Robust expected information gain for optimal Bayesian experimental design using ambiguity sets.
International Conference on Uncertainty in Artificial Intelligence(2022)
摘要
The ranking of experiments by expected information gain (EIG) in Bayesian experimental design is sensitive to changes in the model’s prior distribution, and the approximation of EIG yielded by sampling will have errors similar to the use of a perturbed prior. We define and analyze Robust Expected Information Gain(REIG), a modification of the objective in EIG maximization by minimizing an affine relaxation of EIG over an ambiguity set of distributions that are close to the original prior in KL-divergence. We show that, when combined with a sampling-based approach to estimating EIG, REIG corresponds to a "log-sum-exp" stabilization of the samples used to estimate EIG, meaning that it can be efficiently implemented in practice. Numerical tests combining REIG with variational nested Monte Carlo (VNMC), adaptive contrastive estimation (ACE) and mutual information neural estimation (MINE) suggest that in practice REIG also compensates for the variability of under-sampled estimators.
更多查看译文
关键词
optimal bayesian experimental
AI 理解论文
溯源树
样例
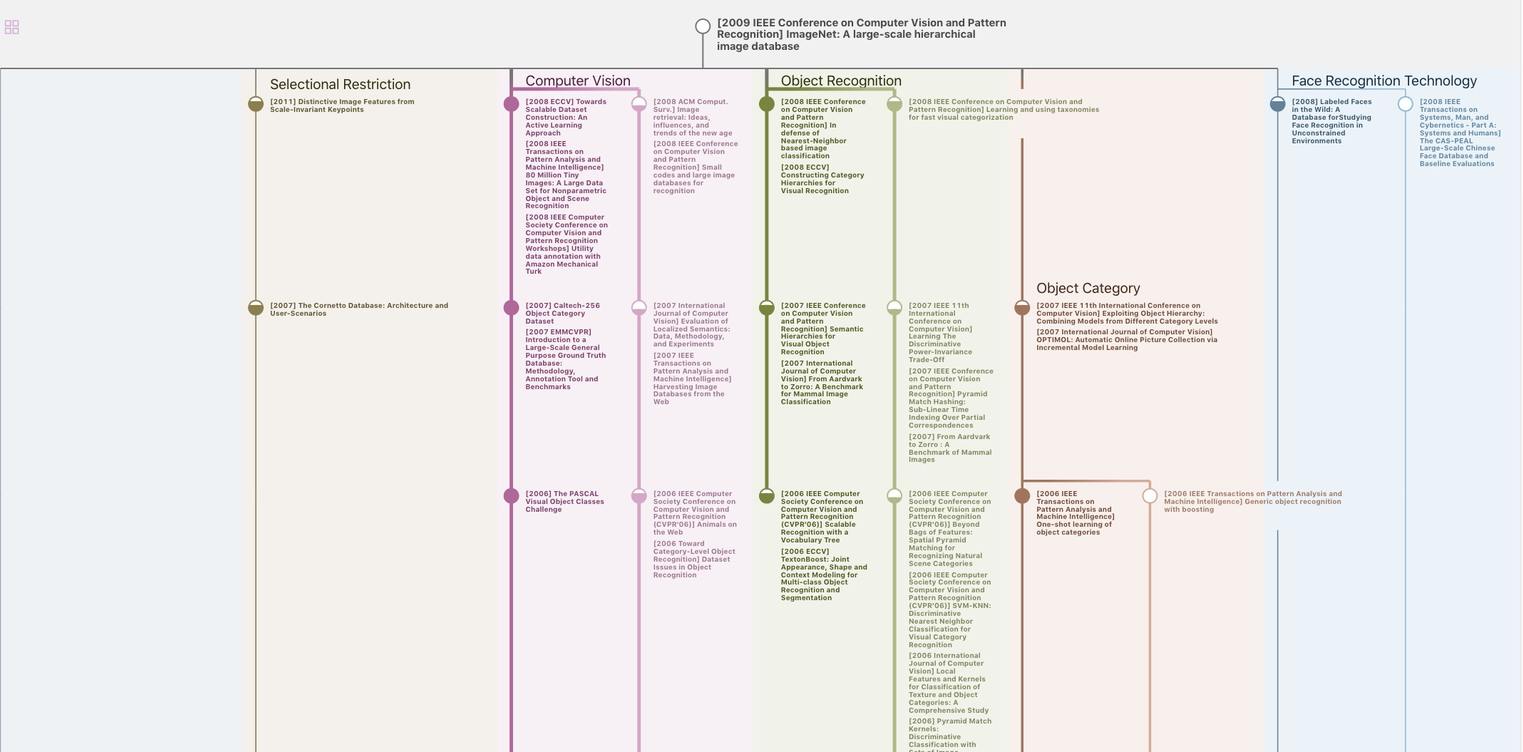
生成溯源树,研究论文发展脉络
Chat Paper
正在生成论文摘要