Forecasting network events to estimate attack risk: Integration of wavelet transform and vector auto regression with exogenous variables
Journal of Network and Computer Applications(2022)
摘要
Analyzing network traffic data to detect suspicious network activities (i.e., intrusions) requires tremendous effort due to the variability of the data and constant changes in network traffic patterns. This study introduces an approach to predict future network event (normal and attack) frequencies by generating forecast models and estimating their attack risks. Wavelet transform is used to extract features, and the multivariate time series method, Vector Auto Regression with eXogenous variables (VARX), is utilized to predict future network traffic events. Attack risks for network events are estimated with an adaptive threshold method and assessed by performing classification with two machine learning techniques. We also performed a comparative evaluation to examine the relationship among different time scales (one second, five seconds, and fifteen seconds) and three wavelets in determining attack risks. Our analysis results indicate that wavelet features with VARX show the capability to analyze multivariate network traffic time series data to forecast future network events and estimate their attack risks.
更多查看译文
关键词
Deception,Multivariate time series analysis,Attack risk estimation,Honeypot,Wavelet transform,Network intrusion
AI 理解论文
溯源树
样例
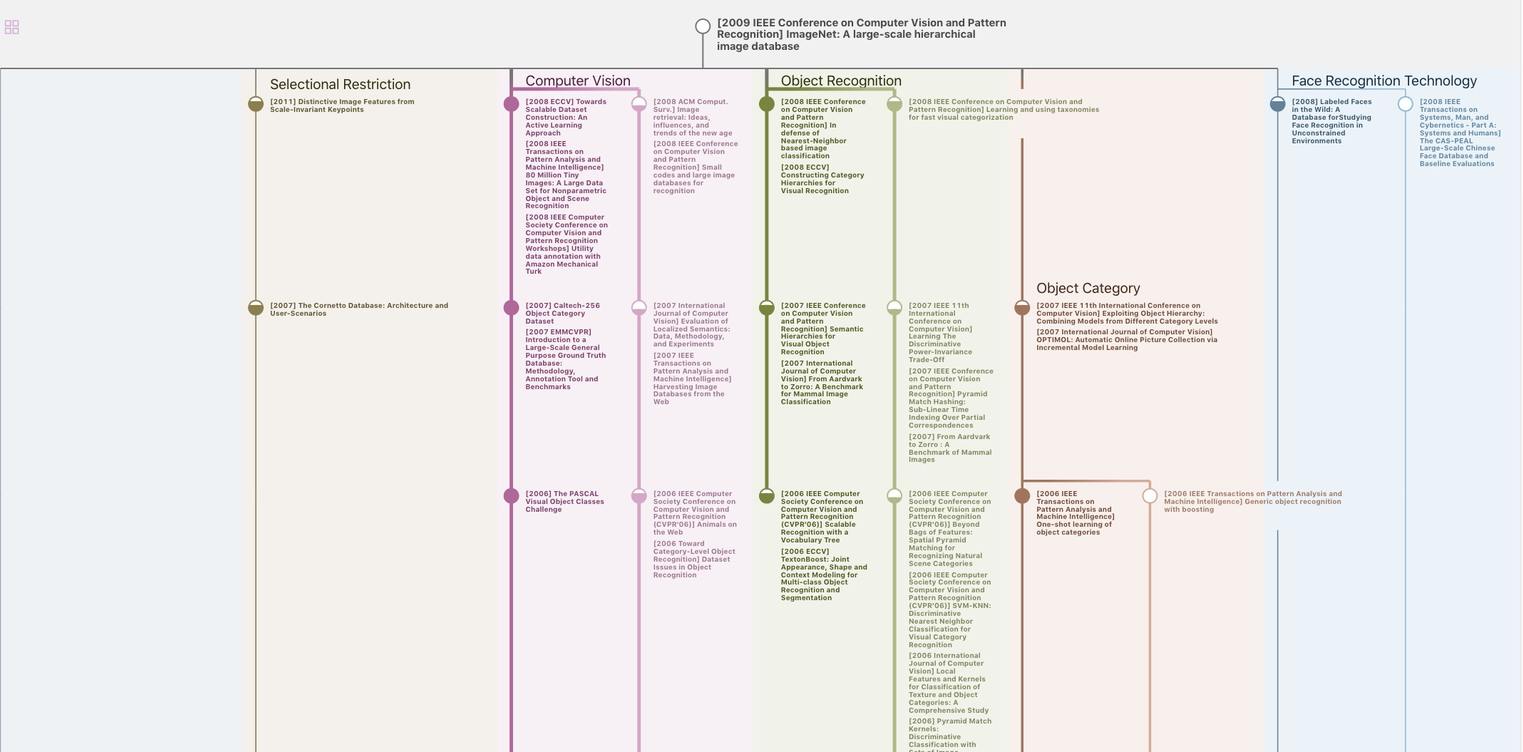
生成溯源树,研究论文发展脉络
Chat Paper
正在生成论文摘要