Increasing Context for Estimating Confidence Scores in Automatic Speech Recognition
IEEE-ACM TRANSACTIONS ON AUDIO SPEECH AND LANGUAGE PROCESSING(2022)
摘要
Accurate confidence measures for predictions from machine learning techniques play a critical role in the deployment and training of many speech and language processing applications. For example, confidence scores are important when making use of automatically generated transcriptions in training automatic speech recognition (ASR) systems, as well as down-stream applications, such as information retrieval and conversational assistants. Previous work on improving confidence scores for these systems has focused on two main directions: designing features correlated with improved confidence prediction; and employing sequence models to account for the importance of contextual information. Few studies, however, have explored incorporating contextual information more broadly, such as from the future, in addition to the past, or making use of alternative multiple hypotheses in addition to the most likely one. This article introduces two general approaches for encapsulating contextual information from lattices. Experimental results illustrating the importance of increasing contextual information for estimating confidence scores are presented on a range of limited resource languages where word error rates range between 30% and 60%. The results show that the novel approaches provide significant gains in the accuracy of confidence estimation.
更多查看译文
关键词
Lattices, Hidden Markov models, Speech processing, Estimation, History, Feature extraction, Stability criteria, Attention, confidence, graph structures, recurrent neural network, speech recognition
AI 理解论文
溯源树
样例
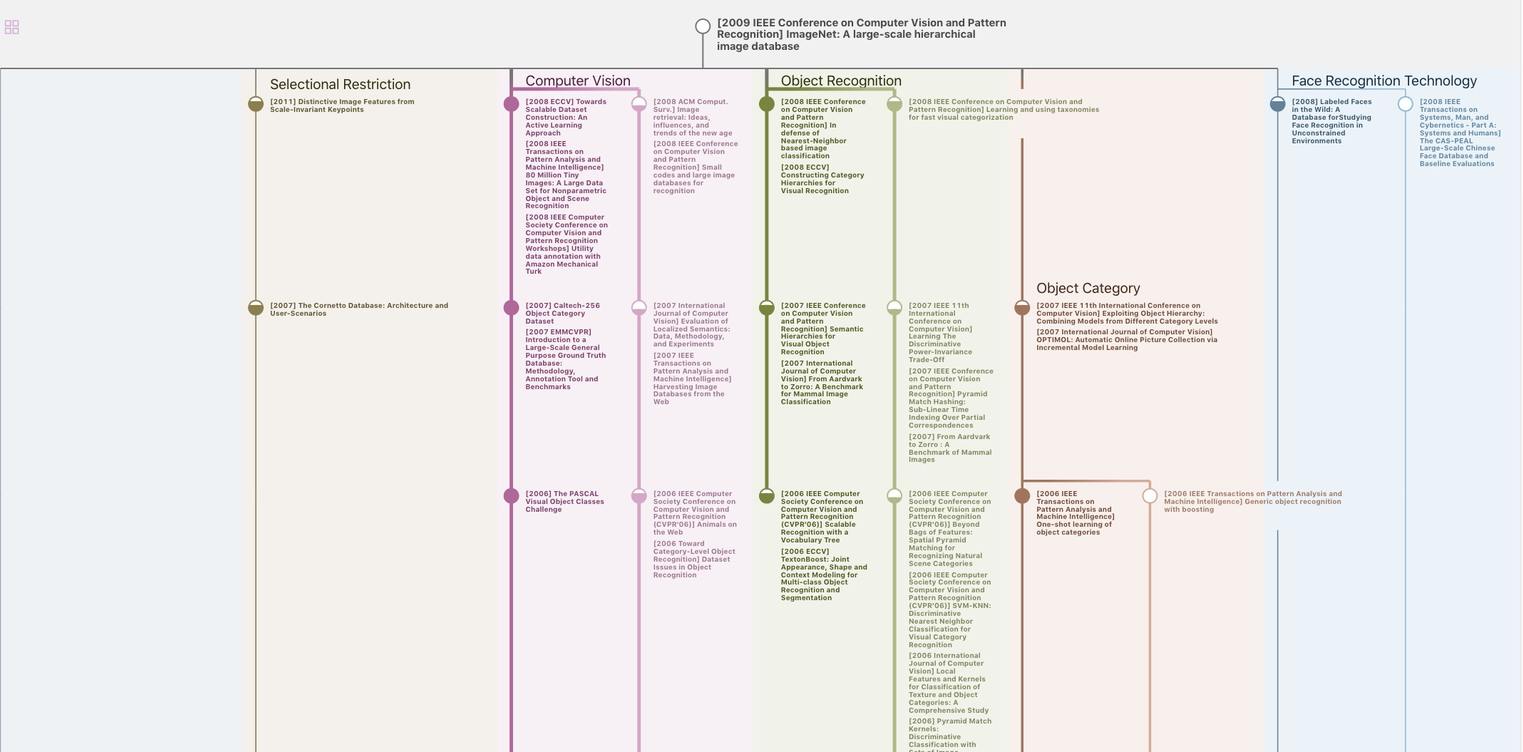
生成溯源树,研究论文发展脉络
Chat Paper
正在生成论文摘要