Scalable multi-view clustering with graph filtering
Neural Computing and Applications(2022)
摘要
With the explosive growth of multi-source data, multi-view clustering has attracted great attention in recent years. Most existing multi-view methods operate in raw feature space and heavily depend on the quality of original feature representation. Moreover, they are often designed for feature data and ignore the rich topology structure information. Accordingly, in this paper, we propose a generic framework to cluster both attribute and graph data with heterogeneous features. It is capable of exploring the interplay between feature and structure. Specifically, we first adopt graph filtering technique to eliminate high-frequency noise to achieve a clustering-friendly smooth representation. To handle the scalability challenge, we develop a novel sampling strategy to improve the quality of anchors. Extensive experiments on attribute and graph benchmarks demonstrate the superiority of our approach with respect to state-of-the-art approaches.
更多查看译文
关键词
Multi-view learning, attributed graph, subspace clustering, multiplex network
AI 理解论文
溯源树
样例
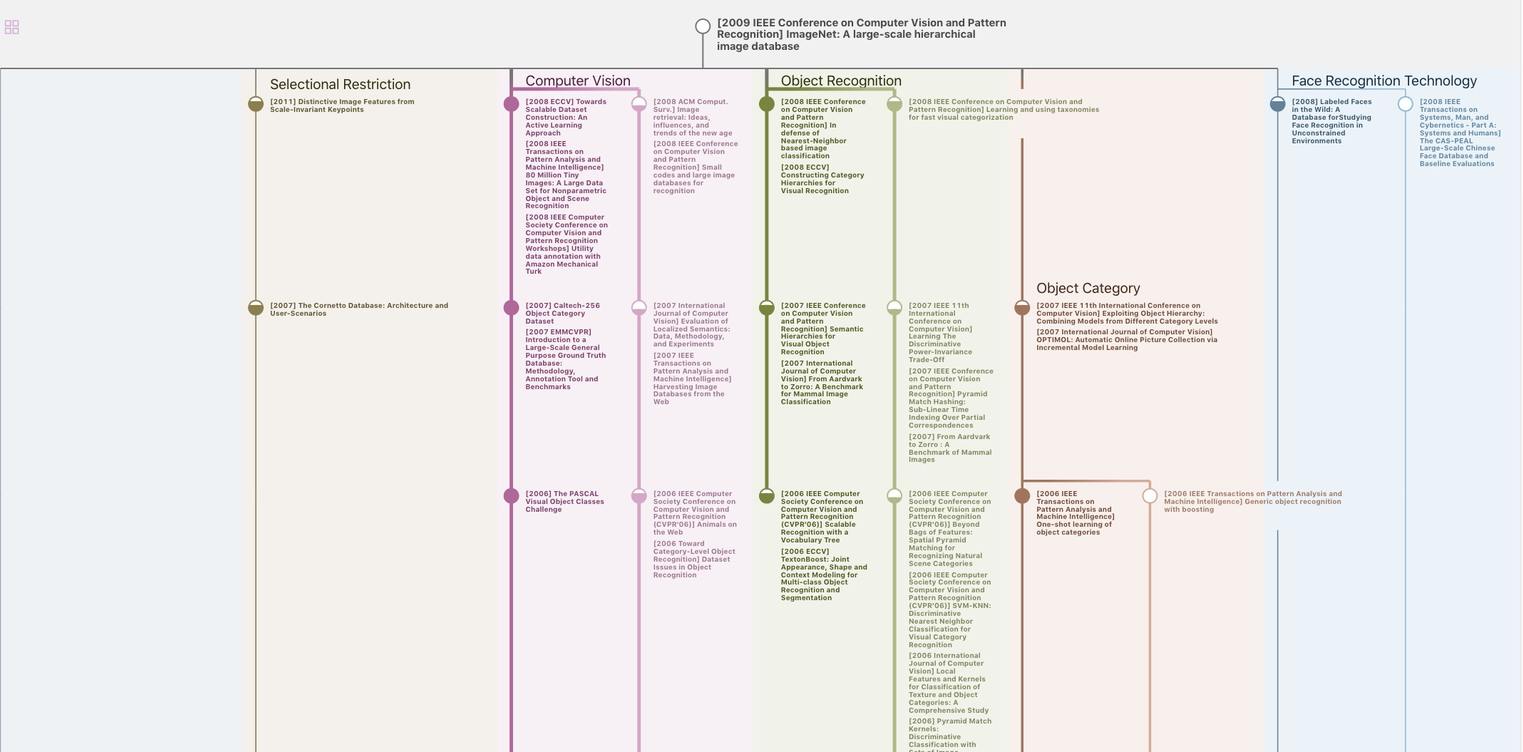
生成溯源树,研究论文发展脉络
Chat Paper
正在生成论文摘要