Calibration Matters: Tackling Maximization Bias in Large-scale Advertising Recommendation Systems.
ICLR 2023(2023)
摘要
Calibration is defined as the ratio of the average predicted click rate to the true click rate. The optimization of calibration is essential to many online advertising recommendation systems because it directly affects the downstream bids in ads auctions and the amount of money charged to advertisers. Despite its importance, calibration optimization often suffers from a problem called "maximization bias". Maximization bias refers to the phenomenon that the maximum of predicted values overestimates the true maximum. The problem is introduced because the calibration is computed on the set selected by the prediction model itself. It persists even if unbiased predictions can be achieved on every datapoint and worsens when covariate shifts exist between the training and test sets. To mitigate this problem, we theorize the quantification of maximization bias and propose a variance-adjusting debiasing (VAD) meta-algorithm in this paper. The algorithm is efficient, robust, and practical as it is able to mitigate maximization bias problems under covariate shifts, neither incurring additional online serving costs nor compromising the ranking performance. We demonstrate the effectiveness of the proposed algorithm using a state-of-the-art recommendation neural network model on a large-scale real-world dataset.
更多查看译文
关键词
Maximization bias,calibration,distribution shifts,neural networks,recommendation system,computational advertisement
AI 理解论文
溯源树
样例
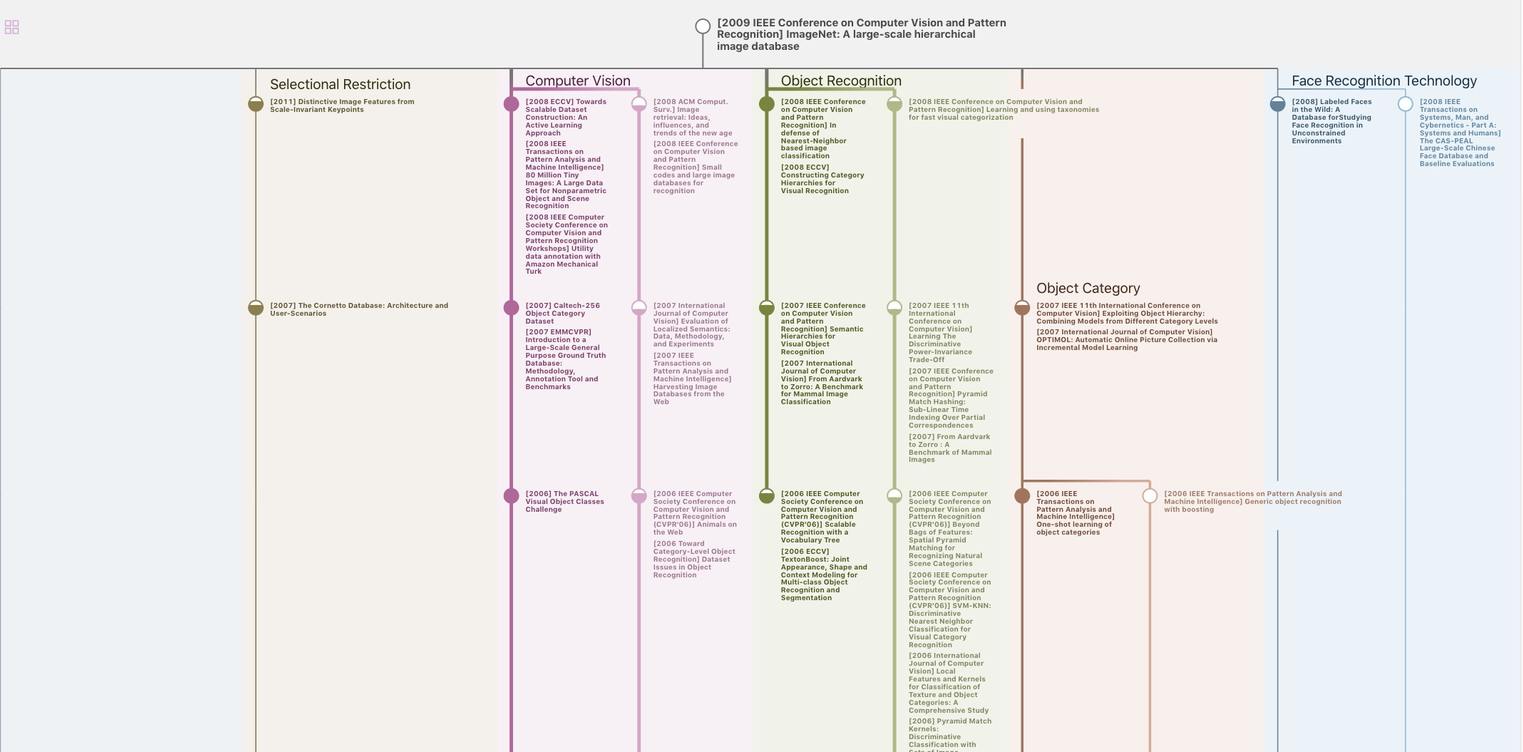
生成溯源树,研究论文发展脉络
Chat Paper
正在生成论文摘要