Building Trust in Interactive Machine Learning via User Contributed Interpretable Rules
Intelligent User Interfaces(2022)
摘要
ABSTRACT Machine learning technologies are increasingly being applied in many different domains in the real world. As autonomous machines and black-box algorithms begin making decisions previously entrusted to humans, great academic and public interest has been spurred to provide explanations that allow users to understand the decision-making process of the machine learning model. Besides explanations, Interactive Machine Learning (IML) seeks to leverage user feedback to iterate on an ML solution to correct errors and align decisions with those of the users. Despite the rise in explainable AI (XAI) and Interactive Machine Learning (IML) research, the links between interactivity, explanations, and trust have not been comprehensively studied in the machine learning literature. Thus, in this study, we develop and evaluate an explanation-driven interactive machine learning (XIML) system with the Tic-Tac-Toe game as a use case to understand how a XIML mechanism improves users’ satisfaction with the machine learning system. We explore different modalities to support user feedback through visual or rules-based corrections. Our online user study (n = 199) supports the hypothesis that allowing interactivity within this XIML system causes participants to be more satisfied with the system, while visual explanations play a less prominent (and somewhat unexpected) role. Finally, we leverage a user-centric evaluation framework to create a comprehensive structural model to clarify how subjective system aspects, which represent participants’ perceptions of the implemented interaction and visualization mechanisms, mediate the influence of these mechanisms on the system’s user experience.
更多查看译文
关键词
machine learning,XML,XIML,Tic-Tac-Toe game,user study,user-centric evaluation framework,user experience
AI 理解论文
溯源树
样例
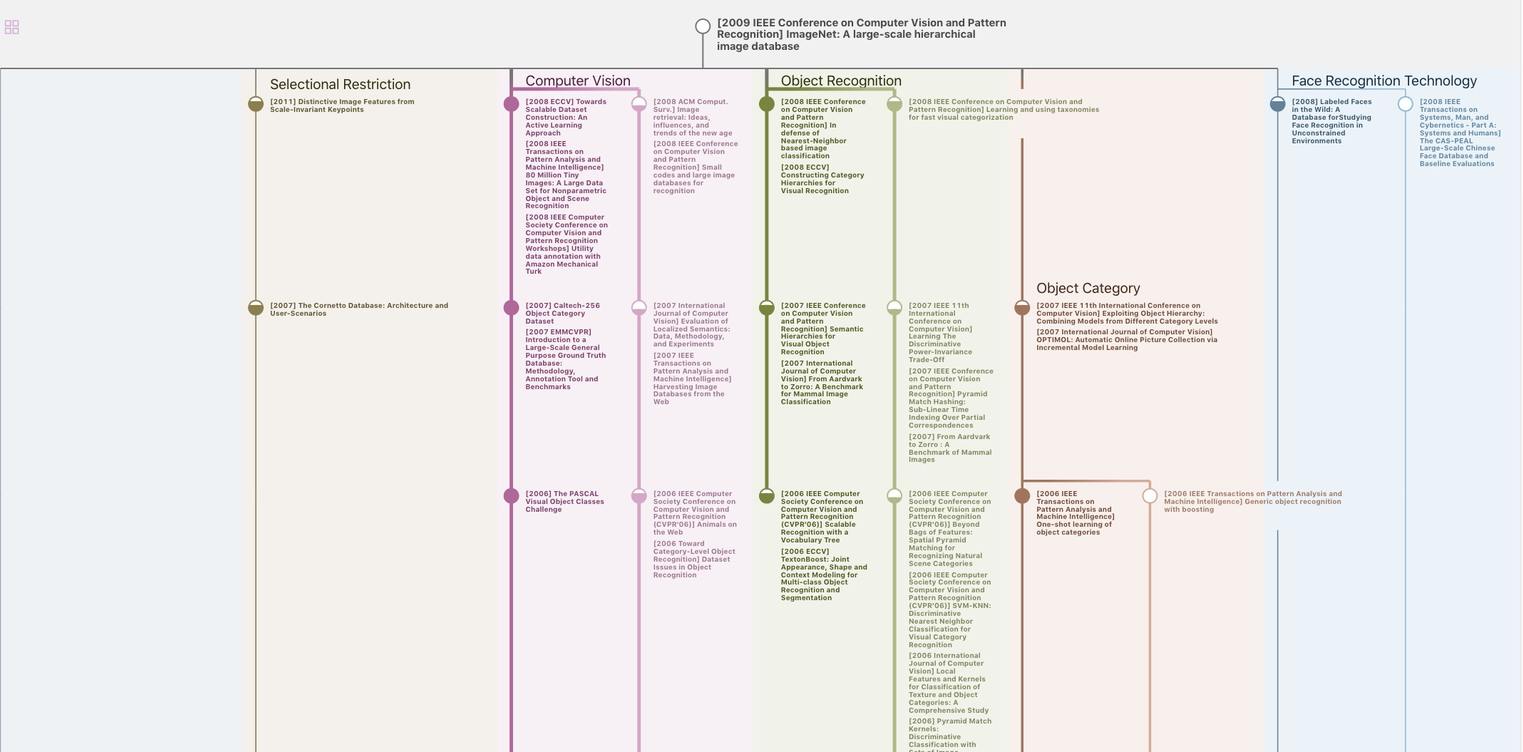
生成溯源树,研究论文发展脉络
Chat Paper
正在生成论文摘要