Prior Knowledge and Memory Enriched Transformer for Sign Language Translation
FINDINGS OF THE ASSOCIATION FOR COMPUTATIONAL LINGUISTICS (ACL 2022)(2022)
摘要
This paper attacks the challenging problem of sign language translation (SLT), which involves not only visual and textual understanding but also additional prior knowledge learning (i.e. performing style, syntax). However, the majority of existing methods with vanilla encoder-decoder structures fail to sufficiently explore all of them. Based on this concern, we propose a novel method called Prior knowledge and memory Enriched Transformer (PET) for SLT, which incorporates the auxiliary information into vanilla transformer. Concretely, we develop gated interactive multi-head attention which associates the multimodal representation and global signing style with adaptive gated functions. One Part-of-Speech (POS) sequence generator relies on the associated information to predict the global syntactic structure, which is thereafter leveraged to guide the sentence generation. Besides, considering that the visualtextual context information, and additional auxiliary knowledge of a word may appear in more than one video, we design a multi-stream memory structure to obtain higher-quality translations, which stores the detailed correspondence between a word and its various relevant information, leading to a more comprehensive understanding for each word. We conduct extensive empirical studies on RWTH-PHOENIX-Weather-2014T dataset with both signer-dependent and signer-independent conditions. The quantitative and qualitative experimental results comprehensively reveal the effectiveness of PET.
更多查看译文
关键词
memory enriched transformer,sign,knowledge,translation
AI 理解论文
溯源树
样例
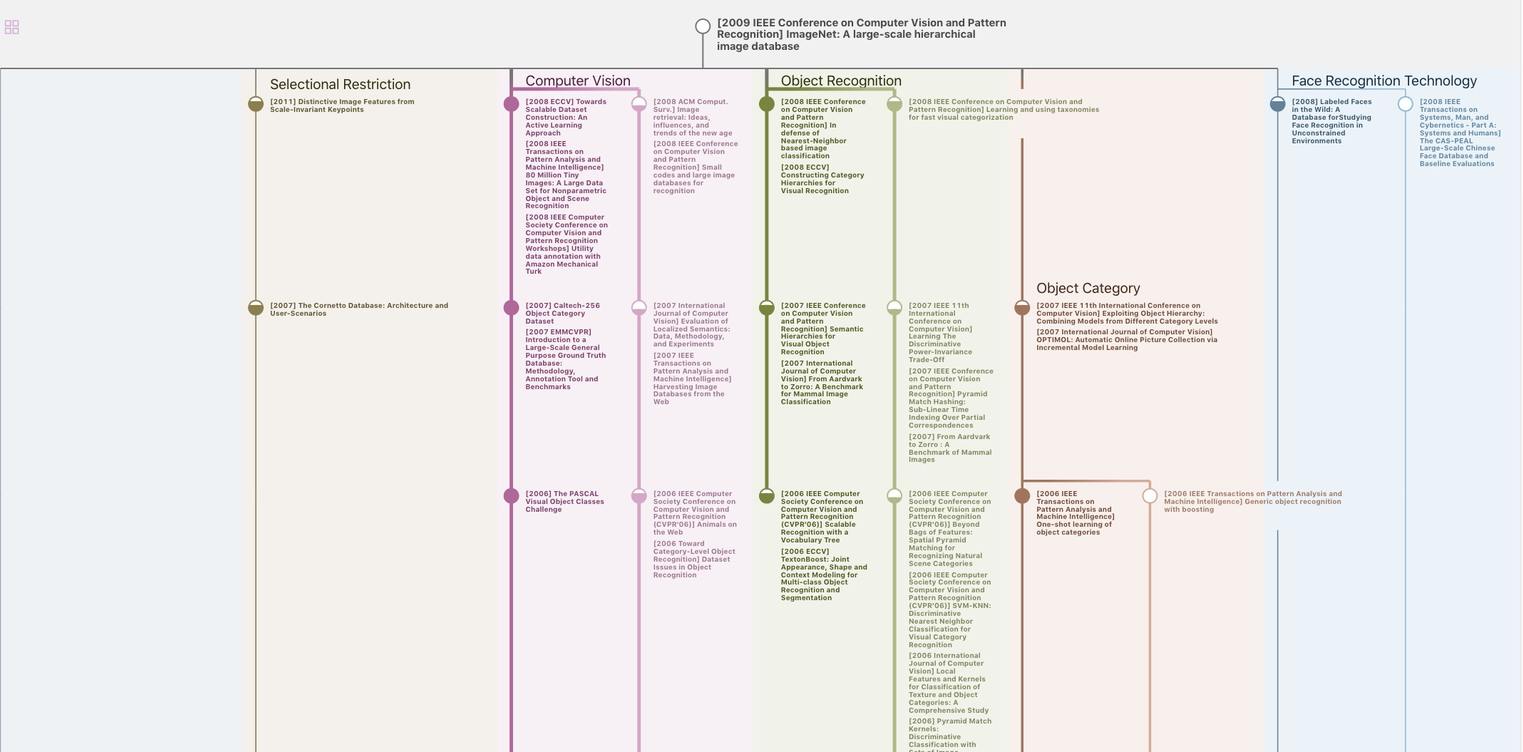
生成溯源树,研究论文发展脉络
Chat Paper
正在生成论文摘要