Toward Image-Guided Automated Suture Grasping Under Complex Environments: A Learning-Enabled and Optimization-Based Holistic Framework
IEEE Transactions on Automation Science and Engineering(2022)
摘要
To realize a higher-level autonomy of surgical knot tying in minimally invasive surgery (MIS), automated suture grasping, which bridges the suture stitching and looping procedures, is an important yet challenging task needs to be achieved. This paper presents a holistic framework with image-guided and automation techniques to robotize this operation even under complex environments. The whole task is initialized by suture segmentation, in which we propose a novel semi-supervised learning architecture featured with a suture-aware loss to pertinently learn its slender information using both annotated and unannotated data. With successful segmentation in stereo-camera, we develop a Sampling-based Sliding Pairing (SSP) algorithm to online optimize the suture’s 3D shape. By jointly studying the robotic configuration and the suture’s spatial characteristics, a target function is introduced to find the optimal grasping pose of the surgical tool with Remote Center of Motion (RCM) constraints. To compensate for inherent errors and practical uncertainties, a unified grasping strategy with a novel vision-based mechanism is introduced to autonomously accomplish this grasping task. Our framework is extensively evaluated from learning-based segmentation, 3D reconstruction, and image-guided grasping on the da Vinci Research Kit (dVRK) platform, where we achieve high performances and successful rates in perceptions and robotic manipulations. These results prove the feasibility of our approach in automating the suture grasping task, and this work fills the gap between automated surgical stitching and looping, stepping towards a higher-level of task autonomy in surgical knot tying. Note to Practitioners—This paper aims to automate the suture grasping task in surgical knot tying by leveraging stereo visual guidance. To effectively robotize this procedure, it requires multidisciplinary knowledge to achieve suture segmentation, 3D shape reconstruction, and reliable automated grasping, while there are no existing works tackling this procedure especially using robots with RCM kinematics constraints and under complex environments. In this article, we propose a learning-driven method along with a 3D shape optimizer, which can conduct the suture segmentation and output its accurate spatial coordinates, serving as guidance for automated grasping operation. Apart from this, we introduce a unified function to optimize the grasping pose, and a vision-based grasping strategy is also proposed to intelligently complete this task. The experiments extensively validate the feasibility of our framework for automated suture grasp, and its successful completion can serve as a basis for the following looping manipulation, hence filling a step gap in robot-assisted knot tying. This framework can be also encapsulated into the medical robotic system, and by simply indicating (e.g. mouse click) the rough position of the suture’s tip in one camera frame, the overall framework can be initialized and further accomplish the suture grasping task, which further prompts a full autonomy of surgical knot tying in the near future.
更多查看译文
关键词
Medical robotics,vision-based manipulation,automated suture grasping,surgical knot tying
AI 理解论文
溯源树
样例
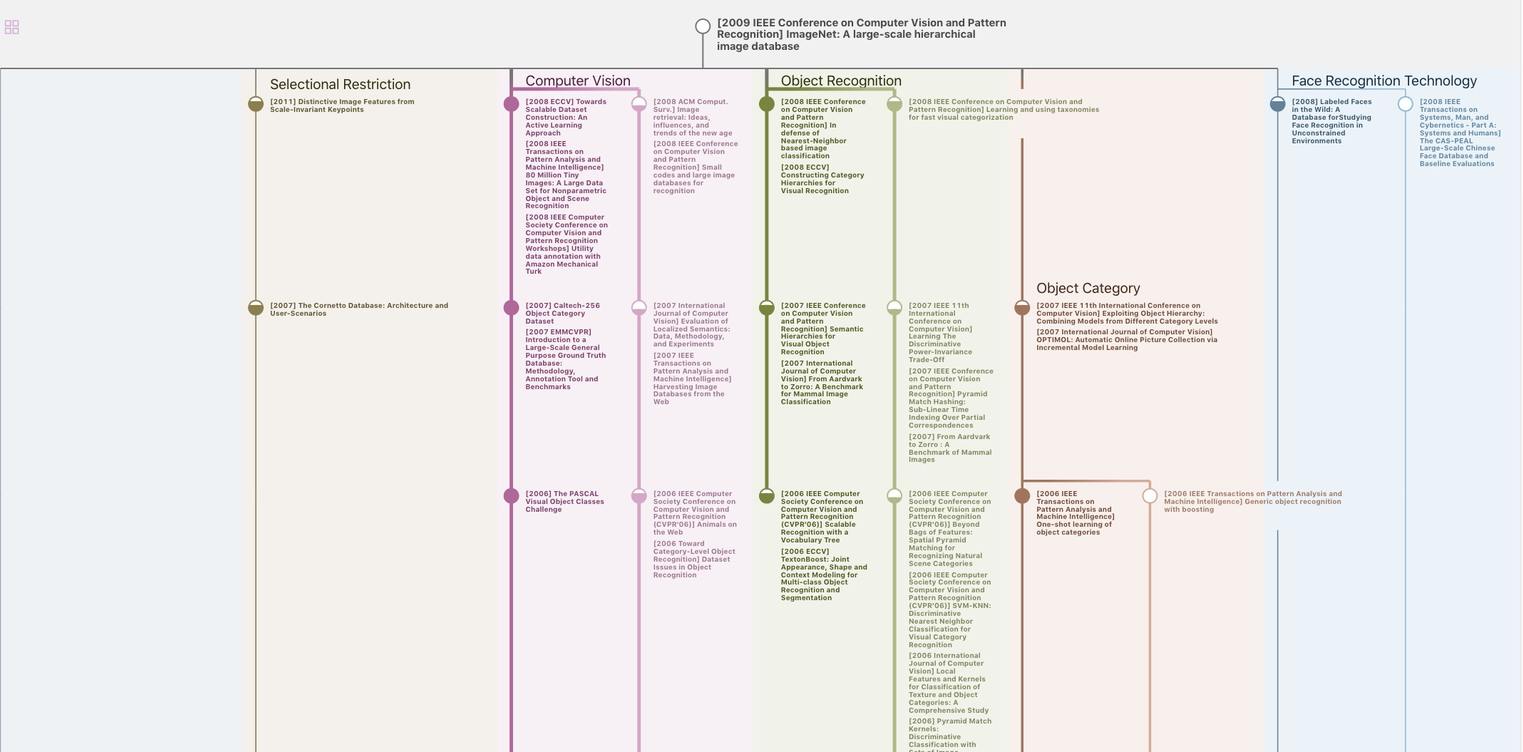
生成溯源树,研究论文发展脉络
Chat Paper
正在生成论文摘要