The Unreasonable Effectiveness of Deep Evidential Regression
arxiv(2022)
摘要
There is a significant need for principled uncertainty reasoning in machine learning systems as they are increasingly deployed in safety-critical domains. A new approach with uncertainty-aware regression-based neural networks (NNs), based on learning evidential distributions for aleatoric and epistemic uncertainties, shows promise over traditional deterministic methods and typical Bayesian NNs, notably with the capabilities to disentangle aleatoric and epistemic uncertainties. Despite some empirical success of Deep Evidential Regression (DER), there are important gaps in the mathematical foundation that raise the question of why the proposed technique seemingly works. We detail the theoretical shortcomings and analyze the performance on synthetic and real-world data sets, showing that Deep Evidential Regression is a heuristic rather than an exact uncertainty quantification. We go on to propose corrections and redefinitions of how aleatoric and epistemic uncertainties should be extracted from NNs.
更多查看译文
关键词
unreasonable effectiveness,deep,regression
AI 理解论文
溯源树
样例
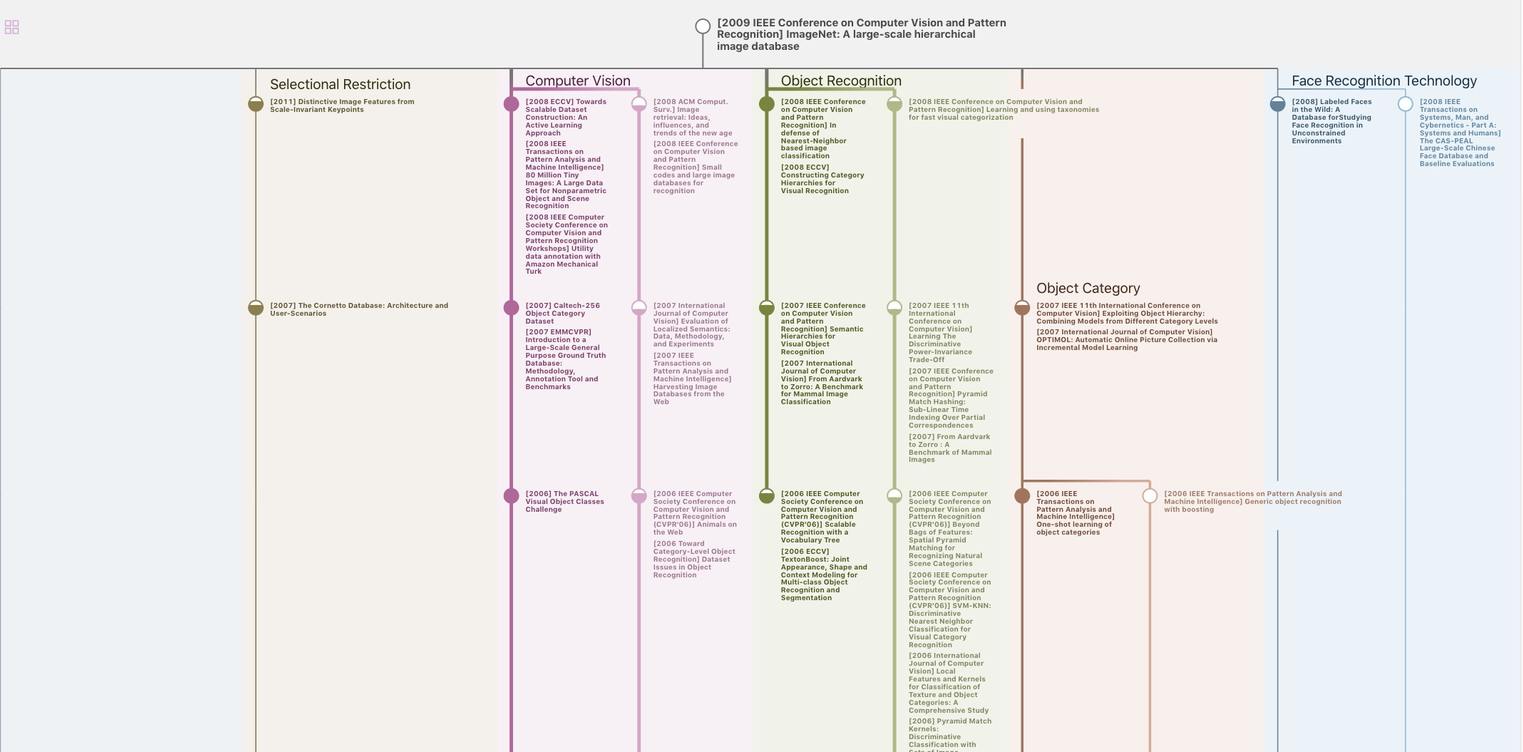
生成溯源树,研究论文发展脉络
Chat Paper
正在生成论文摘要