Domain Adaptation with Representation Learning and Nonlinear Relation for Time Series
ACM Transactions on Internet of Things(2022)
摘要
AbstractIn many real-world scenarios, machine learning models fall short in prediction performance due to data characteristics changing from training on one source domain to testing on a target domain. There has been extensive research to address this problem with Domain Adaptation (DA) for learning domain invariant features. However, when considering advances for time series, those methods remain limited to the use of hard parameter sharing (HPS) between source and target models, and the use of domain adaptation objective function. To address these challenges, we propose a soft parameter sharing (SPS) DA architecture with representation learning while modeling the relation as non-linear between parameters of source and target models and modeling the adaptation loss function as the squared Maximum Mean Discrepancy (MMD). The proposed architecture advances the state-of-the-art for time series in the context of activity recognition and in fields with other modalities, where SPS has been limited to a linear relation. An additional contribution of our work is to provide a study that demonstrates the strengths and limitations of HPS versus SPS. Experiment results showed the success of the method in three domain adaptation cases of multivariate time series activity recognition with different users and sensors.
更多查看译文
关键词
Domain adaptation,deep learning,representation learning
AI 理解论文
溯源树
样例
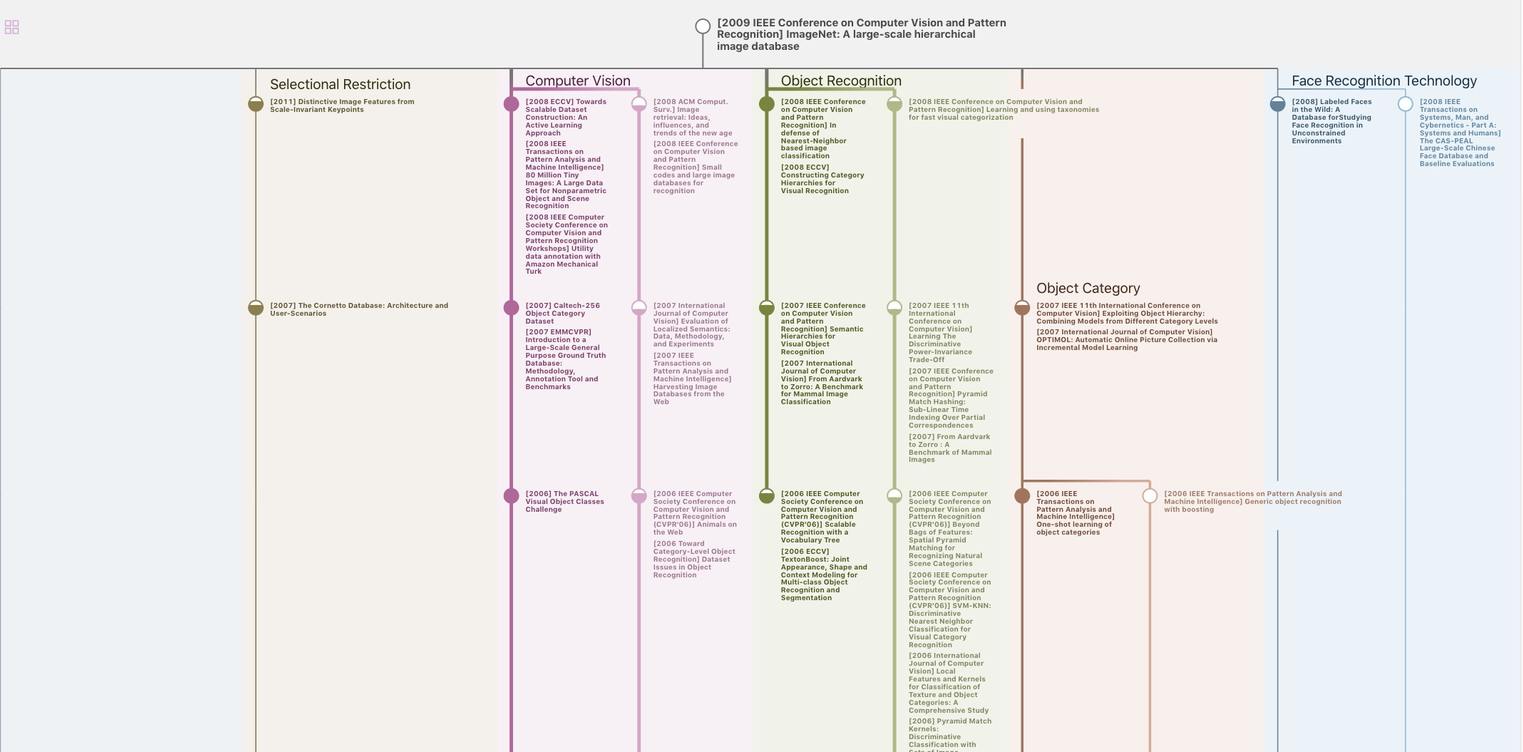
生成溯源树,研究论文发展脉络
Chat Paper
正在生成论文摘要