VFLH: A Following-the-Leader-History Based Algorithm for Adaptive Online Convex Optimization with Stochastic Constraints
2023 IEEE 35TH INTERNATIONAL CONFERENCE ON TOOLS WITH ARTIFICIAL INTELLIGENCE, ICTAI(2023)
摘要
This paper considers online convex optimization (OCO) with generated i.i.d. stochastic constraints, where the performance is measured by adaptive regret. The stochastic constraints are disclosed at each round to the learner after the decision is made. Different from the previous non-adaptive constrained OCO algorithm which is directly generalized from the static online gradient descent algorithm, we propose the novel Virtual Queue-based Following-the-Leader-History (VFLH) strategy to make the constrained OCO algorithm adaptive. In this framework, the algorithm generalizes experts that deal with the static constrained optimization problem within specified time intervals. Subsequently, it combines the predictions of active experts to produce a final choice and unify the average regret and constraints virtual queue. The algorithm's performance is evaluated based on two metrics: the bounds of constraint violation and regret. To address the difficulty of proving the constraint violation bound under the adaptive setting, we first employ the multi-objective drift analysis approach to handle the constraints virtual queue. Further analysis of the regret bound and the numerical results also supports the performance of the newly proposed algorithm.
更多查看译文
关键词
Adaptive regret,online convex optimization,constrained optimization
AI 理解论文
溯源树
样例
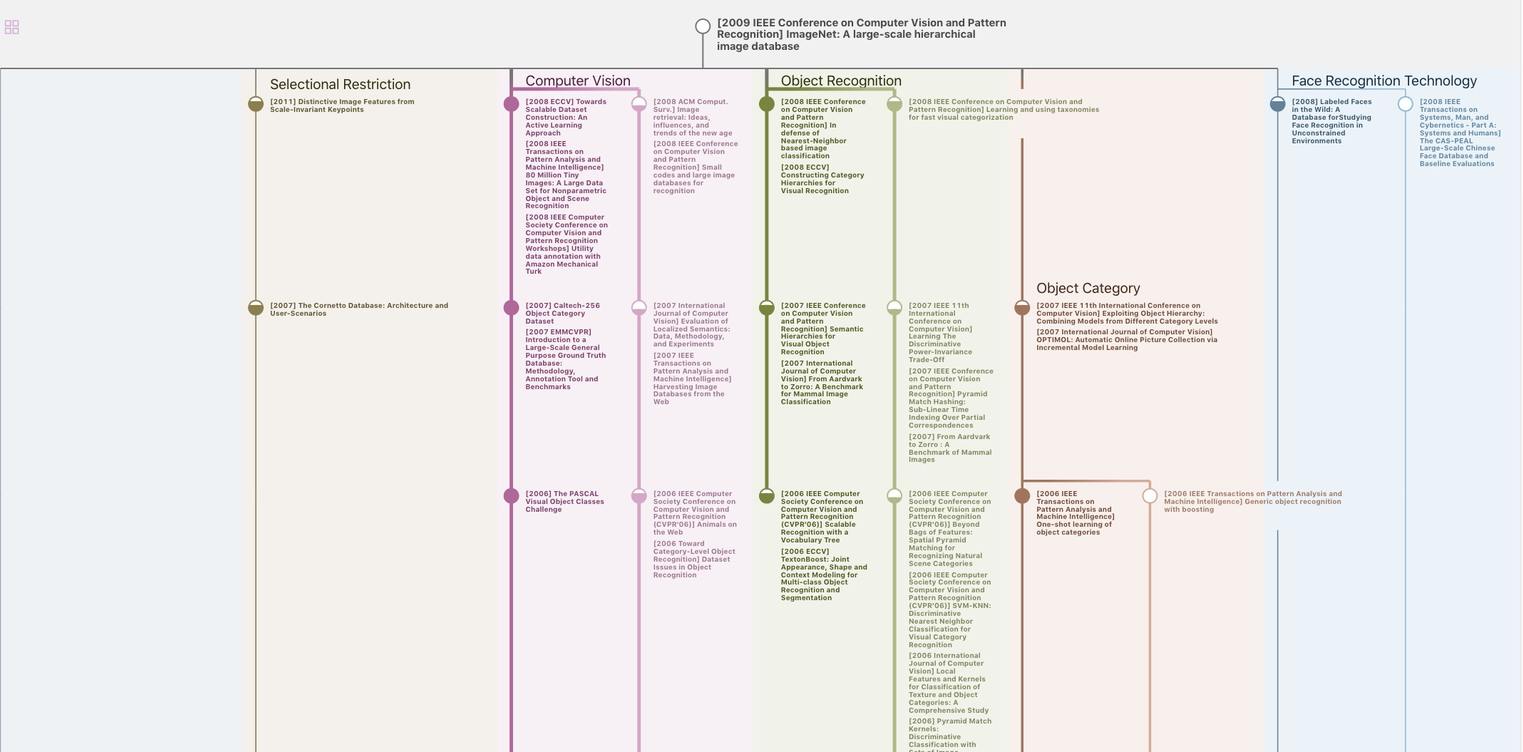
生成溯源树,研究论文发展脉络
Chat Paper
正在生成论文摘要