Automatically Discarding Straplines to Improve Data Quality for Abstractive News Summarization
PROCEEDINGS OF THE FIRST WORKSHOP ON EFFICIENT BENCHMARKING IN NLP (NLP POWER 2022)(2022)
摘要
Recent improvements in automatic news summarization fundamentally rely on large corpora of news articles and their summaries. These corpora are often constructed by scraping news websites, which results in including not only summaries but also other kinds of texts. Apart from more generic noise, we identify straplines as a form of text scraped from news websites that commonly turn out not to be summaries. The presence of these non-summaries threatens the validity of scraped corpora as benchmarks for news summarization. We have annotated extracts from two news sources that form part of the Newsroom corpus (Grusky et al., 2018), labeling those which were straplines, those which were summaries, and those which were both. We present a rule-based strapline detection method that achieves good performance on a manually annotated test set(1). Automatic evaluation indicates that removing straplines and noise from the training data of a news summarizer results in higher quality summaries, with improvements as high as 7 points ROUGE score.
更多查看译文
AI 理解论文
溯源树
样例
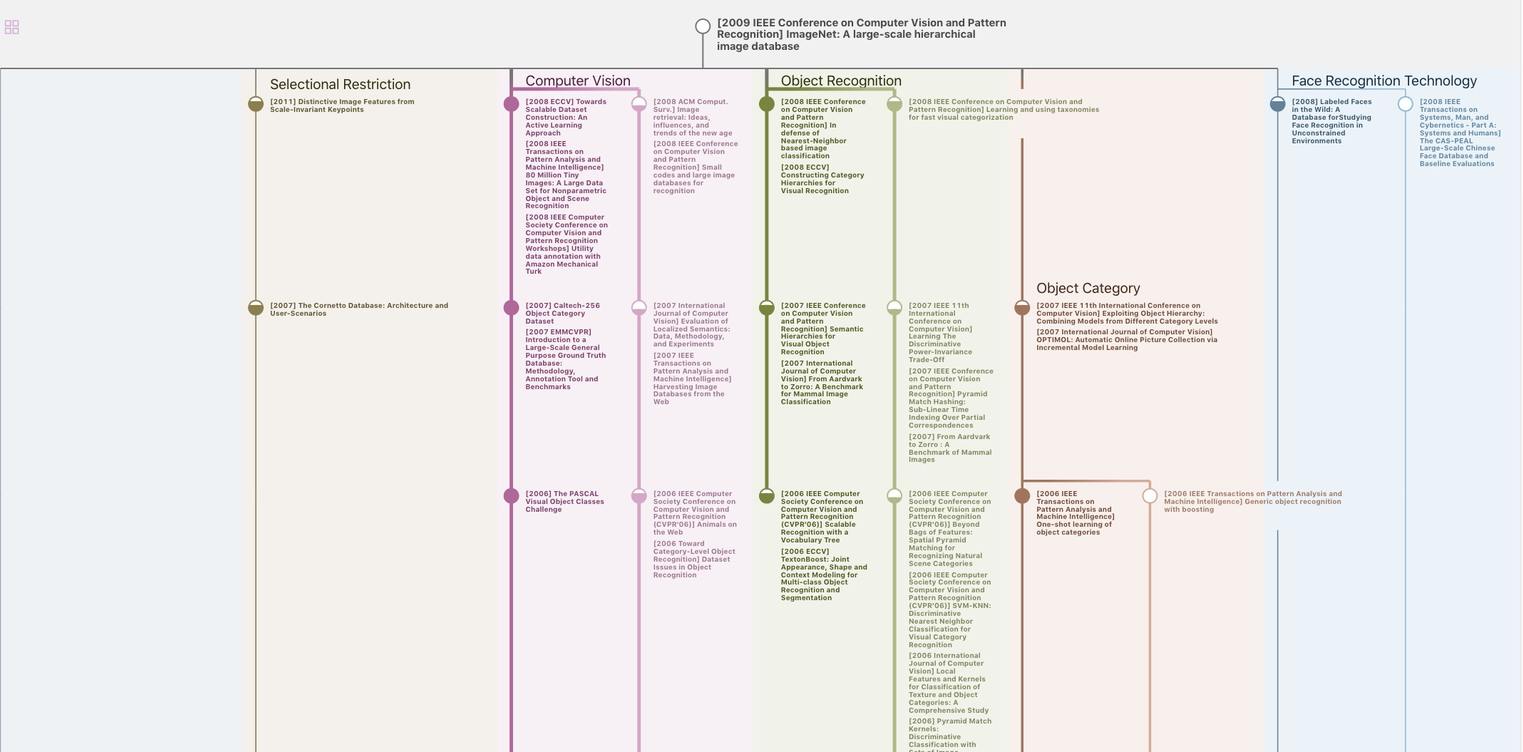
生成溯源树,研究论文发展脉络
Chat Paper
正在生成论文摘要