Hard and Soft Evaluation of NLP models with BOOtSTrap SAmpling - BooStSa
PROCEEDINGS OF THE 60TH ANNUAL MEETING OF THE ASSOCIATION FOR COMPUTATIONAL LINGUISTICS (ACL 2022): PROCEEDINGS OF SYSTEM DEMONSTRATIONS(2022)
摘要
Natural Language Processing (NLP)'s applied nature makes it necessary to select the most effective and robust models. However, just producing slightly higher performance is insufficient; we want to know whether this advantage will carry over to other data sets. Bootstrapped significance tests can indicate that ability. Computing the significance of performance differences has many levels of complexity, though. It can be tedious, especially when the experimental design has many conditions to compare and several runs of experiments. We present BooStSa, a tool that makes it easy to compute significance levels with the BOOtSTrap SAmpling procedure. BooStSa can evaluate models that predict not only standard hard labels but soft labels (i.e., probability distributions over different classes) as well.
更多查看译文
AI 理解论文
溯源树
样例
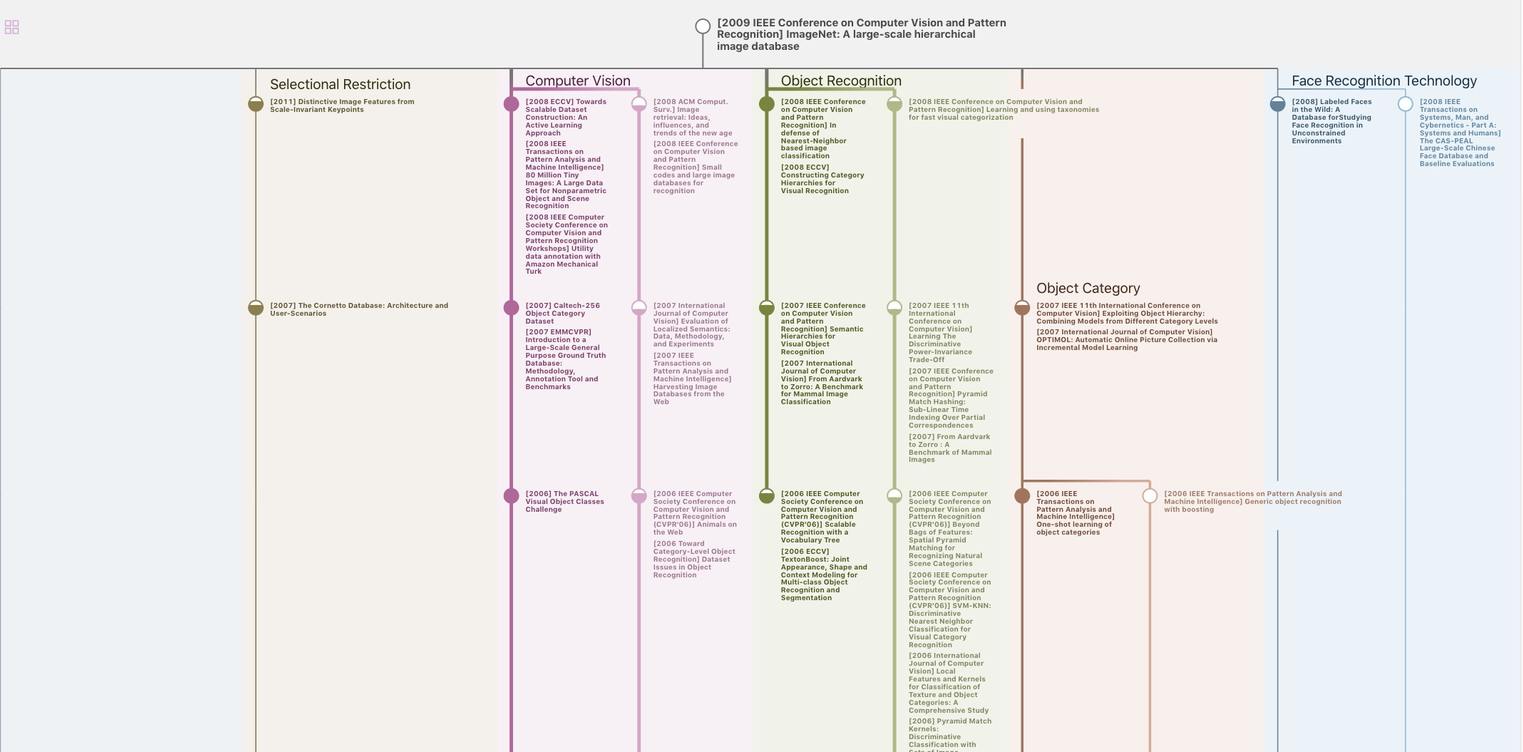
生成溯源树,研究论文发展脉络
Chat Paper
正在生成论文摘要