Entity Alignment with Reliable Path Reasoning and Relation-aware Heterogeneous Graph Transformer
International Joint Conference on Artificial Intelligence(2022)
摘要
Entity Alignment (EA) has attracted widespread attention in both academia and industry, which aims to seek entities with same meanings from different Knowledge Graphs (KGs). There are substantial multi-step relation paths between entities in KGs, indicating the semantic relations of entities. However, existing methods rarely consider path information because not all natural paths facilitate for EA judgment. In this paper, we propose a more effective entity alignment framework, RPR-RHGT, which integrates relation and path structure information, as well as the heterogeneous information in KGs. Impressively, an initial reliable path reasoning algorithm is developed to generate the paths favorable for EA task from the relation structures of KGs, which is the first algorithm in the literature to successfully use unrestricted path information. In addition, to efficiently capture heterogeneous features in entity neighborhoods, a relation-aware heterogeneous graph transformer is designed to model the relation and path structures of KGs. Extensive experiments on three well-known datasets show RPR-RHGT significantly outperforms 11 state-of-the-art methods, exceeding the best performing baseline up to 8.62% on Hits@1. We also show its better performance than the baselines on different ratios of training set, and harder datasets.
更多查看译文
关键词
Data Mining: Knowledge Graphs and Knowledge Base Completion,Knowledge Representation and Reasoning: Semantic Web
AI 理解论文
溯源树
样例
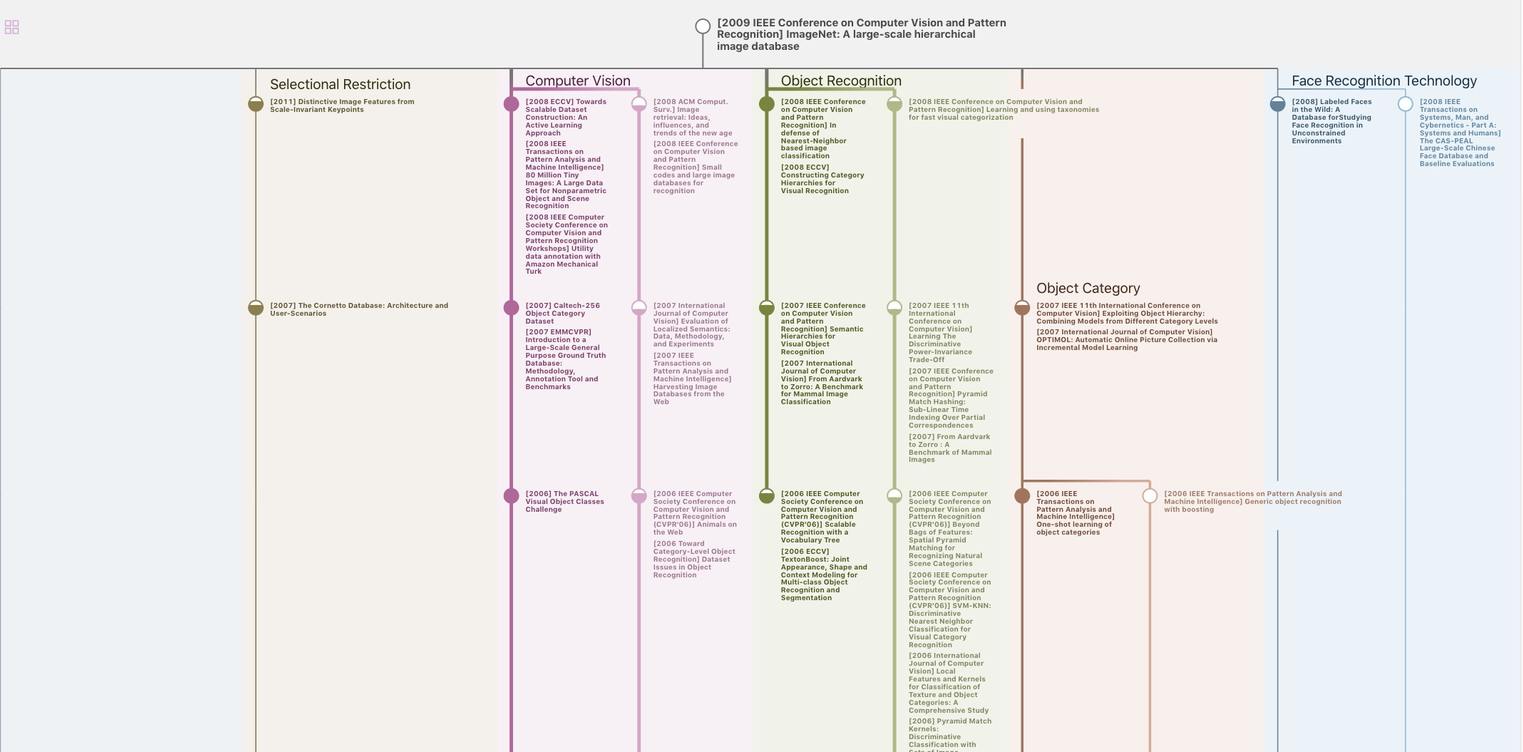
生成溯源树,研究论文发展脉络
Chat Paper
正在生成论文摘要