New Intent Discovery with Pre-training and Contrastive Learning
PROCEEDINGS OF THE 60TH ANNUAL MEETING OF THE ASSOCIATION FOR COMPUTATIONAL LINGUISTICS (ACL 2022), VOL 1: (LONG PAPERS)(2022)
摘要
New intent discovery aims to uncover novel intent categories from user utterances to expand the set of supported intent classes. It is a critical task for the development and service expansion of a practical dialogue system. Despite its importance, this problem remains under-explored in the literature. Existing approaches typically rely on a large amount of labeled utterances and employ pseudo-labeling methods for representation learning and clustering, which are label-intensive, inefficient, and inaccurate. In this paper, we provide new solutions to two important research questions for new intent discovery: (1) how to learn semantic utterance representations and (2) how to better cluster utterances. Particularly, we first propose a multi-task pre-training strategy to leverage rich unlabeled data along with external labeled data for representation learning. Then, we design a new contrastive loss to exploit self-supervisory signals in unlabeled data for clustering. Extensive experiments on three intent recognition benchmarks demonstrate the high effectiveness of our proposed method, which outperforms state-of-the-art methods by a large margin in both unsupervised and semi-supervised scenarios. The source code will be available at https://github.com/zhang-yu-wei/MTP-CLNN.
更多查看译文
关键词
new intent discovery,contrastive learning,pre-training
AI 理解论文
溯源树
样例
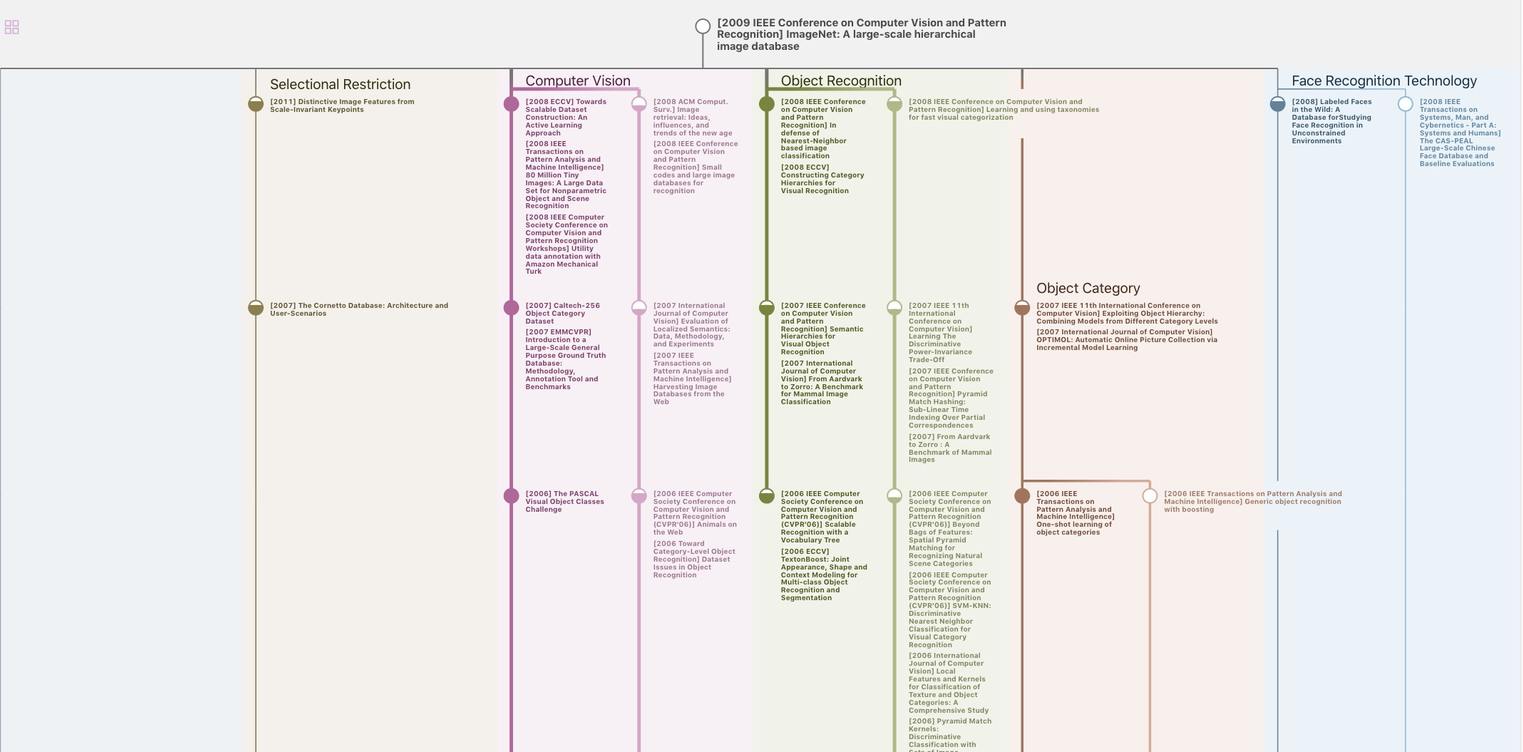
生成溯源树,研究论文发展脉络
Chat Paper
正在生成论文摘要