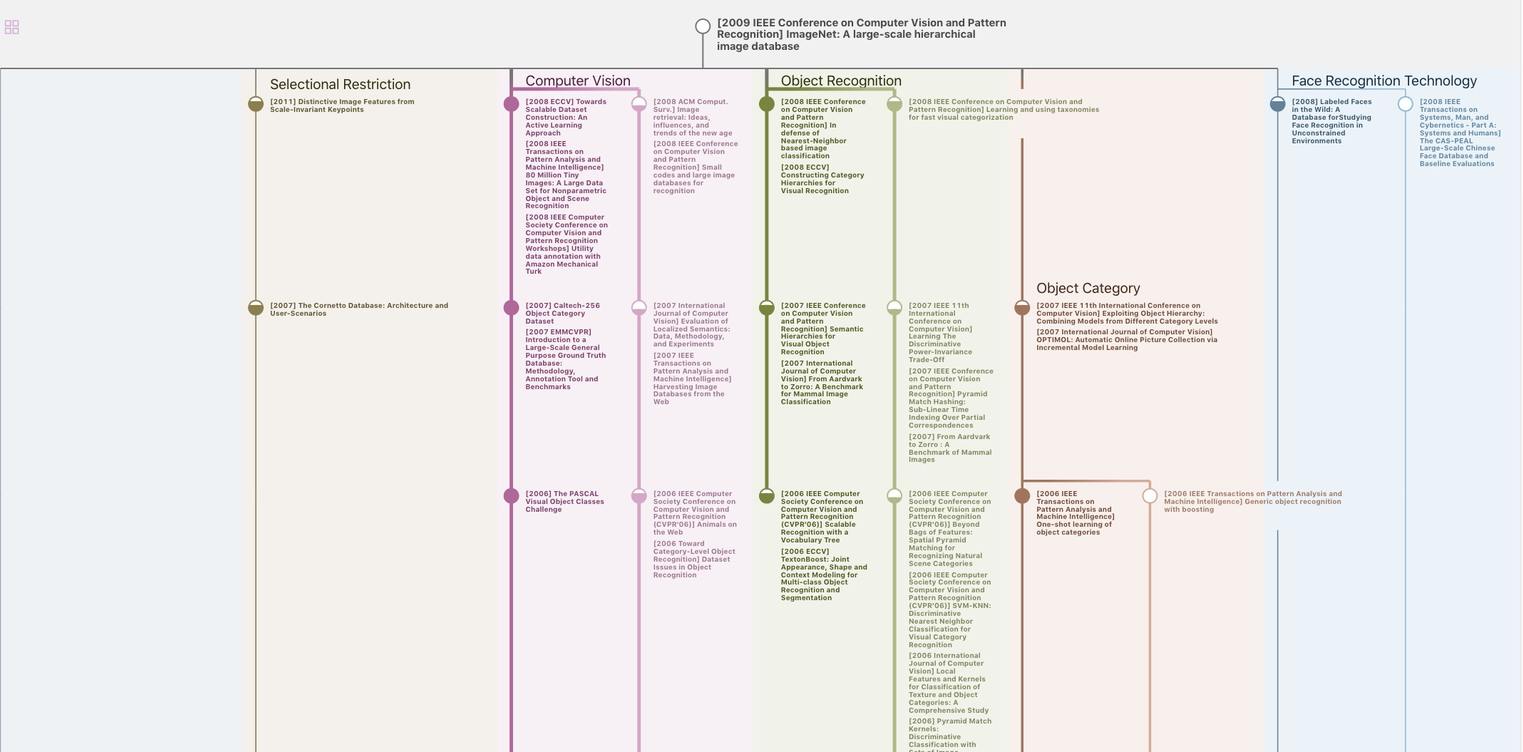
生成溯源树,研究论文发展脉络
Chat Paper
正在生成论文摘要