Neural Lyapunov Differentiable Predictive Control
arxiv(2022)
摘要
We present a learning-based predictive control methodology using the differentiable programming framework with probabilistic Lyapunov-based stability guarantees. The neural Lyapunov differentiable predictive control (NLDPC) learns the policy by constructing a computational graph encompassing the system dynamics, state and input constraints, and the necessary Lyapunov certification constraints, and thereafter using the automatic differentiation to update the neural policy parameters. In conjunction, our approach jointly learns a Lyapunov function that certifies the regions of state-space with stable dynamics. We also provide a sampling-based statistical guarantee for the training of NLDPC from the distribution of initial conditions. Our offline training approach provides a computationally efficient and scalable alternative to classical explicit model predictive control solutions. We substantiate the advantages of the proposed approach with simulations to stabilize the double integrator model and on an example of controlling an aircraft model.
更多查看译文
关键词
control
AI 理解论文
溯源树
样例
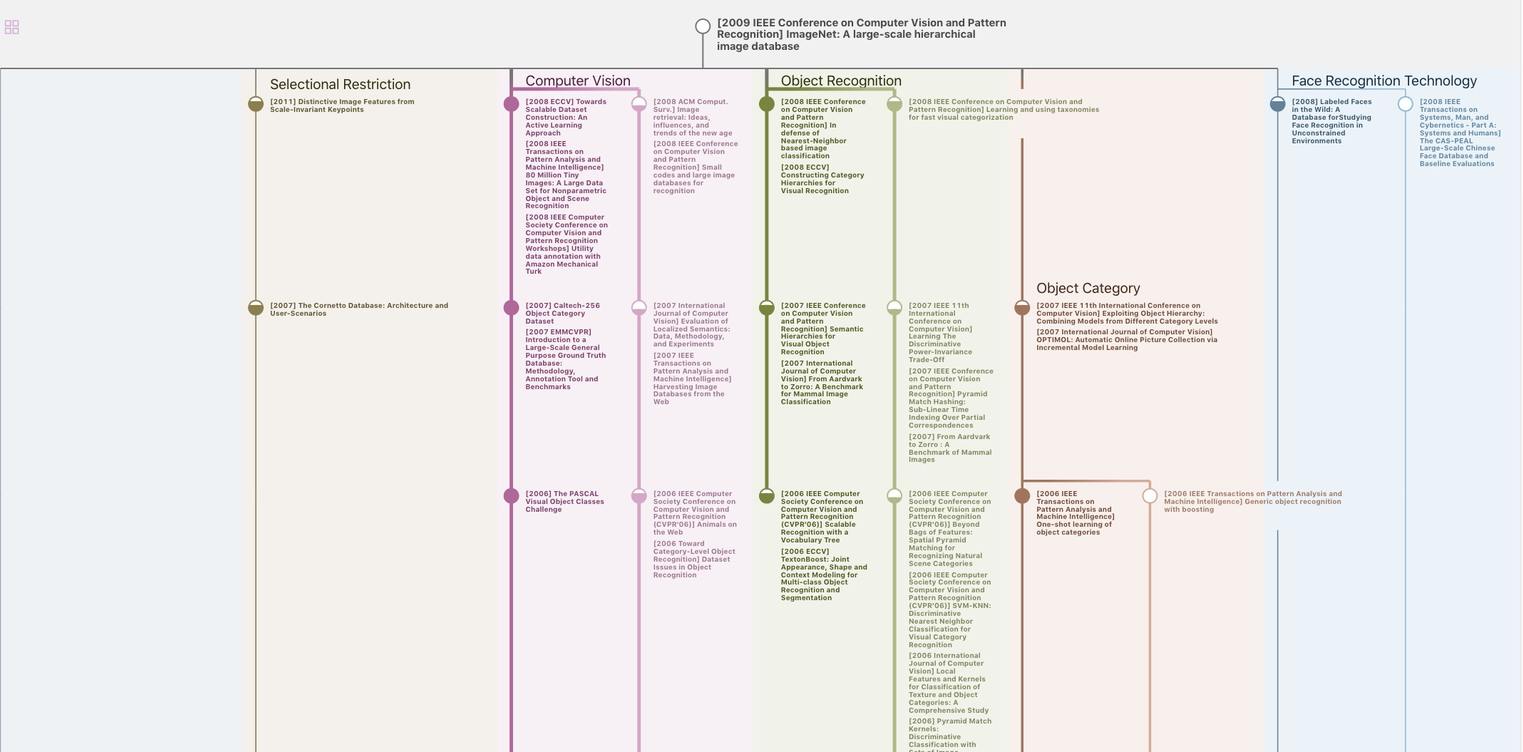
生成溯源树,研究论文发展脉络
Chat Paper
正在生成论文摘要