Meta-analysis under imbalance in measurement of confounders in cohort studies using only summary-level data
BMC Medical Research Methodology(2022)
摘要
Background Cohort collaborations often require meta-analysis of exposure-outcome association estimates across cohorts as an alternative to pooling individual-level data that requires a laborious process of data harmonization on individual-level data. However, it is likely that important confounders are not all measured uniformly across the cohorts due to differences in study protocols. This imbalance in measurement of confounders leads to association estimates that are not comparable across cohorts and impedes the meta-analysis of results. Methods In this article, we empirically show some asymptotic relations between fully adjusted and unadjusted exposure-outcome effect estimates, and provide theoretical justification for the same. We leverage these results to obtain fully adjusted estimates for the cohorts with no information on confounders by borrowing information from cohorts with complete measurement on confounders. We implement this novel method in CIMBAL (confounder imbalance), which additionally provides a meta-analyzed estimate that appropriately accounts for the dependence between estimates arising due to borrowing of information across cohorts. We perform extensive simulation experiments to study CIMBAL’s statistical properties. We illustrate CIMBAL using National Children’s Study (NCS) data to estimate association of maternal education and low birth weight in infants, adjusting for maternal age at delivery, race/ethnicity, marital status, and income. Results Our simulation studies indicate that estimates of exposure-outcome association from CIMBAL are closer to the truth than those from commonly-used approaches for meta-analyzing cohorts with disparate confounder measurements. CIMBAL is not too sensitive to heterogeneity in underlying joint distributions of exposure, outcome and confounders but is very sensitive to heterogeneity of confounding bias across cohorts. Application of CIMBAL to NCS data for a proof-of-concept analysis further illustrates the utility and advantages of CIMBAL. Conclusions CIMBAL provides a practical approach for meta-analyzing cohorts with imbalance in measurement of confounders under a weak assumption that the cohorts are independently sampled from populations with the same confounding bias.
更多查看译文
关键词
Bias,Collective analysis,Confounder imbalance,Data integration,Meta-analysis,Omitted covariate,Omitted variable bias,Regression estimates
AI 理解论文
溯源树
样例
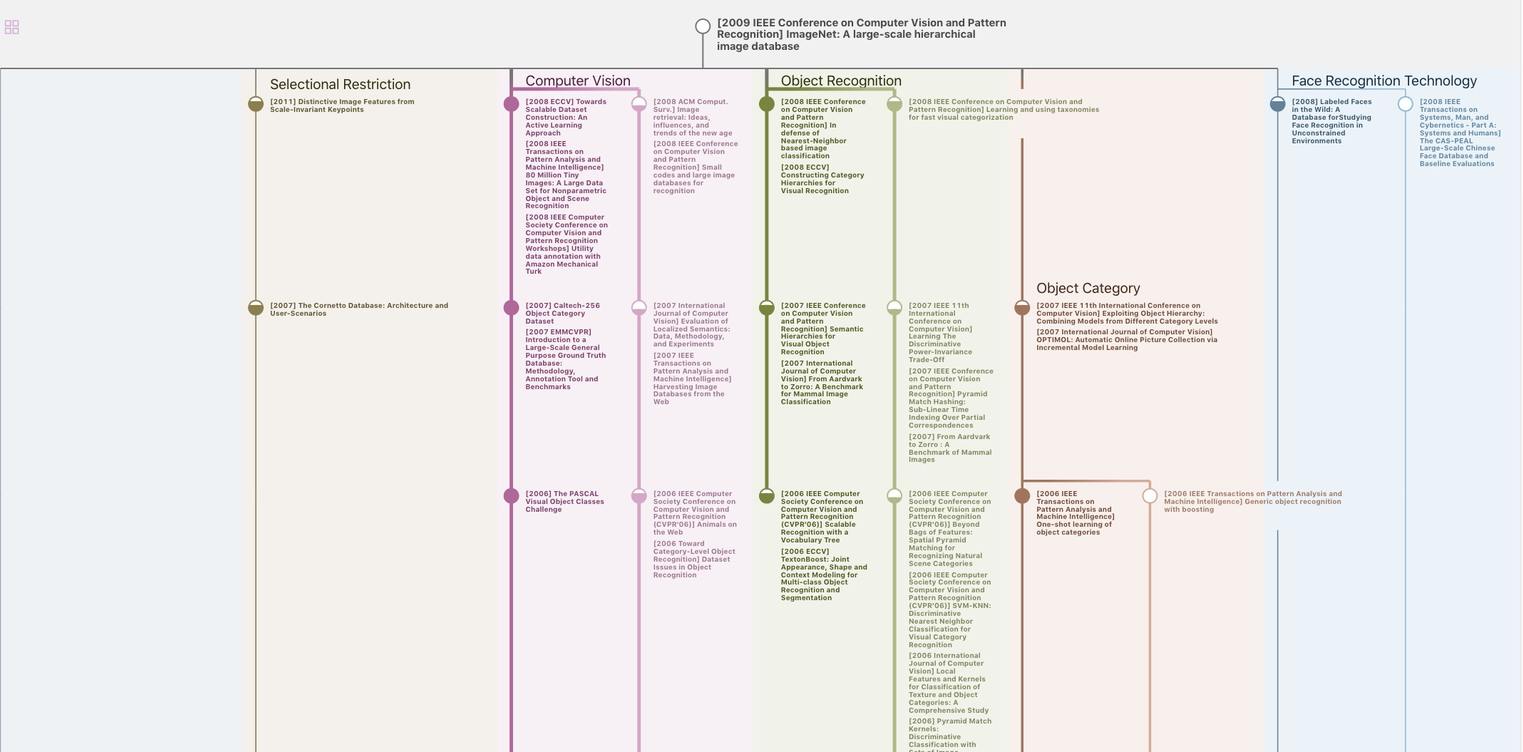
生成溯源树,研究论文发展脉络
Chat Paper
正在生成论文摘要