Kernel Normalized Convolutional Networks
arxiv(2022)
摘要
Existing deep convolutional neural network (CNN) architectures frequently rely upon batch normalization (BatchNorm) to effectively train the model. BatchNorm significantly improves model performance in centralized training, but it is unsuitable for federated learning and differential privacy settings. Even in centralized learning, BatchNorm performs poorly with smaller batch sizes. To address these limitations, we propose kernel normalization and kernel normalized convolutional layers, and incorporate them into kernel normalized convolutional networks (KNConvNets) as the main building blocks. We implement KNConvNets corresponding to the state-of-the-art CNNs such as VGGNets and ResNets while forgoing BatchNorm layers. Through extensive experiments, we illustrate KNConvNets consistently outperform their batch, group, and layer normalized counterparts in terms of both accuracy and convergence rate in centralized, federated, and differentially private learning settings.
更多查看译文
关键词
kernel,networks
AI 理解论文
溯源树
样例
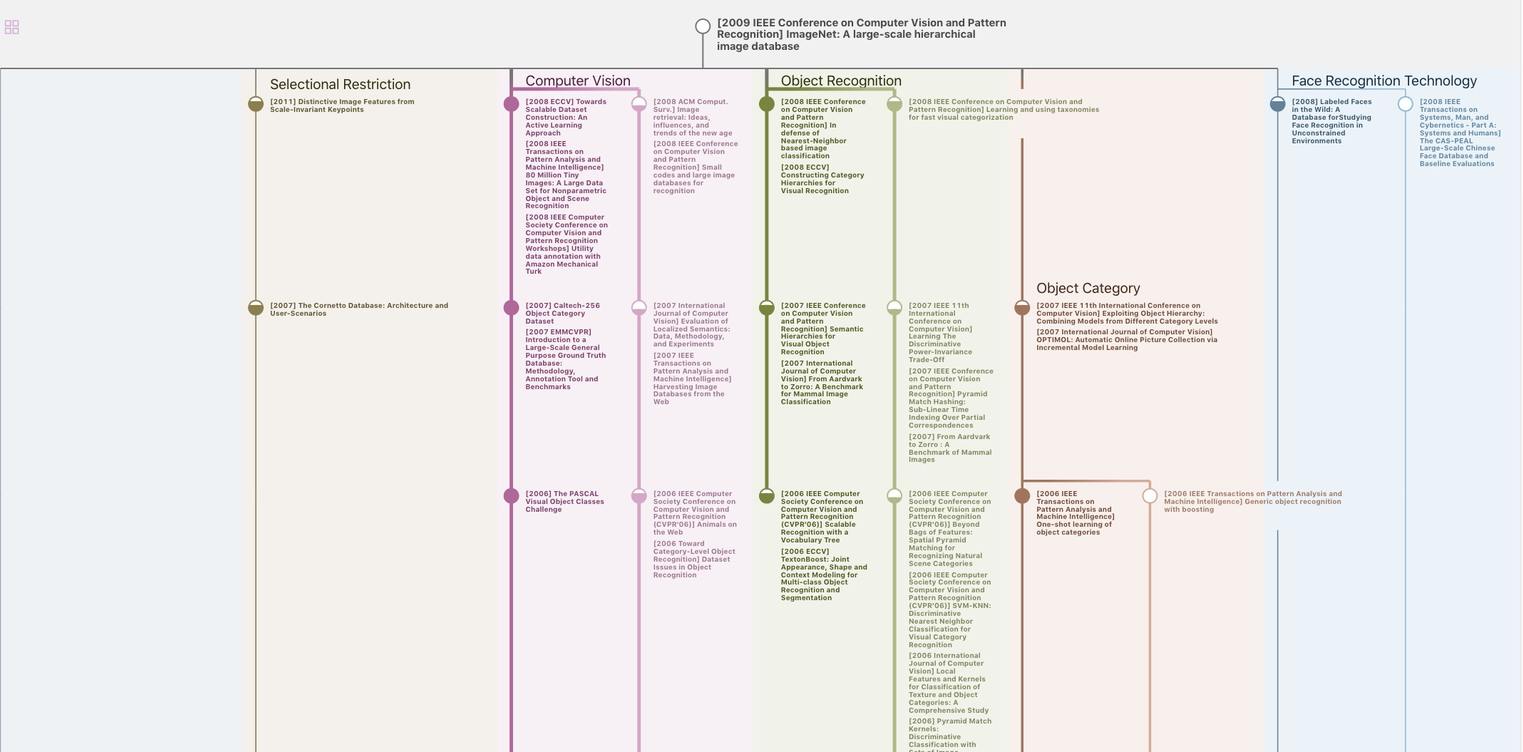
生成溯源树,研究论文发展脉络
Chat Paper
正在生成论文摘要