Ranking-Constrained Learning with Rationales for Text Classification
FINDINGS OF THE ASSOCIATION FOR COMPUTATIONAL LINGUISTICS (ACL 2022)(2022)
摘要
We propose a novel approach that jointly utilizes the labels and elicited rationales for text classification to speed up the training of deep learning models with limited training data. We define and optimize a ranking-constrained loss function that combines cross-entropy loss with ranking losses as rationale constraints. We evaluate our proposed rationale-augmented learning approach on three human-annotated datasets, and show that our approach provides significant improvements over classification approaches that do not utilize rationales as well as other state-of-the-art rationale-augmented baselines.
更多查看译文
关键词
text classification,rationales,learning,ranking-constrained
AI 理解论文
溯源树
样例
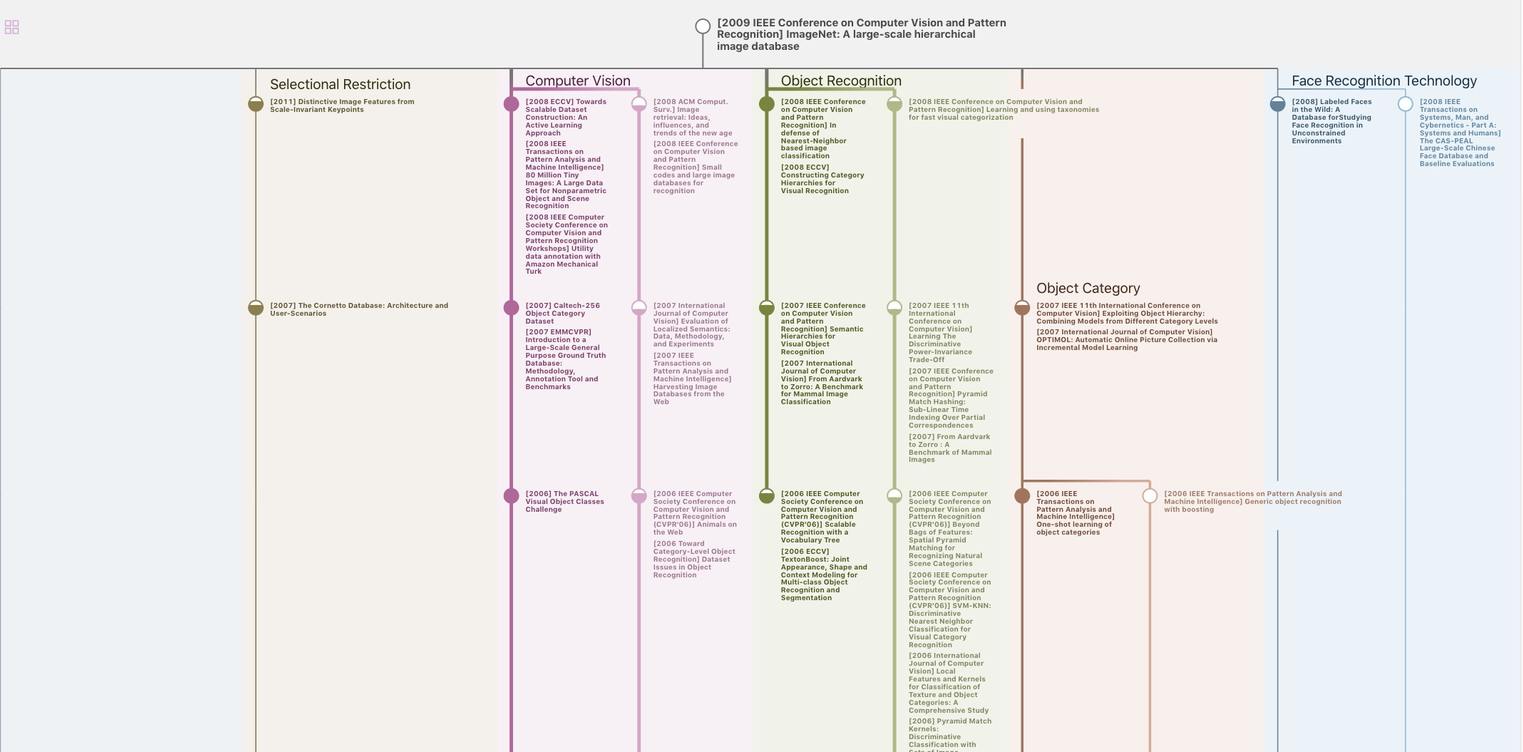
生成溯源树,研究论文发展脉络
Chat Paper
正在生成论文摘要