Multiple Imputation Using the Average Code from Autoencoders
Computer methods and programs in biomedicine update(2022)
摘要
• It is possible to compute a more robust representation of latent spaces through the integration of MI into latent spaces of deep neural networks. • The computation of the average code from an autoencoder Improves the imputation of missing values. Background: Missing information is a constant issue in the clinical setting. The presence of missing values (MV) is triggered by the wrong acquisition of data or sudden events in the patient’s health condition. Imputation arises to replace the non-existent information with the twofold purpose of benefiting from existing information and reducing bias in clinical settings. Mechanisms based on deep learning and multiple imputation (MI) are leading alternatives to impute MVs because of their capacity to extract complex relationships and the consideration of uncertainty that MI adds. Objective: This study aims to improve the reconstruction of missing information through a novel imputation alternative that integrates a MI paradigm into deep learning models. Methods: The proposed method integrates the MI paradigm into the latent representations of an autoencoder, the so-called codes. The average code is then computed, boosting a better latent representation of data. Finally, the average code is decoded to reconstruct MVs. Results: The proposed method is tested in 6 datasets with different patters of MVs. It is compared with solutions based on autoencoders and generative adversarial networks. For the random appearance of MVs, the proposed method outperforms 97% of the scenarios with a reconstruction gain that ranges 1.04-1.45. For the other MVs mechanisms, the proposed method improves the reconstruction in at least 69% of the experiments, with a gain of 1.13-1.91. Conclusion: The findings of the proposed approach showed that the reconstructive capacity of the average code outperforms in most of the scenarios its competitors and close to the best solution in the rest of the scenarios. The integration of the MI paradigm into latent representations of data and the computation of average codes allow a more robust representation of the data and enables the enhancement of current state-of-the-art methods for high MVs rates.
更多查看译文
关键词
Average code,Deep learning,Autoencoder,Multiple imputation
AI 理解论文
溯源树
样例
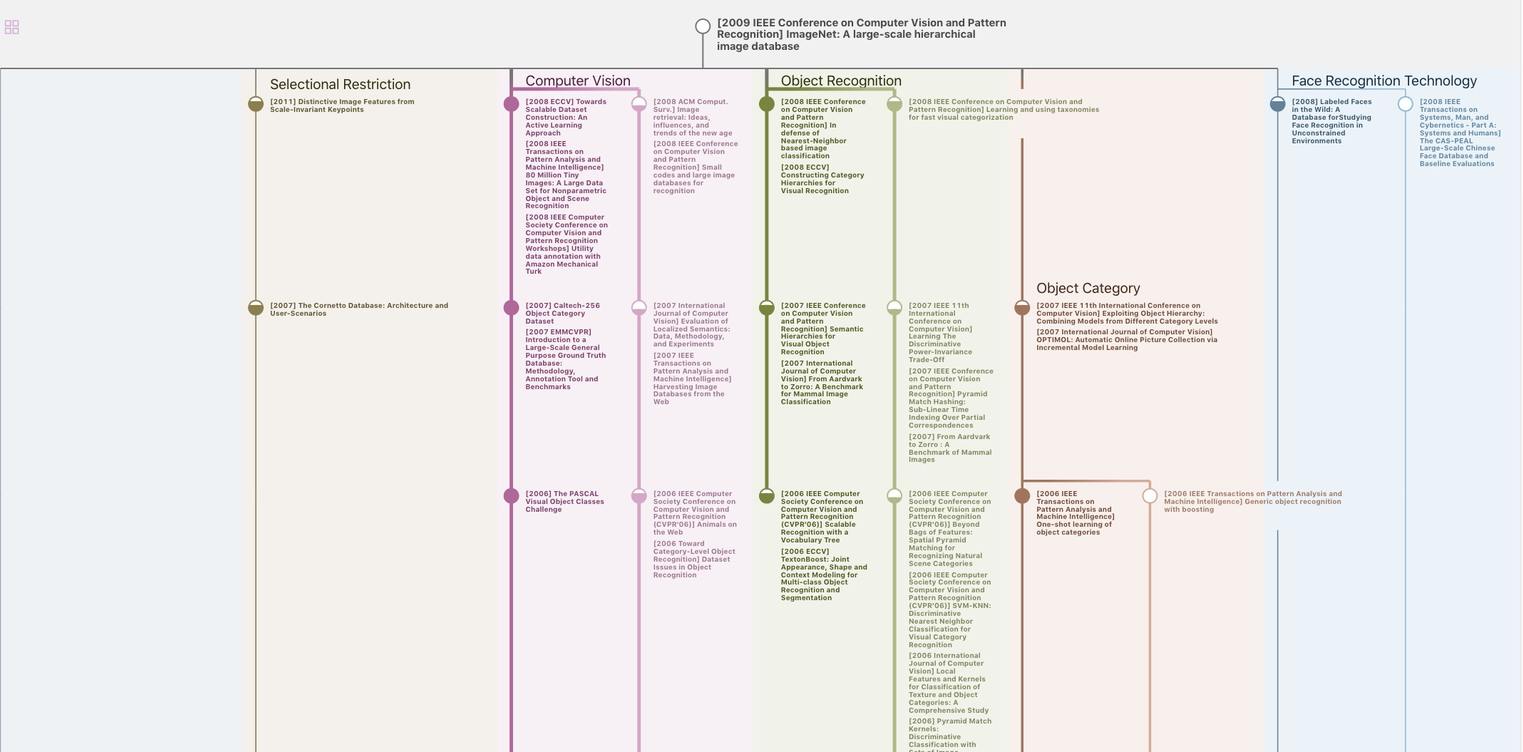
生成溯源树,研究论文发展脉络
Chat Paper
正在生成论文摘要