Estimation of Entropy in Constant Space with Improved Sample Complexity
NeurIPS 2022(2022)
摘要
Recent work of Acharya et al. (NeurIPS 2019) showed how to estimate the entropy of a distribution $\mathcal D$ over an alphabet of size $k$ up to $\pm\epsilon$ additive error by streaming over $(k/\epsilon^3) \cdot \text{polylog}(1/\epsilon)$ i.i.d. samples and using only $O(1)$ words of memory. In this work, we give a new constant memory scheme that reduces the sample complexity to $(k/\epsilon^2)\cdot \text{polylog}(1/\epsilon)$. We conjecture that this is optimal up to $\text{polylog}(1/\epsilon)$ factors.
更多查看译文
关键词
Sample complexity,Data streams,Shannon Entropy
AI 理解论文
溯源树
样例
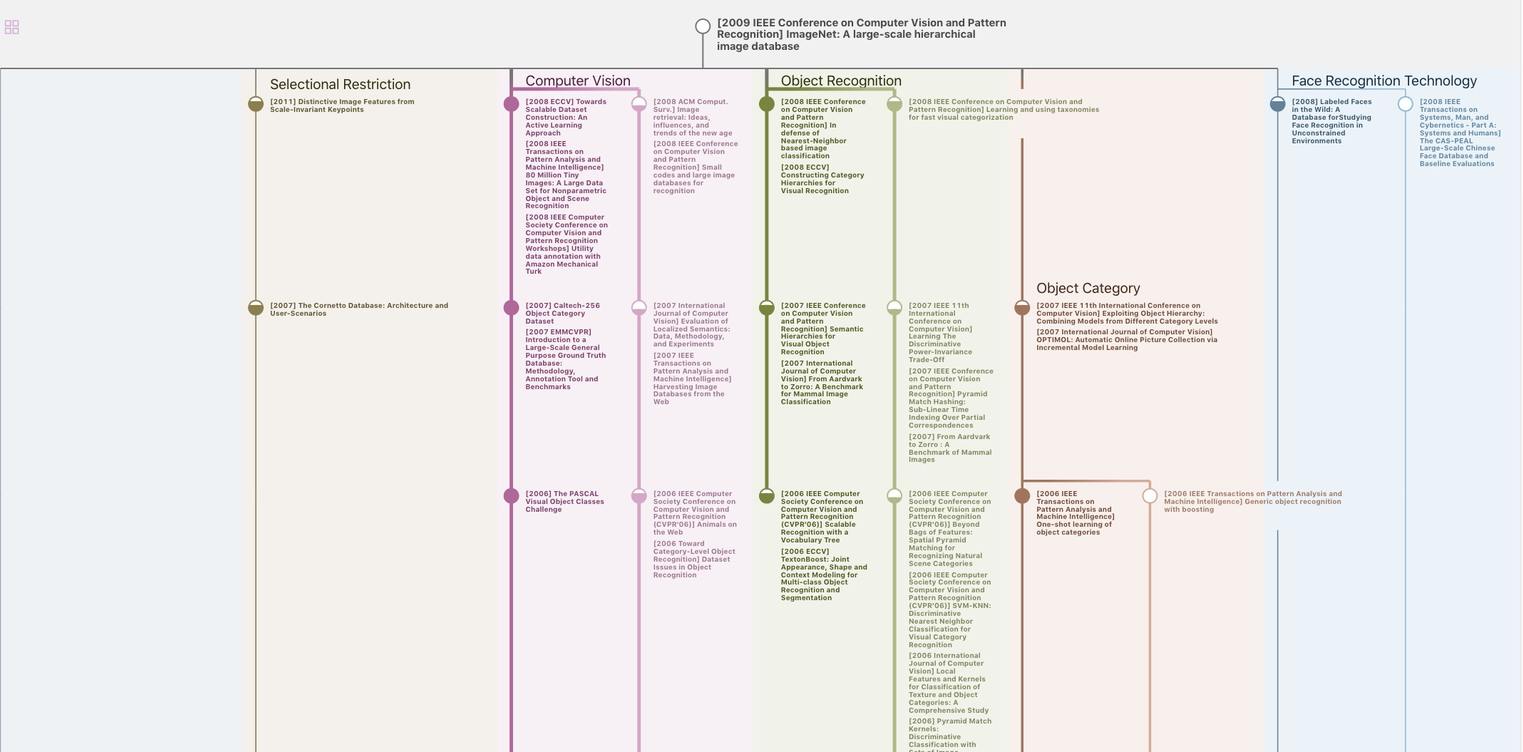
生成溯源树,研究论文发展脉络
Chat Paper
正在生成论文摘要