Trade-offs in Sampling and Search for Early-stage Interactive Text Classification
Intelligent User Interfaces(2022)
摘要
ABSTRACT For many automated classification tasks, collecting labeled data is the key barrier to training a useful supervised model. Interfaces for interactive labeling tighten the loop of labeled data collection and model development, enabling a subject-matter expert to quickly establish the feasibility of a classifier to address a problem of interest. These interactive machine learning (IML) interfaces iteratively sample unlabeled data for annotation, train a new model, and display feedback on the model’s estimated performance. Different sampling strategies affect both the rate at which the model improves and the bias of performance estimates. We compare the performance of three sampling strategies in the “early-stage” of label collection, starting from zero labeled data. By simulating a user’s interactions with an IML labeling interface, we demonstrate a trade-off between improving a text classifier’s performance and computing unbiased estimates of that performance. We show that supplementing early-stage sampling with user-guided text search can effectively “seed” a classifier with positive documents without compromising generalization performance—particularly for imbalanced tasks where positive documents are rare. We argue for the benefits of incorporating search alongside active learning in IML interfaces and identify design trade-offs around the use of non-random sampling strategies.
更多查看译文
关键词
sampling,classification,interactive machine learning
AI 理解论文
溯源树
样例
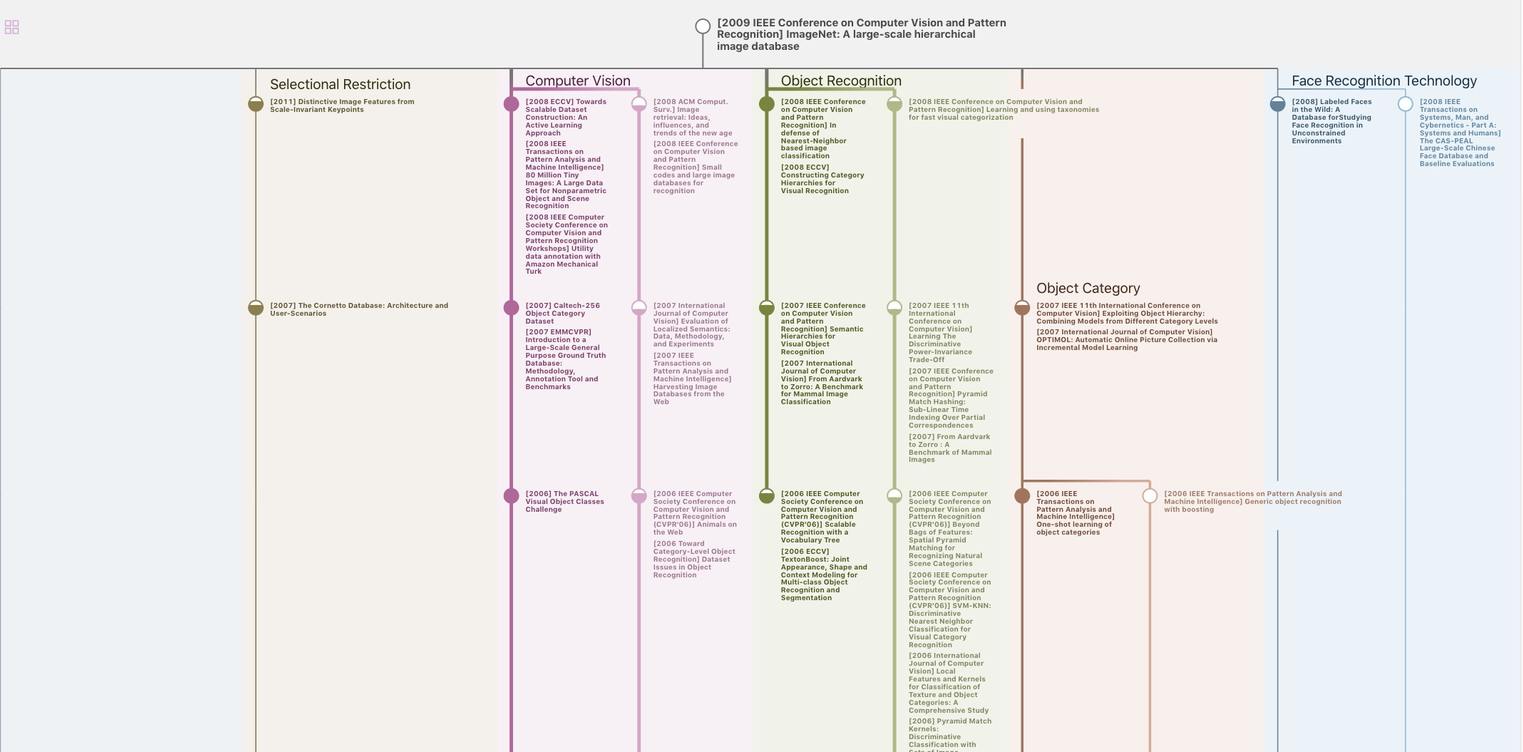
生成溯源树,研究论文发展脉络
Chat Paper
正在生成论文摘要