Zero-shot Cross-lingual Transfer is Under-specified Optimization
PROCEEDINGS OF THE 7TH WORKSHOP ON REPRESENTATION LEARNING FOR NLP(2022)
摘要
Pretrained multilingual encoders enable zero-shot cross-lingual transfer, but often produce unreliable models that exhibit high performance variance on the target language. We postulate that this high variance results from zero-shot cross-lingual transfer solving an under-specified optimization problem. We show that any linear-interpolated model between the source language monolingual model and source + target bilingual model has equally low source language generalization error, yet the target language generalization error reduces smoothly and linearly as we move from the monolingual to bilingual model, suggesting that the model struggles to identify good solutions for both source and target languages using the source language alone. Additionally, we show that zero-shot solution lies in non-flat region of target language error generalization surface, causing the high variance.
更多查看译文
关键词
transfer,zero-shot,cross-lingual,under-specified
AI 理解论文
溯源树
样例
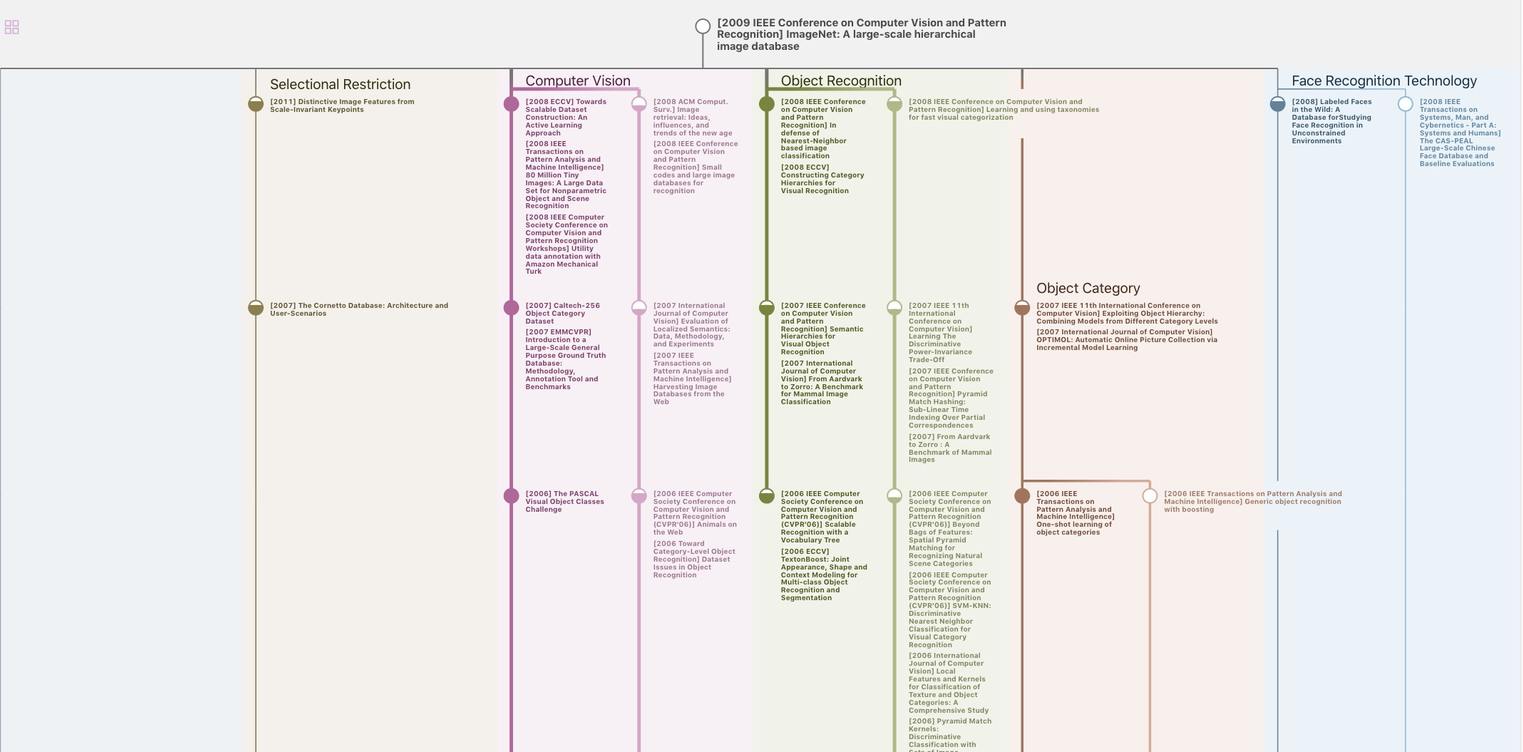
生成溯源树,研究论文发展脉络
Chat Paper
正在生成论文摘要