A Feature Selection Approach Guided an Early Prediction of Anthocyanin Accumulation Using Massive Untargeted Metabolomics Data in Mulberry
PLANT AND CELL PHYSIOLOGY(2022)
摘要
Identifying the early predictive biomarkers or compounds represents a pivotal task for guiding a targeted agricultural practice. Despite the various available tools, it remains challenging to define the ideal compound combination and thereby elaborate an effective predictive model fitting that. Hence, we employed a stepwise feature selection approach followed by a maximum relevance and minimum redundancy (MRMR) on the untargeted metabolism in four mulberry genotypes at different fruit developmental stages (FDSs). Thus, we revealed that 7 out of 226 differentially abundant metabolites (DAMs) explained up to 80% variance of anthocyanin based on linear regression model and stepwise feature selection approach accompanied by an MRMR across the genotypes over the FDSs. Among them, the phosphoenolpyruvate, d-mannose and shikimate show the top 3 attribution indexes to the accumulation of anthocyanin in the fruits of these genotypes across the four FDSs. The obtained results were further validated by assessing the regulatory genes expression levels and the targeted metabolism approach. Taken together, our findings provide valuable evidences on the fact that the anthocyanin biosynthesis is somehow involved in the coordination between the carbon metabolism and secondary metabolic pathway. Our report highlights as well the importance of using the feature selection approach for the predictive biomarker identification issued from the untargeted metabolomics data.
更多查看译文
关键词
Anthocyanin, Feature selection, Machine learning, Metabolomics, Mulberry, Univariate statistics
AI 理解论文
溯源树
样例
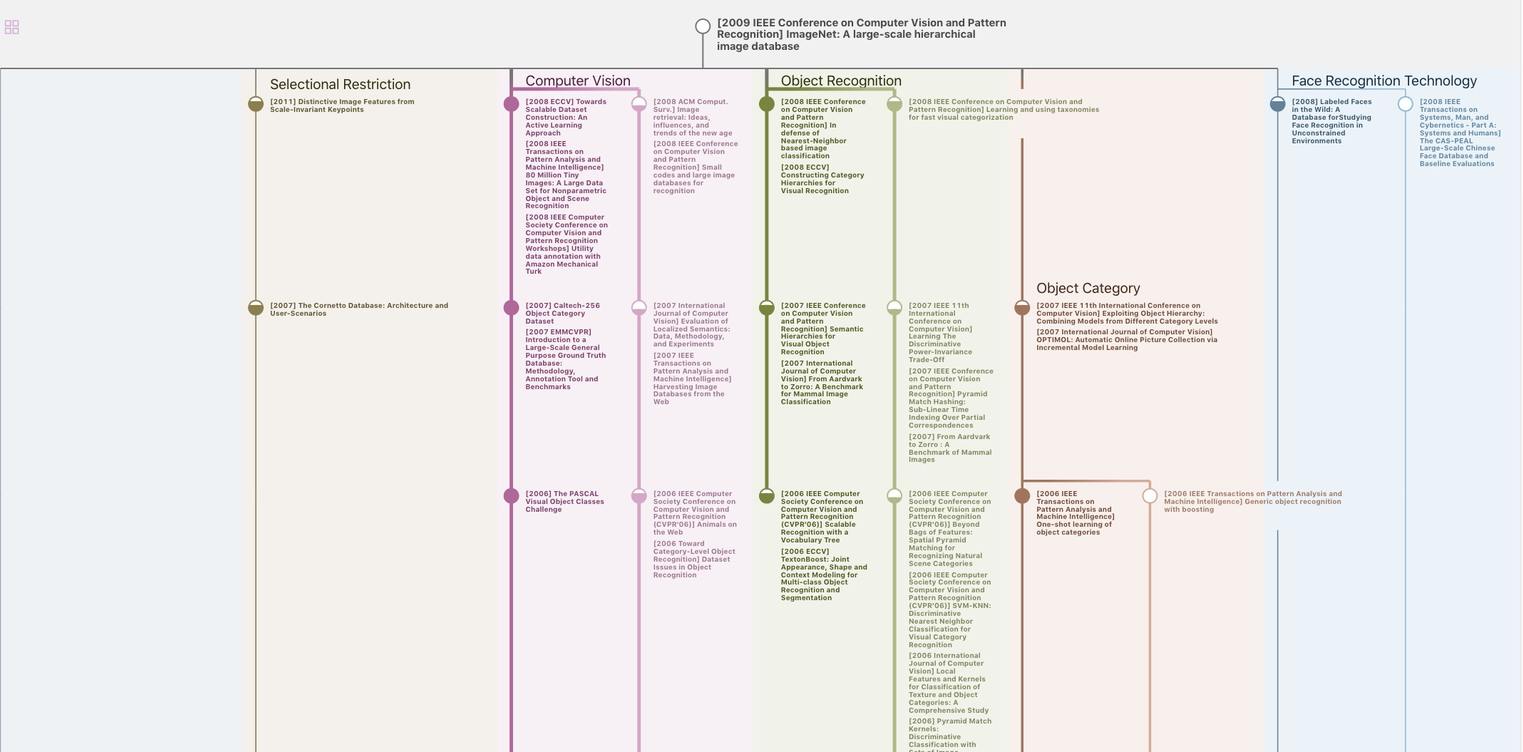
生成溯源树,研究论文发展脉络
Chat Paper
正在生成论文摘要