Unsupervised Dependency Graph Network
PROCEEDINGS OF THE 60TH ANNUAL MEETING OF THE ASSOCIATION FOR COMPUTATIONAL LINGUISTICS (ACL 2022), VOL 1: (LONG PAPERS)(2022)
摘要
Recent work has identified properties of pre-trained self-attention models that mirror those of dependency parse structures. In particular, some self-attention heads correspond well to individual dependency types. Inspired by these developments, we propose a new competitive mechanism that encourages these attention heads to model different dependency relations. We introduce a new model, the Unsupervised Dependency Graph Network (UDGN), that can induce dependency structures from raw corpora and the masked language modeling task. Experiment results show that UDGN achieves very strong unsupervised dependency parsing performance without gold POS tags and any other external information. The competitive gated heads show a strong correlation with human-annotated dependency types. Furthermore, the UDGN can also achieve competitive performance on masked language modeling and sentence textual similarity tasks (1).
更多查看译文
关键词
graph,network
AI 理解论文
溯源树
样例
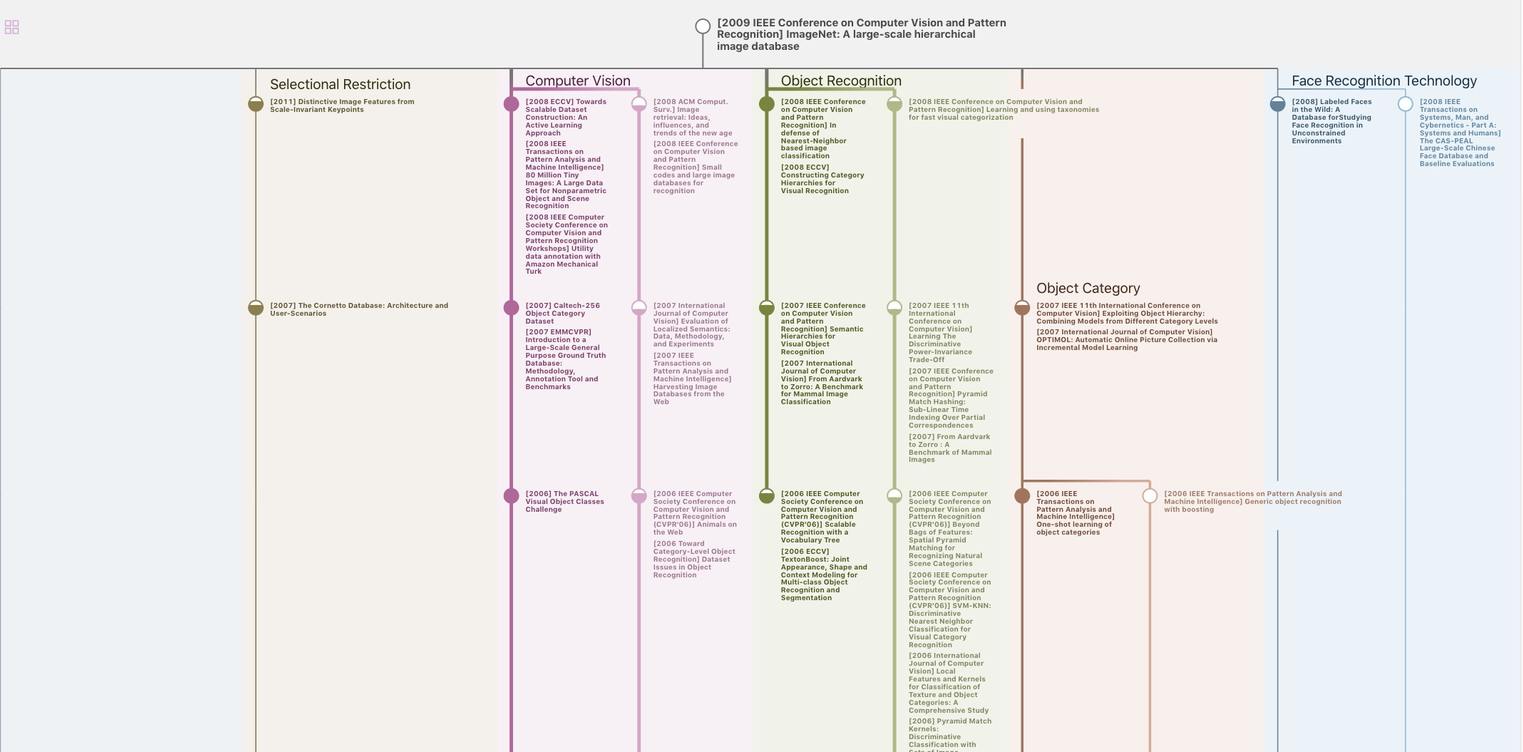
生成溯源树,研究论文发展脉络
Chat Paper
正在生成论文摘要